Researchers at King’s College London and chipmaker NVIDIA have released 100,000 synthetic 3D brain scans that were created using the Cambridge-1 supercomputer, as well as a framework for deep learning in healthcare imaging. The AI-generated images, which are intended for use by scientists researching neurological conditions, highlight the emerging use of synthetic data to bolster healthcare research.
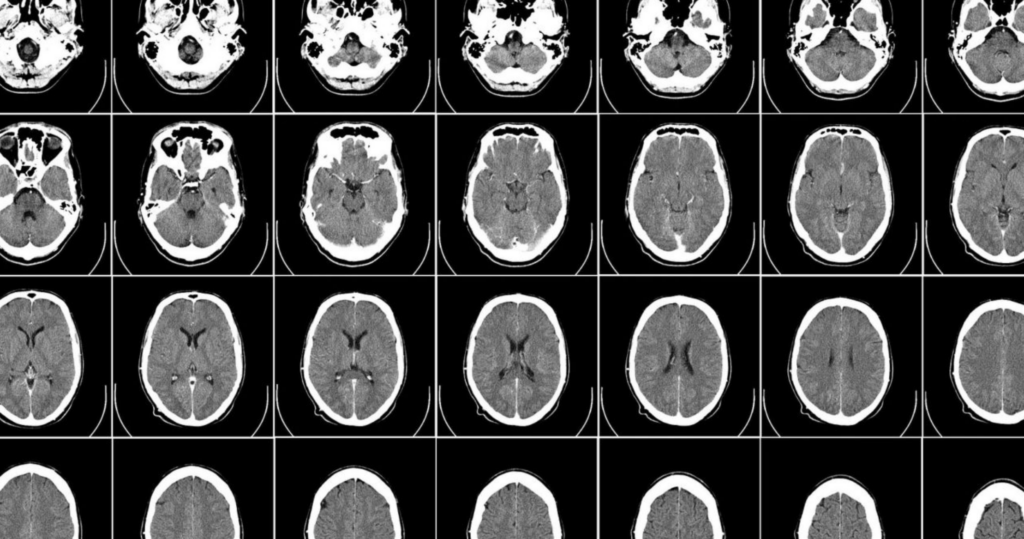
The synthetic MRI scans were developed using MONAI, a deep-learning framework for medical imaging that was launched by NVIDIA and King’s College London in 2020. The framework, based on Python-based deep learning library PyTorch, provides tools for researchers to apply deep learning to medical images, from data labelling and analysis to application development.
The team used MONAI and the Cambridge-1 supercomputer to create an “AI factory for synthetic data”, running hundreds of experiments to find the best AI models with the most accurate data possible. Using this system, KCL researchers were able to generate 100,000 clinically accurate synthetic MRI scans in just 48 hours.
In the past, the application of AI to biomedical research has been hampered by a lack of suitable training data, said lead researcher Jorge Cardoso, reader in artificial medical intelligence at King’s College London and founding member of the MONAI consortium, in a statement. The MONAI project has created a “treasure trove” of images for researchers to use, he said.
An NVIDIA spokesperson told Tech Monitor that using the MONAI framework, Cambridge-1 will be able to create synthetic medical images “to order”. “Female brains, male brains, old ones, young ones, brains with or without disease – plug in what you need, and it creates them,” the spokesperson said. “Though they’re simulated, the images are highly useful because they preserve key biological characteristics, so they look and act like real brains would.”
The same technique could be used to simulate any kind of medical imaging, including CAT and PET scans, Cardoso said in statement. “In fact, this technique can be applied to any volumetric image”.
“It can be a bit overwhelming,” he added. “There are so many different things we can start thinking about now.”
Synthetic data for healthcare research: pros and cons
As well as creating realistic training data for AI models, synthetic data can also help researchers skirt any privacy concerns about using patient records, explains Frederik Hvilshøj, machine learning engineer at computer vision data platform provider Encord.
“Due to data privacy and other restricting factors, the amount of available data within medicine has been scarce,” Hvilshøj told Tech Monitor. “As a consequence, model performance has also not been optimal.”
Synthetic data is also cheap and quick to produce, Hvilshøj added. “Imagine having to bring 100,000 people to an MRI to scan them one at a time. This would be super costly. With the synthetic generation of data, this can be done within a fraction of the time with a click of a button.”
There are downsides to using synthetic data, however, including the need to ensure it is realistic. This is a “hard problem to solve”, says Hvilshøj, and costly if you need to keep a human in the loop to verify training models.
“This is not a trivial task. We also need to make sure that the data generation process has not overfitted to the training data, which would cause us to end up with close to identical samples as those in the training set.”
Quality assurance is essential when using synthetic data to train AI systems, Hvilshøj explained, as it might otherwise lead to skewed results.
“The biggest risk is relying blindly on synthetic data. Without a proper quality assurance of the data, chances are that the downstream models trained on the data will be even more ‘rogue’.”
Synthetic data is being used to support AI development in a number of application areas. Last year, for example, the International Organisation for Migration (IOM) and Microsoft launched a synthetic dataset for human trafficking.