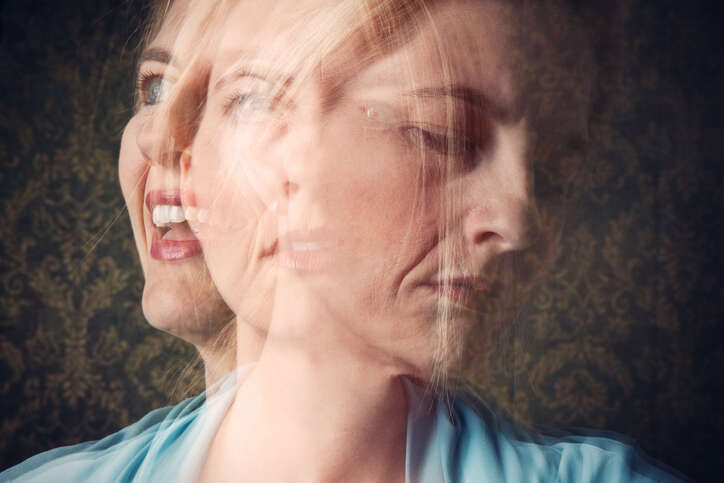
Have you ever been angry at your computer? Rosalind Picard knew plenty of people that had. There were those minor cases, of course: a slap on the chassis, say, or the shouts of frustration when software was taking too long to load. Then there were the more extreme examples. “I love the story of the chef in New York who threw his computer in a deep-fat fryer,” the MIT computer science professor told Wired in 2012. “There was a guy who fired several shots through the monitor and several through the hard drive. You don’t do that because you’re having a great experience.”
What the world needed to prevent these outbursts, Picard reasoned, weren’t just faster computers, but ones capable of anticipating the build-up of frustration through the analysis of contextual signals: the furious tapping of a mouse, say, or the haptic feedback from a keyboard being prodded harder and harder.
In 1995, she debuted this vision of machine learning in her book Affective Computing. In it, Picard envisioned a future where artificial intelligence would be capable of interpreting anger and frustration in the user, but also the expression of all types of emotions – leading not only toward more intelligent product design, but new applications in medicine, learning and in the creative arts.
Since then, affective computing has acquired another name: emotion recognition, or ER. Recent years have seen numerous applications for ER emerge, from using facial analysis to spot when drivers are on the brink of dozing at the wheel, to assessing the suitability of job candidates during interviews or by staff at call centres to obtain early warning of customers who sound particularly irate.
Along the way, though, these systems’ capabilities seemed to inflate. Many weren’t just claiming that they could analyse physical expressions, but also that they could use this data to infer an interior emotional state – in other words, know exactly what the user was feeling.
For many academics, this was ludicrous: a smile, for example, could denote deep-seated frustration as it does happiness. A backlash against ER quickly developed. Leading the charge was psychologist Lisa Feldman Barrett, who explained in a review article she co-authored in 2019, the dangers of drawing such profound conclusions from the curve of a brow or the intonation of someone’s voice.
The following two years saw this debate spill over into mainstream coverage, using the premise that ER was inherently unreliable to question whether it was inflicting undue emotional labour, preventing people from being hired, wrongly identifying people as criminally suspicious, or being used to oppress Uyghurs in Xinjiang. The apotheosis of this debate, however, came in an article in The Atlantic that not only attacked ER as fundamentally flawed, but charted a link between these applications and the controversial theories of psychologist Paul Ekman, who claimed that certain emotions are expressed in universal ways.
Perhaps that should have been the end of it. Indeed, some tech companies, like Microsoft, have walked back prior endorsements of ER, while Hirevue has suspended its use of the technology to assess job candidates. But others haven’t. In April, Zoom stepped into the field of emotion recognition software when it announced its intention to incorporate AI models capable of analysing user engagement during video calls, while a US start-up called EmotionTrac claims its facial analysis software can help law firms assess how juries might react to certain arguments (neither company responded to interview requests).
In the face of such excoriation by the academic community, continued interest in these applications seems baffling. But so far, the allure of supposedly “mind reading” technology, and the simple intuition that our emotions are reflected in our faces, are proving to be more persuasive than the nuanced argument that debunks it.
