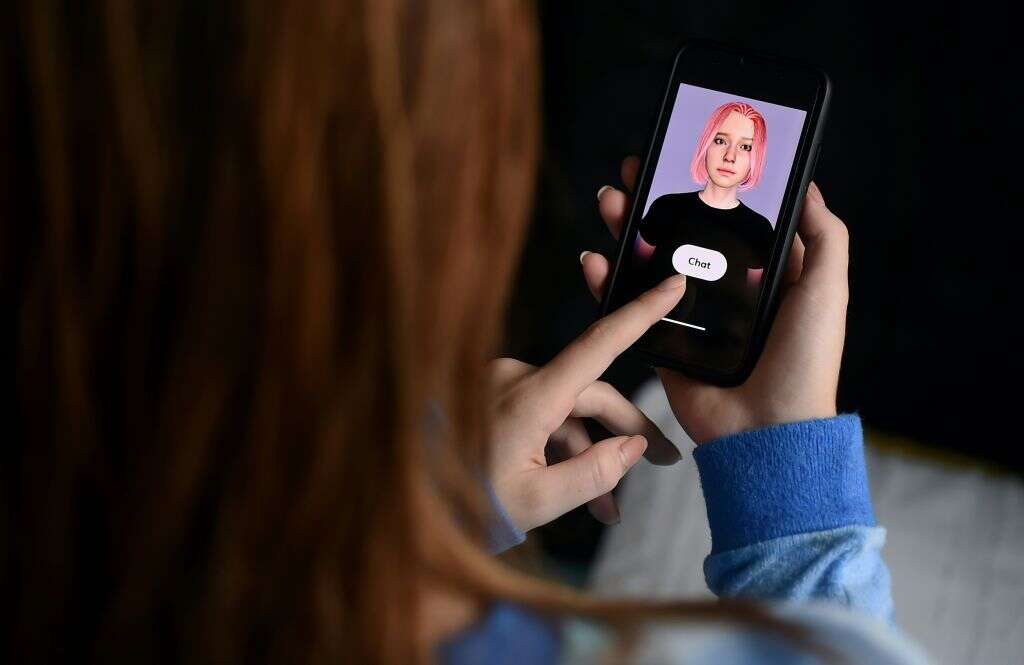
One of the most famous philosophers of the twentieth century, Bertrand Russell, was fixated on the problem of how humans gather knowledge. In his efforts to pick apart our truth-seeking methods, he denounced inductive reasoning – generalising a rule from a large number of observations – as one of the core “problems of philosophy”. Russell used a neat illustration to demonstrate the limits of this approach.
His example involved an ‘inductivist turkey’. Every day on the farm, this turkey was fed at 9am, come rain or shine, summer through autumn. Finally, the consistency of the morning feeding was sufficient for the turkey to conclude through inductive inference: “I am always fed at 9am.” Of course, the turkey’s conclusion was invalidated when on Christmas eve, instead of being fed, its throat was slit.

What might seem an arcane thought experiment is startlingly relevant to the problems faced by artificial intelligence today, argues a new book The Myth of Artificial Intelligence: Why computers can’t think the way we do by Erik Larson. The book posits that not only are we nowhere near achieving human-like artificial intelligence, but AI powered by inductive reasoning will never get us there. Even worse, no one really knows what a better approach might be.
The quest for artificial general intelligence
Another way of describing human-like artificial intelligence is ‘general intelligence’. It’s a slippery concept to define, and some AI researchers argue the two are not interchangeable. But roughly speaking, it’s artificial intelligence that can be applied to many different problems. Right now, DeepMind’s AlphaGo can beat a human Go champion with ease, but ask it to turn its hand to driving a car, or even playing chess, and it will be stumped. The quest for general intelligence seeks to solve this.
AI is bubble-wrapped in hype, where the next big breakthrough is always “five years away”. But Larson cautions that without achieving something approximating general intelligence, the field could soon stall. Take self-driving cars. “In 2016, I remember thinking ‘why is Elon Musk and company declaring victory on self-driving cars?’ and five years later, all of a sudden, you don’t hear about them. And there’s a reason – because they were running into obstacles that aren’t surmountable using the AI systems on board.”
Larson has worked in AI since 2000, primarily on natural language processing using machine learning, and is the founder of two Darpa-funded AI start-ups. He says the mismatch between AI futurist hype and the nuts and bolts of what’s happening on the ground has plagued the field since the 50s, when it was first getting started.
Larson has always possessed a “kind of philosophical scepticism” about the possibility of making a computer with something akin to a human mind. But when he first started in the field, the web was exploding into existence, the data sets were expanding by a factor of ten, and there was excitement in the air. “Methods that didn’t used to work very well in the 90s – suddenly, they started working in the 2000s,” he says.
His sceptical turn came gradually. First when he realised that “more data” wasn’t going to answer AI’s central challenges. Then, while working for his own company, he realised that despite doing fruitful and interesting work, “this idea that we were developing general intelligence artefacts for machines – that just never transpired.”
“All the talk that you would hear from the futurists, there was just this disconnect,” Larson says. He attended conferences where evangelists giddily described the proximity of a machine superintelligence. But he was working with cutting-edge AI at the time and was well aware the rhetoric didn’t stand up. “I just couldn’t figure out why they were saying that… you don’t hear people running around in physics saying that time travel is just around the corner, that there’s a couple of theoretical obstacles we have, but they’ll be overcome.”
You don’t hear people running around in physics saying that time travel is just around the corner, that there’s a couple of theoretical obstacles we have, but they’ll be overcome.
