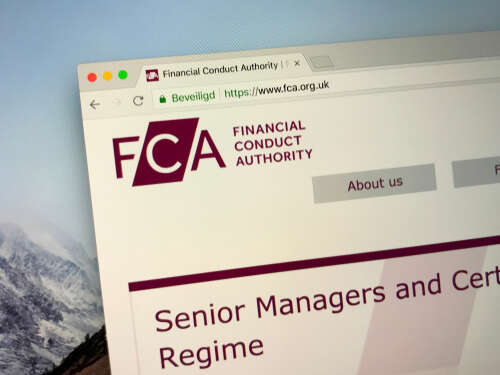
The UK’s Financial Conduct Authority (FCA) is recruiting synthetic data experts for a new panel that will explore its use in financial services. This will include training machine learning models for fraud detection, reducing lending risk and know your customer (KYC) compliance services without putting sensitive and valuable real data at risk. One academic told Tech Monitor the challenge is to ensure the synthetic data matches the patterns of real data and can be properly validated.
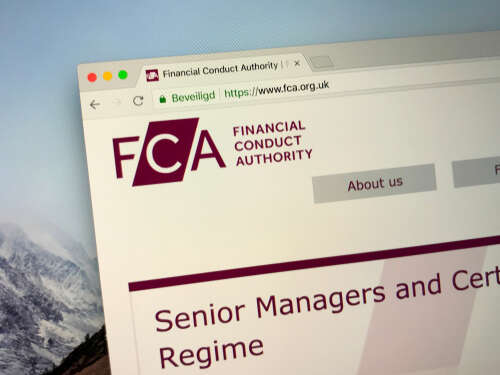
The new Synthetic Data Expert Group will sit within the financial regulator’s Innovation Advisory Group and help the FCA better understand what role the artificially generated information could play in “enabling enhanced capabilities to protect consumers and encourage beneficial innovation in financial services.”
Meeting every eight weeks or so, the group will be expected to identify relevant use cases for synthetic data in financial markets, clarify key issues in the theory and practice of synthetic data and develop best practice as relevant to UK financial services, including a framework for collaboration across industry and with regulators, academia and civil society.
According to AI research body the Alan Turing Institute there are a number of potential benefits to using synthetic data for financial services including using data in an unsafe environment, training new models without using real customer information, increasing the data size for a training model, validating models and fixing structural deficiencies in data.
“Synthetic data can be shared between companies, departments and research units for synergistic benefits,” a report by the institute explained. “By using synthetic data, organisations can store the relationships and statistical patterns of their data, without having to store individual level data” and it can allow for testing extreme scenarios not covered by real data.
Financial services data is both valuable and high risk in terms of regulatory oversight. It contains highly confidential and personal information that could leave a company caught mishandling it vulnerable to extensive fines. This has led to a scenario where companies are reluctant to put this data to work in training machine learning models which could help predict fraud attempts, streamline KYC and reduce lending risk.
Synthetic data solves some of the regulatory issues by allowing models to be trained on “real-looking data” without the risk of actual customer information being exposed. Models based on synthetic data, once trained, can then be set to work on the real data after validation and testing is complete.
The first meeting of the panel will be in April this year and will operate every eight weeks for 18 months, with the option of extending it by six months if further examination is required. It is expected the panel will be made up of experts from regulated companies, consultants, legal professionals, accelerators, consumer groups and academia.
Benefits and risks of synthetic data
Professor Lukasz Szpruch from the University of Edinburgh is the director of the Alan Turing Institute Finance and Economics Programme. He said synthetic data is already in widespread use in other areas of the economy, from self-driving vehicles to generative text tools like OpenAI’s large language model GPT-3. It is also in use for research into protein folding and drug discovery, trained on existing large datasets to uncover new information not previously available.
In finance, he said there are two key applications for the technology. The first is connected to privacy, allowing machine learning models to be trained, tested and shared without using risky and highly personal information subject to GDPR restrictions. “Banks can’t share private financial transactions of people even inside their organisation or with other banks to have a better picture of trends and risks,” says Szpruch. “The idea is that if we can simulate fake data that captures the statistical properties of real data without the privacy risk then we can safely train these models that can then be run on real data.
“Synthetic data isn’t a real replacement for real data, it is just a way to speed up the process. You don’t have to wait for months to get access to the real data. It also allows a company to check the value they’d gain from a new model without having to share their real data. It can be used to accelerate that. If you are able to generate it well and have privacy guarantees then in principle it is a great idea.”
The other key area is in augmenting data to make better predictions, or to carry out statistical and risk analysis on data that doesn’t exist in the real world. This could include simulating the impact of a pandemic or natural disaster, or simply testing whether an algorithm will operate under those stress conditions.
It isn’t risk-free, he explained. “We are running into a risk that we will be surrounded by huge amounts of data. Some real, some synthetic and it will be hard to tell which is which. It is also important we have a way to ensure the synthetic data is accurate and reflects the patterns of real data.”
The Alan Turing Institute is working with the Office of National Statistics on ways to reduce privacy risks that might make it easier to train synthetic models on real-world data. “Data holders have a number of questions when it comes to requests to use their data in this way,” said Szpruch. “In the same way we want to trust algorithms and have a way of monitoring them, we need a way to validate and monitor to ensure the synthetic data is accurate.”
This could include ensuring any synthetic data generator has validation statements that contain some statistical information an auditor can process to show a percentage chance that “what I’m being told is trustworthy” and accurate, he said.