GPT-4. IBM Watson. Dall-E. These large language models look poised to transform how we work and play – and it’d all be impossible without data.
Consider the numbers. According to OpenAI, ChatGPT was first trained on a bewildering 45 terabytes of text data. Encompassing books, articles, web pages and more, that’s the equivalent of nearly 60,000 filing cabinets of paper.
More recent versions of ChatGPT are honed on far less, but with the average large language model (LLM) boasting several billion parameters or more, you can quickly see how desperate Sam Altman and his rivals should be for information.
Not that real-world data is simply there to be farmed. From privacy and copyright fears to simple inaccessibility, securing it can be tricky. Scavenging the wilds of cyberspace for training data turns up trash as well as treasure, obliging flesh-and-blood staff to review datasets before they’re used.
Amid these challenges – and warnings that the global stock of high-quality language data could be exhausted before 2026 – an alternative does exist: synthetic data.
Rather than coming from human sources online, synthetic data sets are created algorithmically. Though typically based on real-world examples, that theoretically allows users to create endless datasets curated to specific tasks and cleansed of inappropriate material.
“One of the things that’s really exciting about synthetic data,” says Alex Watson, co-founder and chief product officer at Gretel, “is it allows you to iterate on your data and address gaps or issues in your data very quickly.”
Yet if it’s already a $300m market, and one expected to enjoy a CAGR of over 45% through 2030, companies should probably reflect before barreling ahead with the synthetic data revolution. Whatever its strengths, after all, it could perpetuate the biases of whatever real-world data it takes as inspiration.
More than that, some experts fear that leaning too heavily on synthetic data could spark something called ‘model collapse,’ whereby generative systems regurgitate patterns they’ve already seen, destroying the very models they’re meant to train.
No wonder, then, that insiders are thinking carefully about how synthetic data should be deployed – and how it may need to be bolstered with real-world information to stay truly useful.
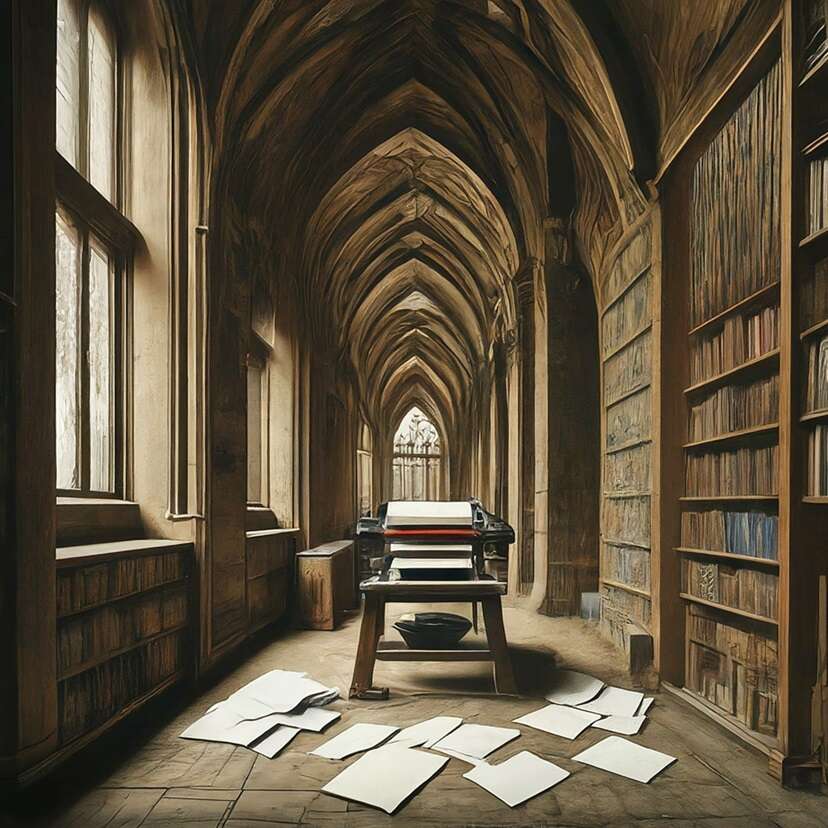
The drawbacks of common-variety training data
Ask ChatGPT a question and the sophistication of its responses can feel miraculous (others less so.) But for Dan Padnos, it’s important to remember that all machine learning is predicated on something tangible: data.
“It’s not magic,” stresses the vice president for alignment at AI21 Labs, an Israeli tech firm, adding that data is a “cornerstone” of LLMs everywhere from extracting knowledge to teaching statistical patterns.
As AI models have developed, tech firms have relied on real-world data to train their models. In Silicon Valley, for instance, Google boasts a ‘Dataset Search’ of 25 million research sets, echoed by a plethora of public and commercial options.
In practice, though, real-world data comes with a range of limitations. That begins with the information itself. Scraped from a bewildering array of sources, some is bound to be irrelevant. Another hurdle, notes Padnos, is that important details may not be included, especially if they’re in unusual formats, buried in some forgotten corner of the web, or else owned by one of the big tech companies.