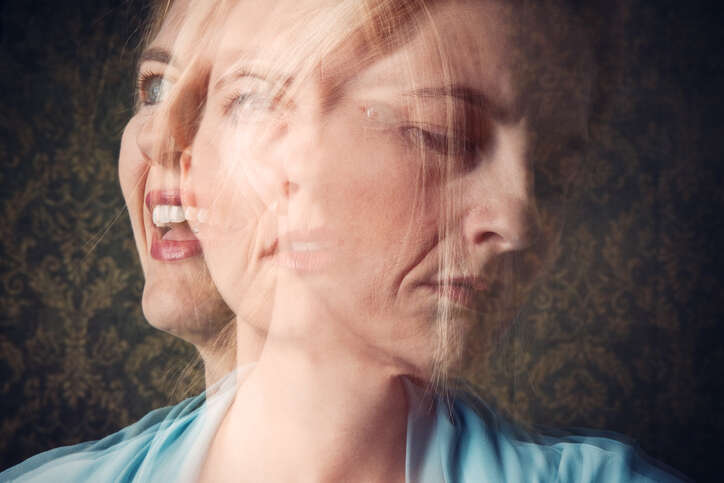
Does emotion recognition work?
It was never meant to be this way, says Picard. Her work at the Affective Computing Lab and Affectiva, a start-up she co-founded in 2009, wasn’t aimed at reading people’s minds, but instead attempted to automate the very human action of looking at someone’s face and guessing how they were reacting to a situation in the moment. Using those signals to infer an interior emotional state, she explains, is a step too far.
“I might see you nod, and I think, ‘Okay, he’s trying to look like he’s paying attention,’” says Picard. “Does it mean that you’re happy with what I said? No. It’s a social gesture.”
Context is key when making these judgements. Just as an interviewee can guess correctly that a journalist is nodding their head is a sign that they’re listening, so too does an AI need to be aware of precisely what situation it is being asked to judge the reactions of an individual.
This was precisely how Picard wanted Affectiva to apply affect recognition in ad testing (she eventually left the firm in 2013 and founded her own start-up, Empatica). Companies including Coca-Cola or Kellogg’s have used its technology to look for outward signs that a commercial is funny or interesting, findings which are then benchmarked against self-reported data from the subject. But using the software outside of those constraints, explains Picard, would see its effectiveness diminish dramatically.
The limits of emotion recognition AI
When taken in context, emotion recognition technology can be of use, says Andrew McStay, professor of digital life at Bangor University. “Even some of the most vociferous critics will kind of agree that, if you understand an expression in context, in relation to a person – who they’re with and what they’re doing, and what’s occurring at that time – there is greater confidence in the result.”
Even so, there’s a ceiling in how effective even this approach can be: the subject might have a muted reaction to an advertisement because they’ve had a bad day, for example. The essential unknowability of how that wider context influences an individual’s outer expressions means emotion recognition AI can only ever provide limited insight – something Picard herself acknowledges.
“In my book, I said you need context, you need the signals, and you can still be wrong,” she says. “Because it’s not the feeling.”
But even burying ER systems within larger contextual frameworks has its downsides, argues Os Keyes, a researcher into technology ethics at the University of Washington. Even if its contribution is small, an ER component drawing the wrong conclusions from physical expressions of affect can still contaminate that larger system’s decision-making process. “Some human, somewhere, has decided that 65% is the threshold for, 'this is true or not,’” he explains. “If you are at 64%, and emotion recognition brings you to 66%, there is a different outcome.”
Physical affect also varies according to the nationality of the individual. Even the cultural biases of AI researchers themselves can’t help but influence the output of their systems. “If you're looking at eye movements, or body movement, or facial muscle movements…I’m sure there is something they can tell you,” says Shazeda Ahmed, a researcher at Princeton University and an expert on ER applications in China. “But, it's very hard to design systems like this and not having imposed your cultural presumptions about what emotion is, how you define it.”
As a field, emotion recognition also suffers from a basic problem of semantics. For scientists like Picard, ‘emotion’ is a technical term used in reference to the uncertain analysis of physical affect. Most other people define emotion as an all-encompassing feeling – making it much harder conceptually to untangle physical expression from mental states. “I’m partly to blame for this,” says Picard, conceding that another term, such as ‘affect processing’, might have proven less controversial.
It doesn’t help that there’s no agreement in psychology about what terms like ‘affect’ and ‘emotion’ actually mean. Picard originally approached dozens of psychologists about how best to define the outputs under scrutiny by facial analysis software. What she found was a balkanised field where researchers jealously guarded the dogma of their sub-theories of emotion. “It’s very antagonistic,” says Picard. “You plant a flag, you stand by that flag.”
