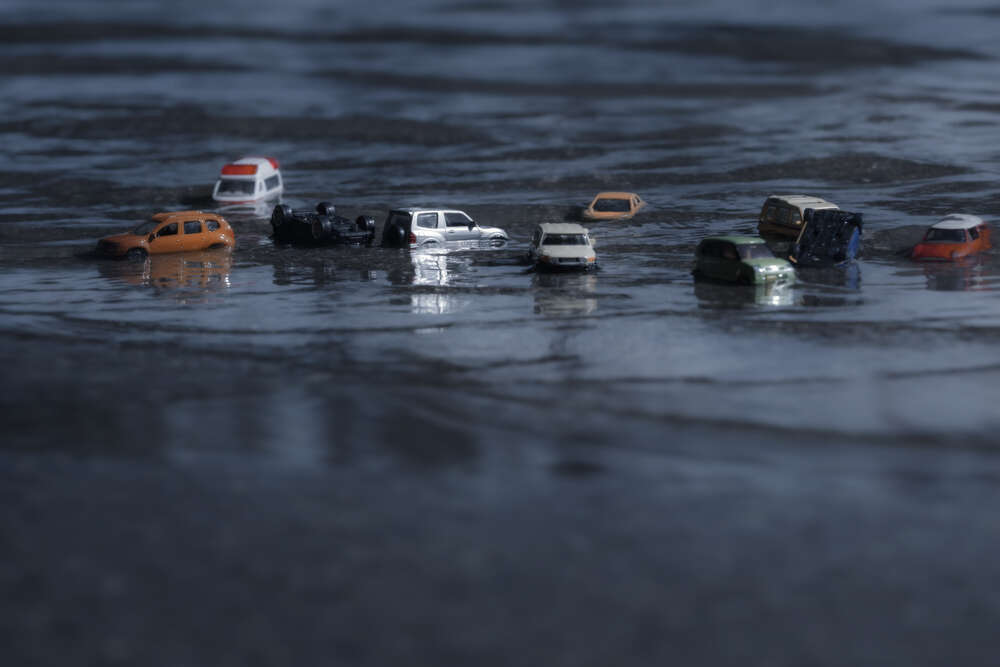
This is an amended version of the latest edition of Quantum Untangled, Tech Monitor’s weekly newsletter that delves into the practical implications of quantum computing. Subscribe here to get it delivered to your inbox every Thursday morning.
By the time you’re reading this, it should be raining in Cornwall. If the UK’s Met Office’s predictions are correct, this will not be ordinary rain — that, after all, is no great revelation for this country in deepest, darkest November. No, this will be unusual rain, menacing rain, rain that falls not in dribs or drabs or pitters and patters or in the fashion of your typically sodden English winter but sheets and needles, thrown onto the ground and into the skin by gales running at cyclonic speeds. The rain will fall and the gutters will fill and the rivers running through Cornish granite valleys will burst their banks, carrying sewage and tree branches and the unmoored belongings of thousands of people past sandbags and sea walls along a new and uncertain course.
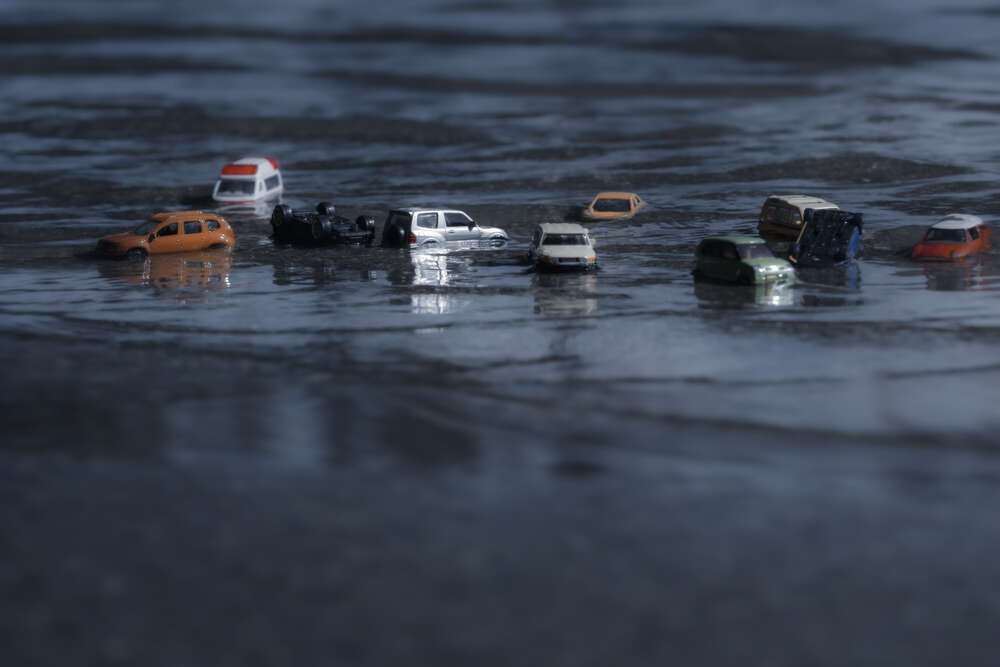
This vision of Storm Ciarán has already appeared in the Met Office’s forecasting models, powered by some of the world’s most powerful supercomputers. But what these models cannot always predict are the details. Though classical supercomputers and deep learning models are perfectly capable of parsing billions upon billions of data points to predict what the weather will be like across a wide space, that task inevitably becomes more difficult as they zoom in on a city, a town or a village. More and more variables come into play, conspiring to complicate forecasting weather in the coming hours and days and resulting in mistakes — predicting rainfall instead of sunny spells, disaster instead of tranquillity.
There are ways to solve this problem of precision — ways that many a company would like to harness for their own ends — and as Tech Monitor has previously explored, they usually involve scaling up supercomputers to ever greater sizes or using deep learning models run by big tech giants. Quantum is another contender. By harnessing the power of even a handful of qubits, its advocates argue, the computational capacity of forecasting models will be launched into the (purely metaphorical) stratosphere, resulting in predictions of such accuracy and delivered with such speed that they will, in time, acquire the authority of prophecy.
