
The complexity of weather systems is too great for conventional machine learning. (Photo by Shutterstock/elRoce)
The UK’s Meteorological Office was the first organisation in the world to deploy what would now be termed a supercomputer. Speaking this week at the New Statesman Media Group‘s CIO Symposium, CIO Charles Ewen shared how the organisation stays at the cutting edge of computing to wrestle with the inherent complexity of weather systems and inform life and death decision-making.
“The Met Office was the first organisation to deploy what you would consider to be an operational supercomputer in the UK, back in 1958, and we’ve done 13 since,” Ewen explained. “Each one brings different characteristics, capabilities, foibles and idiosyncrasies to the table.”
The agency currently uses three Cray XC40 supercomputing systems. These are capable of 14,000 trillion arithmetic operations per second and have a combined 460,000 processor cores, 2 petabytes of memory and 24 petabytes of storage.
Impressive though that may sound, the Cray fleet will be retired in 2022 to make way for the next generation of supercomputing. Earlier this year, the UK government announced a £1.2bn investment to ensure the Met Office can continue to rely on state-of-the-art weather modelling systems.
“We’re in the final throes of justification, specification and submission for funding of that supercomputer that’s been underway for the last couple of years,” explains Ewen. “And we’re just in that transition point of identifying the potential vendors. That’s a massive thing for the organisation and a lot of other decisions are dependent upon it.”
How supercomputers are designed for maximum performance is always evolving, Ewen explains. In recent generations, the focus was on using the most high-powered processors available. Now, though, distributed architectures that use a greater number of less-powerful chips are making a comeback.
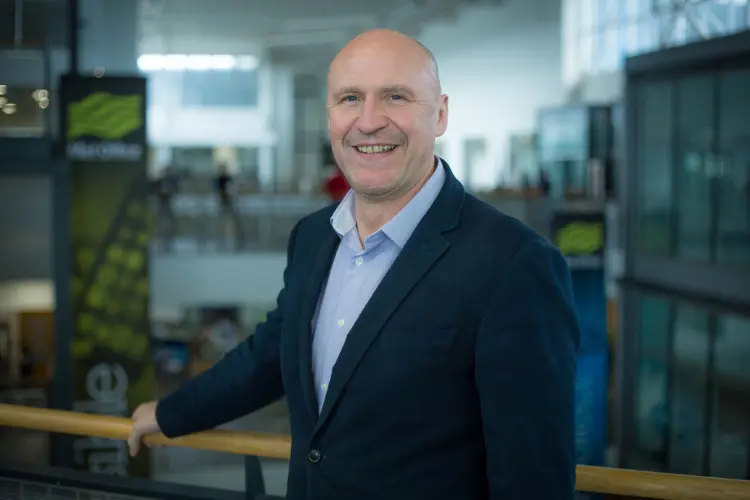
Charles Ewen, chief information officer and director of technology, Met Office. (Photo courtesy of Met Office)
“We’ve come from a period where there used to be a lot of diversity in architectures that were plausible to use for our kind of work, to a period where it has become very centred on CPUs,” he explains. “Now we’re beginning to see that broaden out again.”
Crucially, he says, processors based on designs by the UK’s ARM Holdings, which draw less power than comparable alternatives, are increasingly viable for supercomputers. That is especially important for the Met Office: as a direct witness to climate change, it is committed to reducing its carbon footprint.
“A big driver [of carbon emissions] is the supercomputer itself and where the energy comes from. We’ve just had the opportunity to reassign our electricity supply contracts to carbon-neutral sources. That takes us a long way down the road to our carbon neutral policy.”
The growing need for weather prediction
The Met Office uses its supercomputers to forecast the weather. This is not just to help the British public decide whether they need an umbrella, however: a growing number of life and death decisions rest on the accuracy and timeliness of its forecasts.
For example, airlines need accurate weather forecasts to fuel their aircraft appropriately. “When an aeroplane takes off from North America, it relies on the jet stream in part to get it to the UK,” Ewen explains. “Often the plane would not have enough fuel to get it there in dead air, so it’s reliant on those back winds in the jet stream that flow across when the plane is in flight to help get you there.
“So to calculate the amount of fuel that goes into that plane – that decision is made based on how the atmosphere is going to behave, which is based upon the simulations that sit on the supercomputer.
Smooth running of the UK’s railway system also relies on the Met Office’s forecasts – which extend beyond predicting the weather alone. “Trains don’t run because of leaves on the line,” Ewen explains. “So we help the rail operators, for example, by helping them understand how many leaves will be on the line. And that’s not simply a weather forecast: that [requires] understanding of what the winds are going to look like, whether the trees are dropping, shedding their leaves.
As vehicles become automated, Ewen says, these predictions will become all the more vital. “An area that we’re now active in is research on autonomous vehicles,” he says. This is a transition between people making decisions, to machines making much of the same decisions.
“One of the things that changes the game is a good understanding of the environment within which that autonomous vehicle is operating,” he explains. “A distance sensor on a car could easily be impacted by slushy road conditions and may not be safe as a sensor anymore, in which case the vehicle must accommodate that,” by slowing down and taking greater care in its decisions.
The Met Office’s predictions are also helping to tackle climate change by forecasting the output of renewable energy sources such as wind and solar. “We help on all aspects of that; where to put a wind farm with climatological analysis of where there are going to be the right winds.”
Why the weather is too complex for machine learning
The ability of computers to make predictions – the core project of the Met Office – has made great strides in recent years, thanks to breakthroughs in machine learning and artificial intelligence. But conventional machine learning methods are of little use for predicting a system as complex as the weather, says Ewen.
“A question that’s often asked is why can’t you simply use a machine learning algorithm running in the public cloud to predict the weather? The core reason is all to do with something that was enumerated by a chap called Edward Lorenz, who worked for the Met Office for a bit back in 1964.”
Lorenz was a meteorologist who described chaos theory, the idea that in sufficiently complex systems, tiny changes can have an enormous impact. “The environment, the weather, exhibits chaos. It doesn’t matter how well you train an algorithm to recognise what happened in the past, if you start from precisely the same measurable point, the situation will evolve differently because of chaotic factors.”
This means that conventional machine learning techniques, which extrapolate trends from prior datasets, are unable to anticipate weather patterns with the accuracy the Met Office demands.
Instead, the Met Office uses its supercomputers to simulate the near-future of the weather system multiple, each with tiny variations. “Rather than take our best guess, which is what we used to do back in the day, we now run something called an ensemble prediction system. So what that means is we don’t run the same forecast just once. We’ve run it many, many times. And each time that we run it, we slightly change the start state of the simulation.
As opposed to a single forecast, this technique produces a distribution of likely weather outcomes. “Sometimes… there are minor variations in what we think the weather is going to do in three days. Sometimes, however, it will run the same forecast 50 times and the outcomes will be very different. And so that gives us statistical information on the reliability of our weather forecast.”
When it comes to complex systems such as the weather, Ewen argues, this probabilistic approach to decision making is the gold standard. “We live in a deterministic world. We say yes or no to things. We turn left and right. But because of the characteristics of trying to determine whether the weather is not deterministic, to predict it, we have to be probabilistic.”
The evolution of supercomputing should therefore be accompanied by a cultural shift in the way organisations use information. “The big thing is… turning heavily probabilistic information into things that enable decision making,” says Ewen.