Even if artificial intelligence and machine learning are often used interchangeably, they are not quite the same thing, even if deeply connected. Artificial intelligence is the broader concept of managing a machine, it is the ability of a computer to mimic human cognitive abilities, including pattern recognition and problem-solving. while machine learning is a specific application of artificial intelligence that lets machines learn information.
In the last decade, both concepts have become more widespread, especially when it comes to big data, analytics and other technological developments. artificial intelligence has gone from being an academic research tool that is used by organisations around the world to a mainstream topic. But what is artificial intelligence and how does it compare to machine learning?
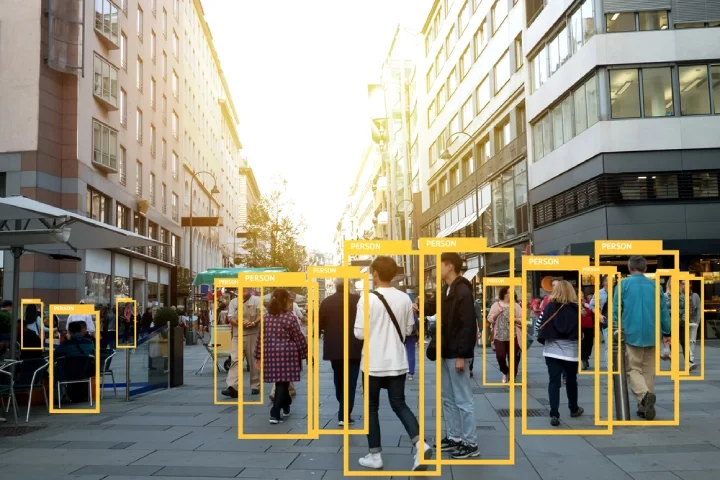
Google Cloud explains that “One helpful way to remember the difference between machine learning and artificial intelligence is to imagine them as umbrella categories. Artificial intelligence is the overarching term that covers a wide variety of specific approaches and algorithms. Machine learning sits under that umbrella, but so do other major subfields, such as deep learning, robotics, expert systems, and natural language processing.”
What is artificial intelligence?
Artificial intelligence means the ability of computers to perform cognitive functions that mimic the way humans think. This could be anything from a simple algorithm designed to stop and start a self-driving car to recognise patterns in the universe.
AI is increasingly found in all aspects of modern life. It can range from the relatively mundane such as the recommendation of other products on Amazon, through to the more sinister such as emotion tracking using facial recognition, a practice widely criticised for its lack of scientific basis.
The concept of machine intelligence dates to antiquity and has been featured in philosophy and storytelling for centuries. But the exploration of mathematical logic, which led to artificial intelligence, began to emerge with the work of British mathematician Alan Turing.
On the other hand, AI focuses on aspects of human cognition including learning, reasoning and self-correction and uses those abilities in a range of scenarios, from automating existing processes, making new processes possible and finding information that would take a human too long or requiring too many people to be cost-effective.
Work began on the development of artificial intelligence systems in the 1940s, but the technology didn’t enter mainstream use until the mid-1990s when the focus shifted from creating artificial general intelligence, which can theoretically solve any problem, to more narrow AI solutions that are designed for specific problems.
Mark Beccue, the principal analyst for technology research company Omdia, said AI has now entered the mainstream. It has reached the “early majority” stage of its evolution, he says, where enterprise companies are beginning to scale up projects and roll out AI solutions for widespread adoption.
What is machine learning?
Where artificial intelligence is the mimicking of human cognition, machine learning is the analysis of a significant amount of historical data to enable a computer to make predictions based on past events – it is the process a machine uses to learn and is a form of artificial intelligence.
According to IBM, machine learning “focuses on the use of data and algorithms to imitate the way that humans learn, gradually improving its accuracy.” The phrase was coined by IBM engineer Arthur Samuel in 1959 in relation to training a computer to play checkers.
“Through the use of statistical methods, algorithms are trained to make classifications or predictions, uncovering key insights within data mining projects,” IBM wrote in an article on the technology. “These insights subsequently drive decision-making within applications and businesses, ideally impacting key growth metrics.”
Machine learning uses computing technology to analyse vast amounts of information, in a way that a human brain would find possible, in order to make predictions. Data scientists train machine learning models using large datasets. They then optimise those models so they perform their function – whether that is spotting patterns in a certain kind of image, or making predictions from real-time data – as efficiently as possible.
Machine learning models have many applications in science, such make predictions based on past medical data or pictures of distant galaxies, as well as in industry. Financial services companies use machine learning to predict potential market outcomes based on past data, or it can be used in law enforcement to spot trends in behaviour.
The term is sometimes used interchangeably with the phrase “deep learning“, although there are differences between the two. Deep learning is a sub-field of machine learning where there is less input from humans, with much of the process automated. Traditional machine learning involves human experts labelling and determining the set of features and data inputs being analysed.
What is the difference between machine learning and artificial intelligence?
Overall, the biggest difference between artificial intelligence and machine learning is that while the former can imitate human intelligence, the latter cannot. Machine learning’s aim is to teach a machine how to perform a specific task and identify patterns.
Dr Ashley Spindler, an astrophysicist, told Tech Monitor that all machine learning is a form of AI, but not all AI is machine learning: “When we think about AI, it is a really broad concept. We are referring to anything that is a computer program that mimics human behaviour. It is deliberately a broad definition. It is anything that replicates the way human beings think and act.
“When we talk about machine learning, it is a very specific type of artificial intelligence. It is about taking algorithms that learn from historical data – such as facial recognition. They learn from images and they learn to map to find a property or label. They aren’t pre-programmed behaviours. The learning part is the most important aspect. They are mathematical models that are built on historical data.”
Dr Spindler uses machine learning to dig into astronomy data. “I take machine learning models and create predictions. We compare the results that come out of the model with stuff done by human experts and citizen science to produce a picture of the universe.”
Some AI tools work in different ways. Some have pre-programmed algorithms that haven’t been trained using data. “It is still artificial intelligence in that it mimics human behaviours, but it isn’t something gained through historical data, so is not machine learning,” says Dr Spindler.