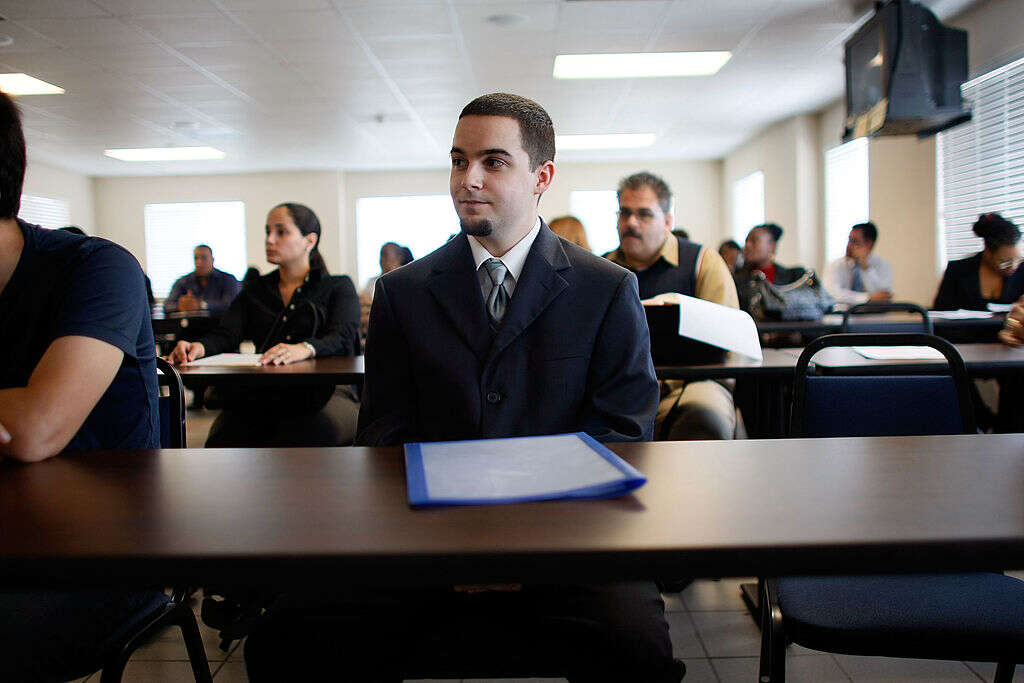
From discriminatory recruitment systems to racist chat bots, the ethical pitfalls of artificial intelligence are well documented. With the spectre of AI regulation looming, businesses are racing to understand the moral implications of algorithms.
Rising to meet this need is the nascent AI auditing industry. But the lack of a universal AI ethics framework has led to haphazard approaches – and fears that audits might not just fail to catch bias, but legitimise harmful technologies.

“In 2018, there was an explosion in interest in digital ethics as a result of the Cambridge Analytica scandal,” says Emre Kazim, researcher at UCL and co-founder of auditing and assurance start-up, Holistic AI. “At that point, engineers became more interested in hiring people from different disciplines – sociology, anthropology, law. I did my PhD in philosophy and started to work with engineers who were actually building these systems.”
Kazim says that a bifurcated approach to AI auditing has evolved. At one extreme, there is light-touch consultancy, where companies seek relatively high-level guidance, an ethics strategy or reputational boost. At the other end, there is a forensic audit, where an auditor will investigate a company’s data and algorithms. “Sometimes we go in and give a presentation, and say, ‘maybe think about these problems’ and ‘here is good practice’,” says Kazim. “In other contexts, we can go in and do a deep dive.”
Big and medium-sized companies will often solicit Holistic AI’s opinion before purchasing a new AI system, he explains. “We’ve worked with some companies where they’ve asked us to do due diligence on a system that they’re procuring – is it actually doing what it claims to do? How does it stand up to standard fairness metrics, how robust is it, etc.”
We’ve worked with some companies where they’ve asked us to do due diligence on a system that they’re procuring – is it actually doing what it claims to do?
Emre Kazim, Holistic AI
Other AI audit start-ups include Parity AI, Saidot, Clearbox and Cognitive Scale. Meanwhile, big consultancies including PwC and Deloitte are positioning themselves as dominant players. This is prompting the smaller players to differentiate themselves with specialist knowledge in areas such as algorithmic privacy and algorithmic robustness, says Kazim.
Some explicitly focus on ‘de-biasing’, “because bias is such a hot topic”, he explains. He says there’s also a great number of companies that work in the space of “explainability” – ensuring a company can explain why an AI system made the decision it did. Compared to the big four, these start-ups’ “edge would come from this kind of specialised expertise”, says Kazim.
AI auditing: what is ethical?
The diversity of approaches on offer from this nascent industry reflects the fact that there is little agreement on what ‘ethical’ should mean in the context of AI. “There is no consensus on what an audit means,” says Mona Sloane, a senior research scientist at the NYU Centre for Responsible AI and a fellow at the NYU Institute for Public Knowledge. “We don’t even know what ‘bias’ means or what ‘harm’ means, so that is a real concern.”