That’s echoed by the opposite problem: racism and sexism are unfortunate staples of online discourse, and some of that material risks infecting corpora, not least when datasets span billions of web pages.
Given these issues, tech firms are unsurprisingly forced to laboriously curate their datasets. Though tech-specific figures are scarce, one recent study found that data scientists spend 80% of their time collecting, cleaning and processing information – and just 20% actually analysing it.
Beyond the quality of real-world data, Henry Ajder says it’s unclear how it can actually be used. As the generative AI expert explains, “legal ambiguities” around the fair use of online data abound. A fair point: though Japan lately relaxed its rules and the UK dropped its own plans to write a voluntary code of conduct around the use of copyrighted data in training sets, other jurisdictions like the US have left the courts to arbitrate ambiguities – see, for example, the suit filed by the New York Times against OpenAI and Microsoft for copyright infringement after the outlet alleged the tech firms had used its articles to train their models.
Another struggle involves privacy. Mindless blog posts are hardly top-secret – but patient data is clearly rather different, and even when names are anonymised, the specifics of individual cases could still be revealing. Nor is this merely a hypothetical danger: though the case was ultimately thrown out, Google was recently taken to court for allegedly misusing NHS data.

The promise of synthetic data
How could synthetic data overcome these challenges? To answer that question, you must first understand how it works. Ideally, operators will first take what Watson calls a “seed” of real-world data, before using an AI algorithm to generate new datasets from it.
Relying on genuine material should preserve the essential distribution of a corpus – and that’s not synthetic data’s only strength.
Consider, for instance, those knotty privacy questions. Able to purge names and other identifiers, while retaining a dataset’s underlying structures and correlations, Watson says synthetic data can help meet “privacy guarantees” in delicate areas like medicine.
That’s shadowed, Padnos suggests, by its organisational ability. Gathering together information from disparate sources, he describes how algorithms can transform random factoids into “well-organised essays” or even proper textbooks.
In a more general sense, synthetic datasets can support their real-world cousins. Imagine, says Ajder, that medical researchers want to train a model to distinguish benign and malignant tumours, but are struggling to find enough edge cases.
As Ajder explains, synthetic data can plug the gap, while the personalisation that implies can also ensure models aren’t exposed to offensive or otherwise inappropriate material. Add its ability to neatly sidestep some (but not necessarily all) copyright concerns, and it’s easy to see why synthetic data is so popular.
Once again, the numbers here are revealing, with work by Gartner suggesting that 60% of all the data used in AI and analytics projects this year will be synthetic.
You can spot similar excitement at specific companies too. Microsoft, Meta and Google are just three of the giants to embrace the technology, even as firms like Gretel offer APIs to generate safe and robust data fast. For Watson, that could “democratise access” to quality information – encouraging innovation from manufacturing to education.

Jackrabbit disease
Given Google alone handles some 40,000 petabytes of data each and every day, broadening access feels eminently reasonable.
All the same, the future of synthetic data isn’t entirely bright. To an extent, worries stem from the same real-world data that algorithmic alternatives hope to replace. “You can’t put in nothing,” says Padnos, “and expect to get something.” In practice, that means the biases of the input data can be replicated by the synthetic output, ultimately obliging users to thoughtfully curate each corpus just as they’ve always done.
And if that leads Ajder to warn against seeing synthetic data as a panacea, he has wider anxieties too. That’s particularly true when it comes to so-called model collapse. This is essentially when synthetic data models are trained on their predecessors, something that can potentially lead to models forgetting the fundamental statistical distribution they were trained on. From there, they risk ignoring important information and or producing muddled or homogeneous results.
There are various analogies used to explain this phenomenon: for his part, Ajder compares it to the famous Goya painting of Saturn devouring his son. In practice, at any rate, a recent academic paper vividly shows how damaging this could be. Using a tract on religious architecture as its input, the first iteration already showed signs of confusion, referencing a non-existent London cathedral. Things devolved from there: by the ninth generation, the model was ranting about jackrabbits.
Given the remarkable spread of synthetic data, such a scenario could hamstring tech projects, especially ones without the funds to secure alternative data sources. Yet if some scientists reject that synthetic data necessarily trends to disaster, industry insiders seem equally conscious of the threat.
“With the stakes so high, it’s a concern that everybody’s aware of,” says Padnos, adding there’s plenty tech firms can do to mitigate against model collapse. Perhaps the most obvious tactic is leavening synthetic data with its real-world cousin, ensuring models don’t get lost in abstraction. Another involves exploiting synthetic data’s famed adaptability by tweaking algorithms to ensure biases aren’t reinforced.
Whatever synthetic data’s current popularity, however, some industry players are continuing to hedge their bets. It may, after all, be leveraging computer-made data in its models. But OpenAI equally continues to invest in real-world information, gaining access to media archives from Bild to the Financial Times. Despite its strengths, in short, don’t expect synthetic data to completely replace the genuine article.