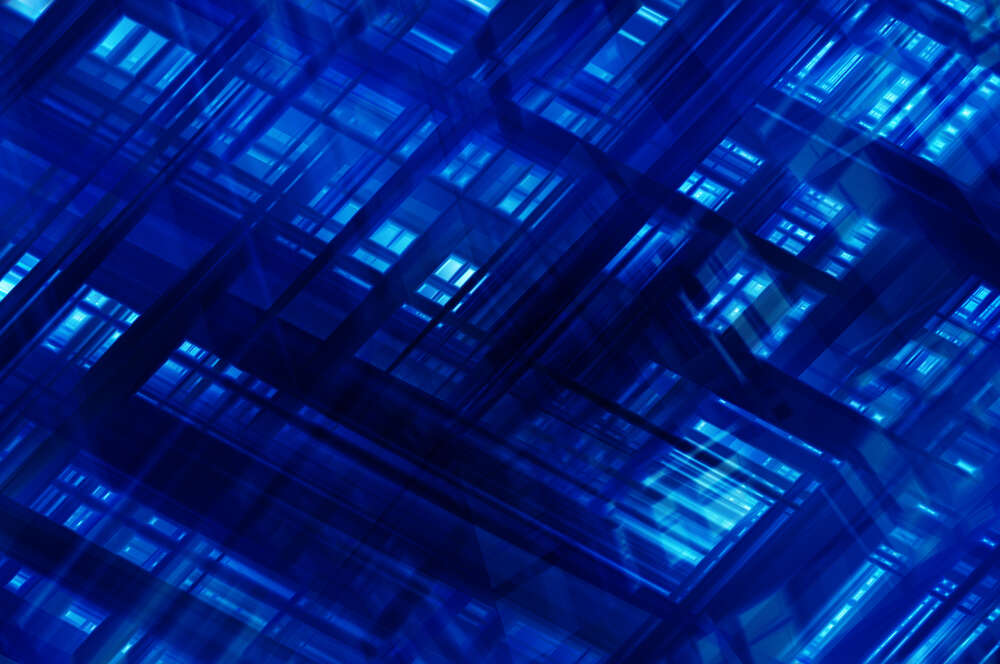
Banks seem to hate when their customers go on holiday. Somewhere in the world, Joe Bloggs has gambled that he won’t need to bring cash with him to sunny Sweden, since the Swedes seem to accept card payments more or less everywhere. As soon as he taps his plastic to the reader, however, there’s still a small chance that his bank decides to block the transaction. After all, say the algorithms, what evidence is there in the corporate records that Mr Bloggs is ever likely to pay for his kladkakka in Stockholm?
Billions of these types of decisions are made every day by machine learning (ML) algorithms in banks. Referred to in computer science as your average classification problem, these models have to decide whether a transaction is in keeping or not with the normal purchasing behaviour of the customer. For classical machine learning algorithms, that problem is mostly solved by obtaining a 360-degree view of the consumer by way of their payment history and other interactions with the bank – a computationally intensive, and imperfect, process.
An alternative could lie in the use of a quantum machine learning algorithm, explains IBM distinguished engineer and Royal Academy of Engineering fellow Richard Hopkins. What takes time and resources, explains Hopkins, is training your average classical ML model to recognise and weigh up all the different features of a transaction that indicate if it’s suspicious or not. Quantum ML models, by contrast, harness the superposition of qubits to deal with many features simultaneously. As such, says Hopkins, “we have the ability – we think – to come up with answers to very difficult classification problems faster. And, we’re seeing early signs of quantum combined with classical algorithms being more accurate than state-of-the-art classical algorithms alone.”
Hopkins believes that, while the field of quantum machine learning remains in its experimental stages, it may take as little as five years before we see quantum machine learning algorithms with applications in everything from fraud detection to drug discovery and computer vision. Not everyone in the field agrees. Dr Maria Schuld, a researcher at quantum start-up Xanadu and the co-author of Machine Learning with Quantum Computers, believes that quantum machine learning certainly has enormous potential in the long run – but that, right now, practical use cases are further away than they seem.
“We’re scientists, but we’re basically trying to make a business case for our science,” says Schuld. “We’re not doing this because we know this will work… we’re doing this because we hope something comes up.”