Those experimenting in the space are starting a little smaller than that. Multiverse Computing, for example, has confined its ambitions to pairing quantum hardware with classical computing infrastructure to demonstrate how the former might be used to quantify flood damage. Normally, that requires building simulations that rely on solving Shallow Water Equations, so-called because they helpfully predict the flow of water over a given area using very complicated mathematics. It’s a computationally intensive operation for even classical supercomputers, but by running a quantum physics-informed neural network (QPINN) algorithm on variational quantum circuits (VQCs), the team hopes to demonstrate how quantum tech might be more efficient and accurate in solving these kinds of problems.
The consortium will spend the next few weeks proving that this is possible to the satisfaction of the UK’s Department for the Environment and Rural Affairs (Defra), which is overseeing the project under the overall auspices of the UK government’s Quantum Catalyst Fund. If they can prove their system works, explains Multiverse Computing’s chief of sales Victor Gaspar, the second phase will involve spending the next 15 months working out how to practically integrate the system into new forecasting workflows, with Defra being the intended end user. At first, the consortium will rely heavily on classical computing hardware to run its quantum algorithms but, as the field of quantum computing matures, “we could use more qubits and increase the part played by traditional quantum systems” in the overall framework.
It’ll also be incredibly beneficial in working out how to use quantum hardware to crack other conundrums in fluid dynamics, explains Gaspar. Such problems abound, after all, across a range of other sectors, where researchers spend years of their lives perfecting models that predict the subtle movements of air, oil and water through space.
“In the end, all of those problems are CFD problems,” says Gaspar. If Multiverse and its collaborators successfully demonstrate the feasibility of using VQCs to help sharpen flood risk assessments, he adds, “this shows that this same algorithm, and this same approach, could be used to tackle some other types of CFD problems. And then, the opportunity is massive.”
Quantum-powered weather forecasting
Gaspar and his colleagues are hardly alone in experimenting with quantum computers in meteorological applications. Last year, chemicals giant BASF and French quantum startup PASQAL collaborated on a project exploring whether quantum algorithms might be used in forecasting applications in the near future. The Met Office, meanwhile, has expressed its own interest in seeing “how quantum computing can be applied to [its] simulated systems.”
