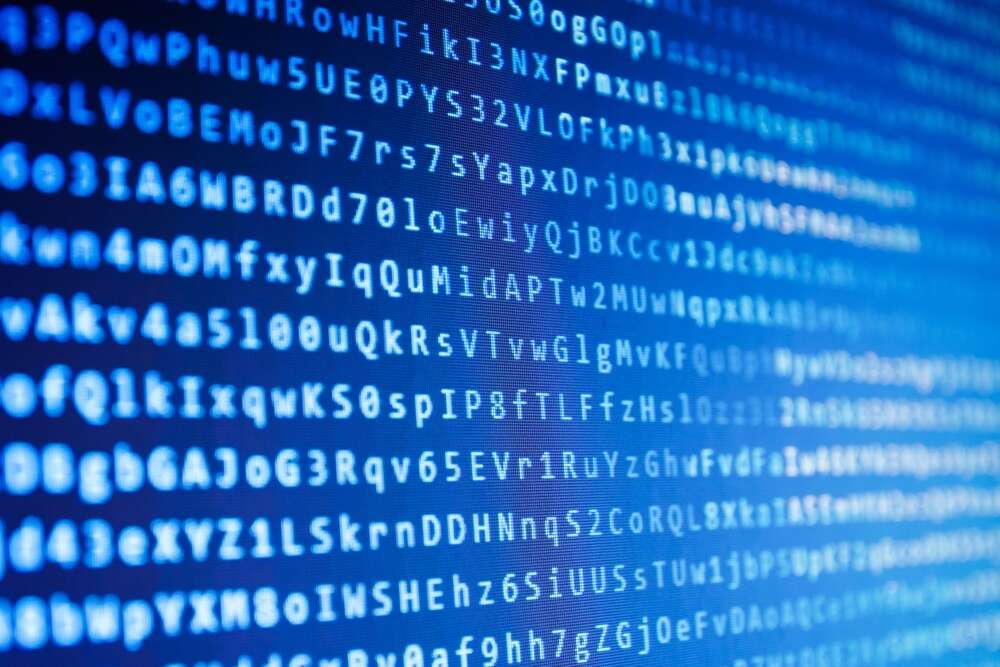
Synthetic datasets are becoming increasingly popular for training artificial intelligence models. Proponents of this computer-generated data say it protects personal information and reduces the chances of bias emerging in AI systems. But for many, concerns over privacy and accuracy remain.

A recent study showed that synthetic data could be reverse engineered to reveal personally identifiable information. (Image by BEST-BACKGROUNDS / Shutterstock)
New use cases for synthetic data are emerging daily. On Thursday, the International Organization for Migration (IOM) charity announced the launch of a synthetic dataset for human trafficking, which has been developed in partnership with Microsoft Research. Taking two years to put together, the dataset is based on records covering 156,000 victims and survivors of trafficking across 189 countries and territories.
Harry Cook, programme coordinator at IOM’s migration protection and assistance division, said the release of the dataset will allow information about the profile of trafficking victims and the types of exploitation to be shared for research purposes, without infringing the privacy and civil liberties of victims.
“Making data on human trafficking widely available to stakeholders in a safe manner is crucial to develop evidence-based responses,” Cook said. “Administrative data on identified cases of human trafficking represent one of the main sources of data available but such information is highly sensitive.”
However, there are doubts about how safe synthetic data really is. A recent paper from researchers in the UK and France found that, in many cases, the process of creating synthetic data does not adequately mask personally identifiable information (PII). These privacy concerns will need to be resolved for the full potential of synthetic data to be realised.
What is synthetic data?
Most AI systems need to be fed with large amounts of training data so that they can deliver accurate results. But for businesses, giving raw customer data to a new system can leave them open to potential privacy breaches.
An unwillingness to share data is a major bottleneck for businesses looking to deploy AI, says Kalyan Veeramachaneni, principal research scientist in the laboratory for information and decision systems at MIT, whose research focuses on making AI and ML more accessible for the industry.
“Access to data is the number one issue and the first problem we encounter in any [AI] project.”
Kalyan Veeramachaneni, MIT
“Access to data is the number one issue and the first problem we encounter in any [AI] project,” he says. “There are contracts you can sign and agreements you can make [to safeguard data] but they can take months to negotiate. It’s a big barrier.”