That lack of consensus might explain why so many start-ups take inspiration from Paul Ekman’s theories about the universalities of emotion, explains McStay. In the many technology conferences he’s attended, he says, “there’s a deep focus on technical method rather than psychological method,” focusing disproportionately on the latest advances in computer vision instead of the limitations and ethics of analysing affect.
In that context, “the Ekman model works really well for technologists,” says McStay, insofar as his idea that certain emotions are innate and have common expressions imply that machines can be trained to identify them.

The allure of emotion recognition technology
If people understood that emotion recognition isn’t capable of making a judgement about internal emotional states, says Keyes, the technology would be a lot less popular – even if it was embedded within a larger framework of contextual data. “If I make you a coffee and I tell you that it has 15 ingredients, one of which is rat shit,” they say, “do you feel more comfortable about drinking that coffee?”
Despite this, the allure of emotion recognition remains strong – and not just among technology start-ups. Having spent several years studying emerging ER applications in China alongside Shazeda Ahmed, lawyer and researcher Vidushi Marda is regularly invited to address authorities in India and the EU about the technology and its limitations. In almost every conversation, she’s had to try harder than she thought to convince her audience that ER is flawed.
“The knowledge that these systems don't work isn't compelling enough for governments to not throw money at it,” says Marda. “The allure of power, and computational power, is so high when it comes to emotion recognition that it's almost too interesting to give up, even though there's overwhelming scientific evidence to show that it actually doesn't work.”
This could be because it’s just easier to argue for emotion recognition rather than against it, Marda continues. After all, people do tend to smile when they’re happy or frown when they’re angry, and it’s a simple idea to imagine technology capable of interpreting those signals.
Explaining that physical expressions or intonations of voice do not always correspond with an interior state of mind is a more complicated argument to make. “I think we can talk about Ekman until the cows come home,” says Marda, “but if people still believe that this works, and people still watch shows like The Mentalist, it’s difficult to fully get them on board.”
It’s little wonder, then, that the impassioned arguments of Barrett et al haven’t stopped technology companies trading on the inflated expectations of what ER can achieve. Emotion Logic, for example, has sought $10m from VC firms to bring to market “an AI engine that understands emotions and cannot only relate to what a person says, but also what the person really feels,” according to the CEO of its parent company. And while Picard maintains that she was clear with clients during her time at Affectiva that the insights to be gained from audience reactions were inherently limited, the company states on its website that its Emotion AI service is capable of detecting ‘nuanced human emotions’ and ‘complex cognitive states’.
In most places around the world, these companies operate in a regulatory vacuum. What would help, says Picard, are laws requiring fully informed consent before such systems are used and banning its operation in certain circumstances while safeguarding others, most obviously in healthcare applications like helping people with autism to interpret basic emotions (though Keyes points out that this latter use has also inflated expectations of what ER can achieve.)
A useful template, Picard explains, might be found in the polygraph, a machine of which the use is restricted in US law precisely because its effectiveness is highly contingent on the context of its operation. Even then, she says, “we know that there’s even trained polygraph people [that] can still screw it up.”
Getting to that point, however, will require a concerted effort to educate lawmakers about the limitations of ER. “I think even people like me, who would rather do the science than the policy, need to get involved,” says Picard, “because the policymakers are clueless.”
Reform on this scale will take time. It may not be possible until the public, and not just academics, believe the technology is failing in its goals, explains Nazanin Andalibi, a professor at the University of Michigan. That’s made even more difficult by the invisibility of failures not only to those having their emotions ‘recognised’ but also those using the services, given the lack of transparency on how such models are trained and implemented.
Meanwhile, the “processes of auditing these technologies remains very difficult,” says Andalibi. This also plays into a groundswell of hype around artificial intelligence in recent years, where the emergence of voice assistants and powerful language models give the lie that neural networks are capable of achieving almost anything, so long as you feed them the right data.
For his part, Keyes is convinced technologists will never get that far. Developing an AI capable of parsing all the many nuances of human emotion, they say, would effectively mean cracking the problem of general AI, probably just after humanity has developed faster-than-light travel and begun settling distant solar systems.
Instead, in Keyes’ view, we’ve been left with a middling technology: one that demonstrates enough capability in applications with low-enough stakes to convince the right people to invest in further development.
It is this misunderstanding that seems to lie at the root of our inflated expectations of emotion recognition. “It works just well enough to be plausible, just well enough to be given an extra length of rope,” says Keyes, “and just poorly enough that it will hang us with that length of rope.”