Professor Tim Palmer is sceptical that these experiments will bear fruit. A Royal Society Research Professor in Climate Physics at the University of Oxford, Palmer has some grounding himself in quantum mechanics, having written his PhD on the mysteries of quantum gravity. But that’s left him a sceptic when it comes to assessing the viability of quantum computers in anything related to weather forecasting. “You get these articles from time to time on the web of groups or companies claiming that quantum computers will be used for weather and climate prediction,” says Palmer. But, he says a little later, “I think there’s an awful long way to go before you could actually do it in practice.”
Some of the obstacles are less obvious than others, as Palmer explained in a recent paper he co-wrote on quantum computers in weather forecasting for the Bulletin of the American Meteorological Society. As it turns out, the fact that quantum computers perform much better when handling linear over non-linear equations is not, says Palmer, “a showstopper,” and neither is the incessant noise that normally interferes with measuring readouts from qubits in most contemporary quantum machines. “One of the interesting things about weather and climate forecasting is that we actually add noise to the models to make them more realistic,” he says.
The problem, Palmer continues, is “when you’re trying to read the data back out.” Your future, 30-qubit quantum computer would probably be able to ingest the billion or so data points handled by the average weather model: with a “state space” in the order of two to the power of 30, you’d ask for your money back for anything less. However, the practicalities of measuring the qubits mean that it’s unlikely you’ll be able to do anything more than ask the computer a small number of binary questions with yes or no answers based on the data inside. In other, more mathematical language, “the measurement problem collapses the state space back down again,” all the way from a billion down to 30 qubits.
As such, says Palmer, “you can frame your calculations such that, when you measure one of these qubits, it tells you the answer to some aspect of the atmospheric state where the answer is yes or no,” but you’ll have used up one qubit in asking that question. Constructing a weather map of the UK, meanwhile, requires asking millions of such binary questions, the answers to which Palmer believes even an advanced quantum computer will prove unlikely to be able to deliver. It’s also a problem Palmer anticipates when using quantum computers to solve problems in fluid dynamics.
“The same objections apply,” he says. “Where the output is big data, the nature of the measurement problem in quantum mechanics, the collapse of the wave function, if you like, is just going to stop you from doing that.”
Multiverse Computing’s chief scientific officer politely disagrees. “The art of designing quantum algorithms is in dealing with the wave function collapse,” Román Orús tells Tech Monitor over email. “Many seminal algorithms, such as Shor’s algorithm, use constructive interference to amplify interesting outcomes, such that the model is not penalized by the size of the state space. The wave function collapse is not a problem, but rather a resource in quantum computing. Otherwise, how could other algorithms with mathematically proven speedup work at all?”
Orús also argues that, while there are problems encoding big data onto a quantum computer, “there are also methods for circumventing this issue for quantum algorithms” in the literature.
“Finally, and most importantly, while quantum computers will likely be the most efficient way to tackle climate models in the future, we believe that the most competitive solutions today are offered by quantum-inspired models, i.e: ideas taken from quantum physics which can accelerate HPC [high-performance computing] today. In previous work, we have shown these techniques can be used to solve complex systems of partial differential equations, including intractable models of physical relevance. Other work has recently shown these approaches can also solve the Navier-Stokes equations and provide accurate simulations of turbulent flows.
“We want to emphasize that, as we believe quantum computing will in the long run be superior to quantum-inspired approaches, it is necessary to work on both approaches in parallel.”
Palmer remains unconvinced. “Even if we can solve things like the Navier-Stokes equation,” he says, “it’s not at all clear that that could be done exponentially faster than a classical computer. That’s still to be proved.”
While Palmer concedes that quantum computing might prove useful in more niche meteorological applications, where it’s desirable to find a definite answer to an incredibly specific, computationally intense problem, he claims that there’s much more buzz about that prospect in the quantum startup community than among his own colleagues in climate modelling, who remain much more excited about the unrealised potential of supercomputing and deep learning in weather forecasting. Those figures in the latter world who are excited about quantum computing, he says, often do not understand the fundamental challenges at play.
“One of the reasons I wrote this paper with my postdoc is that I remember somebody once came up to me and said, “Oh, it’s great: once somebody has written a quantum-Fortran compiler, we’ll be able to use these computers, so when’s it going to happen?”,” recalls Palmer. “And I’m saying, it’s not like that: you don’t understand the nature of the problem. It’s not just [about] finding a new type of language, like Cuda for GPUs. It’s not going to be like that. The nature of the problem is just radically different and most people, unless they have a good background in quantum theory, don’t really understand that.”