Erik Larson
The view that the abilities of AI have been oversold is not uncommon among AI experts. Principal researcher at Microsoft Research Kate Crawford’s new book Atlas of AI argues that AI is “neither artificial nor intelligent”. “AI systems are not autonomous, rational, or able to discern anything without extensive, computationally intensive training with large data sets or predefined rules and rewards,” she writes. “In fact, artificial intelligence as we know it depends entirely on a much wider set of political and social structures.”
Abductive reasoning
For Larson, the issue comes down to the types of reasoning that computers can master – deductive and inductive reasoning. Deductive reasoning is a rules-based way of communicating knowledge about the world, typical of older AI programmes. Inductive reasoning is what machine learning programmes mostly rely on. This is why today’s AI excels at capturing regularities in massive data sets, and, like Russell’s inductive turkey, making predictions based on past observations: posing Netflix recommendations, surfacing content in Facebook timelines, or adeptly identifying photos of human faces or pets.
“By working on both sides of the coin, the traditional rule-based approach, and the inductive or machine learning approaches, it became clear to me that we were just missing something central,” says Larson. His book makes the case for a third kind of reasoning, of which our understanding is more limited: abduction. Unlike induction, which is raw empiricism, abduction accounts for some of the more mystical manifestations of the human mind: instinct, common sense, intuition.
Larson’s book uses scientific breakthroughs as an example of where abductive reasoning comes to the fore. The most brilliant and world-realigning breakthroughs have tipped previous understanding on its head. “Like Turing once did, students of scientific discovery tend to push such intellectual leaps outside the formalities of scientific practice, and so the central act of intelligence “rides along for free,” unanalysed itself,” Larson writes. “But such hypotheses are genuine acts of mind, central to all science, and often not explainable by pointing to data or evidence or anything obvious or programmable.”
He uses the example of Nicolaus Copernicus, the Renaissance-era mathematician and astronomer, who heretically posited that the earth revolved around the sun and not vice versa. To do so, “he ignored mountains of evidence and data accumulated over the centuries by astronomers working with the older, Ptolemaic model… Only by first ignoring all the data or reconceptualising it could Copernicus reject the geocentric model and infer a radical new structure to the solar system,” Larson writes. He adds that big data would have been unhelpful in solving the problem, given the underlying model was wrong.
The intangible essence that current AI models can’t muster is intellectual fluidity (of which Copernicus, also a polyglot, classics scholar, physician, diplomat and economist, had plenty). AI can vastly outstrip the human mind on some discrete, bounded classes of problems such as board games, but its inability to abstract and use common sense means that minor tweaks to its environment can utterly confound it.
“A few irrelevant letters added to the red area of a stop sign are easily ignored by humans, but when an image altered in this way was presented to one deep learning system, it classified it as a speed limit sign,” writes Larson. “And there are similar real-world examples, including autonomous navigation systems on self-driving cars that have misclassified a school bus as a snowplow, and a turning truck as an overpass.”
This is one reason there aren’t yet robots strolling around Manhattan, writes Larson. “A Manhattan robot would quickly fall over, cause a traffic jam by inadvisably venturing onto the street, bump into people, or worse. Manhattan isn’t Atari or Go—and it’s not a scaled-up version of it, either.”
Do we need artificial general intelligence?
Not everyone is convinced we need general intelligence to solve such problems. “Self-driving cars are mostly a matter of legal framework and societal acceptance. We can have useful self-driving cars rather soon if we redesign our urban environment to make it easy enough for them to navigate our roads, and absolve drivers and manufacturers of liability,” says Julian Togelius, associate professor at the department of computer science and engineering at NYU. He doesn’t personally advocate doing so, but says “this question has almost nothing to do with general intelligence”.
Others, like Larson, believe that AI will hit a roadblock without innovation in AI’s learning abilities. The question is, how do you push towards something you have no idea how to solve? The most convincing answers right now involve cultivating causal inference and a form of common sense in AI.
Turing Prize winner and computer scientist at UCLA, Judea Pearl, proposes the concept of the “ladder of causation,” which Larson writes, “steps up from associating data points (seeing and observing) to intervening in the world (doing), which requires knowledge of causes. Then it moves to counterfactual thinking like imagining, understanding, and asking: What if I had done something different?”
This overlaps with Larson’s definition of abduction, which he says “is a kind of leap or guess to an explanation on the basis of observation” that “presupposes a rich understanding of causation”. Most of the rungs of Pearl’s ladder are out of reach of today’s AI.
“We need to develop some way of systems understanding causation, not just correlation or statistics, but actually why things change in the world,” says Larson. But these causal models are in their infancy, and although some have shown promise in the medical domain, “they are not so far extensible to handle these big picture questions like developing really intelligent robots and chatbots and so on”.
Scientists and technologists vary in their optimism for eventually solving the puzzle of human-like AI. Some, such as Google’s director of engineering Ray Kurzweil, are relentlessly optimistic – so suggested by his 2005 book titled The Singularity is Near. (“It’s never clear what ‘near’ means,” says Larson.) Others are more tentative. Some say that it’s an inevitability, but no one knows exactly when it will happen. Yoshua Bengio, professor of computer science at the University of Montreal, and one of the pioneers of deep learning refuses to entertain a timeline at all.
Larson cites twentieth-century philosopher of science Karl Popper, who said that trying to predict a “radical conceptual innovation” before it’s happened is like asking someone in the Stone Age when they think the wheel will be invented. “We really don’t know. We actually don’t understand – there’s a mystery here. There’s no blueprint. What we do know is that we can only go so far with existing approaches,” says Larson. The bigger question is which classes of problem computers could ever hope to solve, and whether one day these will approximate human-like intelligence. “That was [always] an unknown for me, and still is in large part an unknown.”