Sloane’s primary worry is that AI auditing is being used to whitewash companies’ reputations and legitimise harmful technologies. She’s seen “how audits can legitimise eugenicist technologies – technologies with politics that evolve around truly harmful ideologies and contribute to normalising these kinds of technologies, and distract from looking at questions such as, what are the underlying politics? What are the underlying assumptions?”
A recent flashpoint in the AI auditing debate was the furore over HireVue AI. The employment company uses AI to analyse job candidates’ facial features and movements during job interviews. This raises the spectre of physiognomy, which suggests character can be judged based on facial characteristics, and of phrenology, which ties the shape of the skull to mental faculties. Both pseudosciences have deep links to racist and eugenicist ideologies.
At the beginning of this year, HireVue announced the results of an algorithmic audit carried out by O’Neil Risk Consulting and Algorithmic Auditing (ORCAA), which HireVue presented as exonerating its tools from bias. However, AI researchers such as Brookings Institution fellow in governance studies, Alex Engler, said that the company’s summary of ORCAA’s assessment was misleading because the auditors had only been instructed to look at a very narrow use case of HireVue’s technology.
“In mischaracterising the audit, HireVue reveals the shaky foundations of the new algorithmic auditing industry,” Engler wrote in ‘Fast Company‘.
Operationalising AI ethics principles
The AI auditing industry would greatly benefit from the coalescence of formal and standard principles of AI ethics. “[Social scientists and technologists] have been working towards that for a long while,” says Sloane. “If you look at all the work that comes out of the fairness, accountability and transparency space… it’s a well-known problem.”
But developing these principles is complicated by the fact that technologists and social scientists understand the problem very differently, she adds. “Bias means a very specific thing to technologists and data scientists and machine learning experts – it’s a quantifiable phenomenon,” Sloane explains. “Statistical bias is something that can be addressed in certain ways; societal bias is much more tricky and much more important because that is not something that we can fix technologically.”
Statistical bias is something that can be addressed in certain ways; societal bias is much more tricky and much more important.
Mona Sloane, NYU Centre for Responsible AI
Work by researchers at the Oxford Internet Institute and Alan Turing Institute has examined this mismatch in detail. They found that technical work on AI ethics rarely lines up with legal and philosophical notions of ethics. “We found a fairly significant gap between the majority of the work that was out there on the technical side and how the law is actually applied,” said Brent Mittelstadt, one of the researchers.
In fact, the majority of metrics for defining fairness in machine learning clash with EU law, they argue. These metrics are often ‘bias preserving’ because they assume the current state of society to be a neutral starting point from which to measure inequality. “Obviously this is a problem if we want to use machine learning and AI not simply to uphold the status quo, but to actively make society fairer by rectifying existing social, economic, and other inequalities,” tweeted Mittelstadt.
The researchers instead advocate the use of ‘bias transforming’ metrics that better match the aims of non-discrimination law. They have proposed a new metric of algorithmic fairness, conditional demographic disparity (CDD), that is informed by legal notions of fairness. This metric has been incorporated into bias and explainability software offered by Amazon Web Services.
Kazim says that the development of a framework that ensures that algorithmic systems are legal is a “really active debate at the moment”. Holistic AI’s co-founders favour the idea of an AI Impact Assessment, analogous to a Data Protection Impact Assessment, that allows companies to systematically assess their AI ethics risks. At present, there are no agreed-upon standards of what such an assessment should entail, but Kazim proposes five dimensions: governance, privacy, robustness, explainability, and fairness.
Government oversight
In addition to standardised rules, auditors cite the importance of government oversight. “What would be great for us auditors would be clear, objective standards, set by a regulatory body, or a sector-specific body, like the ICO, or a sector-specific body, like the FCA, CQC or MHRA,” says Kazim. “Then we could say whether a system performed according to the standards, or didn’t perform according to the standards – just like we have for GDPR and Data Impact Assessments.”
Government oversight could ensure that audits are conducted appropriately. Right now, companies have no incentive to undertake or publicise audits, far less disclose that they use discriminatory algorithms. For example, Amazon quietly ditched a sexist hiring algorithm, which was only brought to light by a journalist years later.
This oversight is in the works. New York City Council is mulling legislation that would require companies that sell AI hiring tools to perform annual audits to check the technology isn’t discriminatory. In the US, President Biden’s administration has shown far more interest than Trump’s in using available regulatory tools to enforce existing anti-discrimination laws on algorithms.
In the UK, the ICO published a draft algorithmic auditing framework in 2019, and the Competitions and Market Authority is looking into algorithmic regulation too. The EU published its AI White Paper in February 2020, and will publish new AI regulation this month.
But legislation, if imprecise, won’t solve the issues dogging AI auditing. Sloane points out that the New York bill mentions “bias audits” without specifying what this means. “In the absence of a definition, or minimum requirements, or parameters, what people currently do as an audit sets the precedent and that’s what regulators look to – and that’s a very negative dangerous feedback loop.” Rights activists have aired similar concerns that the imposition of a lax AI audit could permit discriminatory software to get certified as having passed a fairness audit.
This can only be solved by marrying up the technical with the legal and societal problems. “You can fix bias as much as you want in the system; it’s not necessarily going to change the actual social situation,” says Sloane. “There’s a ton of work on that, but it needs to be integrated more into the policy space.”