Synthetic data promises to solve this by taking a sample of real information and generating a larger data set that is representative of the original, but with no PII included. “You take some real data and build a statistical model of it,” explains Veeramachaneni. “You can then use that model to generate an entirely artificial set of data. It has nothing to do with the original data but has the same properties.”
As well as maintaining privacy, bias in AI systems is also something that going synthetic may be able to address. “With synthetic data, you can create a broader distribution [of data points] than you potentially acquire with real data,” says Yashar Behzadi, CEO of synthetic data technology provider Synthesis AI. “That means in the case of potential AI bias, you know that the data feeding into the system is fair.”
Synthetic data is already proving popular with financial services and insurance companies, which are using it to develop systems to detect fraud and enforce anti-money laundering rules, and many sectors are showing an interest too.
“Autonomous vehicle companies are some of the earliest adopters – Tesla and Waymo have already shown off simulation platforms they’re developing and it’s a space where this technology makes sense,” says Behzadi. “We’re also seeing more adoption when it comes to people-focused systems like smartphones. There are business reasons for that but also ethical ones, like privacy and bias, which come into play when smartphone developers are building things like facial identification systems.”
The differential privacy conundrum
While synthetic data promises greater privacy, the reality may be slightly different. A research paper, Synthetic Data – Anonymisation Groundhog Day, from academics at University College London and École Polytechnique Fédérale de Lausanne, found that synthetic data sets could be used to trace back the original information on which the artificial data was based.
The study looks at five synthetic data-generating algorithms and found that it was often possible to deanonymise individual records and reassociate them with actual people, particularly in the case of those who are statistical outliers. “If a synthetic dataset preserves the characteristics of the original data with high accuracy, and hence retains data utility for the use cases it is advertised for, it simultaneously enables adversaries to extract sensitive information about individuals,” the authors conclude.
Professor Emiliano De Cristofaro, head of the information security research group at UCL, is working on a project with the Alan Turing Institute looking at the use of synthetic data in finance and economics. He says this fundamental conflict at the heart of synthetic data has yet to be satisfactorily resolved, as achieving differential privacy – the standard for ensuring individuals within a dataset cannot be identified – is not possible without impacting the usefulness of the synthetic data.
“Differential privacy means that, if you have two versions of a dataset where one record is changed, you shouldn’t be able to distinguish which one the algorithm has run on,” De Cristofaro says. “To do this you have to add some noise to the data, which impacts the data’s utility. That’s a problem because people think synthetic data is like a magic bullet that you can apply in all cases. Its usefulness depends on the type of data that you have, and finding the balance between utility and privacy.”
“People think synthetic data is like a magic bullet that you can apply in all cases. Its usefulness depends on the type of data that you have.”
Emiliano De Cristofaro, UCL
Veeramachaneni believes an adequate level of privacy can be achieved for many business use cases. “A lot of research in this area aims for the ‘North Star’ – use cases where data is released publicly for societal good and can be accessed by anyone, including threat actors,” he says. “For that to be achieved the privacy conditions required would be so strict, and the manipulation of data required would be so great, that you might as well just use a random data model instead.”
But, he says, “for the day-to-day functioning of many enterprise users, data is not going to be released publicly. Here the requirements for privacy can be reduced, and you don’t need to have rules which are as harsh. We can’t have a binary situation where we have to achieve the North Star or there’s no access to data at all, and synthetic data can sit in the middle of the spectrum.”
The future of synthetic data
Synthesis AI’s Behzadi is, perhaps unsurprisingly, bullish about the future of synthetic data. “Google has published papers on how it uses synthetic data in its models and you’re seeing more of the Big Tech companies looking at the benefits of this approach,” he says. “That in turn lines up the dominoes for other companies, the leading AI businesses will come out with better models, and that cascades down to other sectors.
“We’re still in the early adopter phase at the moment, but I would expect more mainstream, non-AI companies start to think about how they use synthetic data, but there’s still a big education element required around how these systems can be used.”
Veeramachaneni says openness about how synthetic data is generated is going to be vital. He and his MIT colleagues have set up the Synthetic Data Vault, a set of open-source algorithms companies can use to develop their own synthetic data sets. “It has to be open because the nature of this software is that people have to be able to look at it before they use it on the real data they are using to create their synthetic model,” he says. “If nobody can verify or validate the data, you just end up with another black box.”