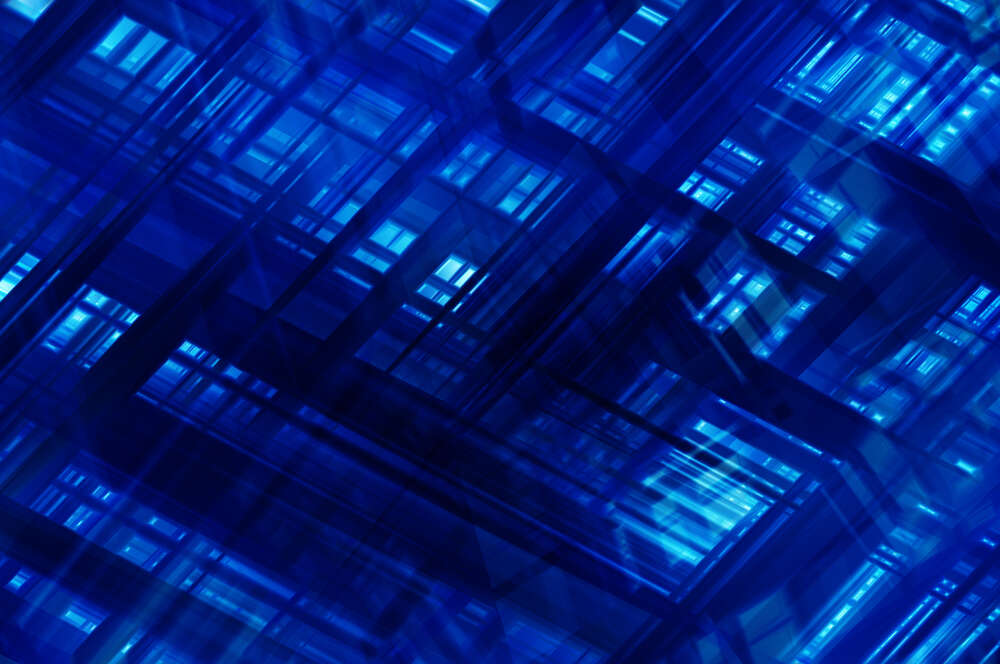
Quantum machine learning experimentation
Quantum ML is a relatively new field. While studies on the topic have been published since at least the mid-1990s, it has only really begun to capture the scientific community’s imagination in the past five or six years. Boiled down, says Schuld, quantum ML is simply the use of a quantum computer to do something, anything, in machine learning. Within that, there are two broad areas of research, the first being the use of quantum computers to speed up classical machine learning algorithms, like a Gibbs sampler.
“The other part is the idea of using a quantum computer as a model,” says Schuld, specifically in the use of quantum circuits themselves as the basis for training models in a similar way to a conventional neural network. “People have pushed it so far,” she says of this particular research area. “It’s absolutely insane.” Even so, says Schuld, the field as a whole remains highly experimental. While there are certainly cases where quantum machine algorithms can demonstrate a ‘quantum advantage’ over their classical counterparts, she explains, there’s still a while to go before we can begin to realistically imagine them being used in real-world applications.
This is belied by the positivity about quantum ML across multiple studies, usually published on the premise that their particular experiment has achieved quantum advantage for a single, narrow use case. This, wrote Schuld in a review article last year, ‘is interesting from an academic point of view, but does not say much about possible quantum applications.’ Many of the problems being solved in such studies, after all, are painstakingly coded to work on quantum computers. In other words, they’re only good at solving a very specific problem, in a very specific way, on a very specific and fragile machine.
This is much less of a problem for classical machine learning techniques, many of which have the bonus of being generalizable to many more problems. We’re not yet at the point in utilising quantum circuits as the basis for new machine learning models, argues Schuld, where the field can definitively say it has specific models equipped, she adds, with “Gaussian processes that are known to work in practice and whose mathematical principles are well motivated.”
Instead, quantum ML researchers continue to have a hard time fitting one method onto a general family of tasks. It is not a field, argues Schuld, where there’s been one huge breakthrough and scientists are scrambling to assess its significance on a multitude of application areas. “It’s the other way around,” she says. “We’re searching, searching, searching.”
Practical magic
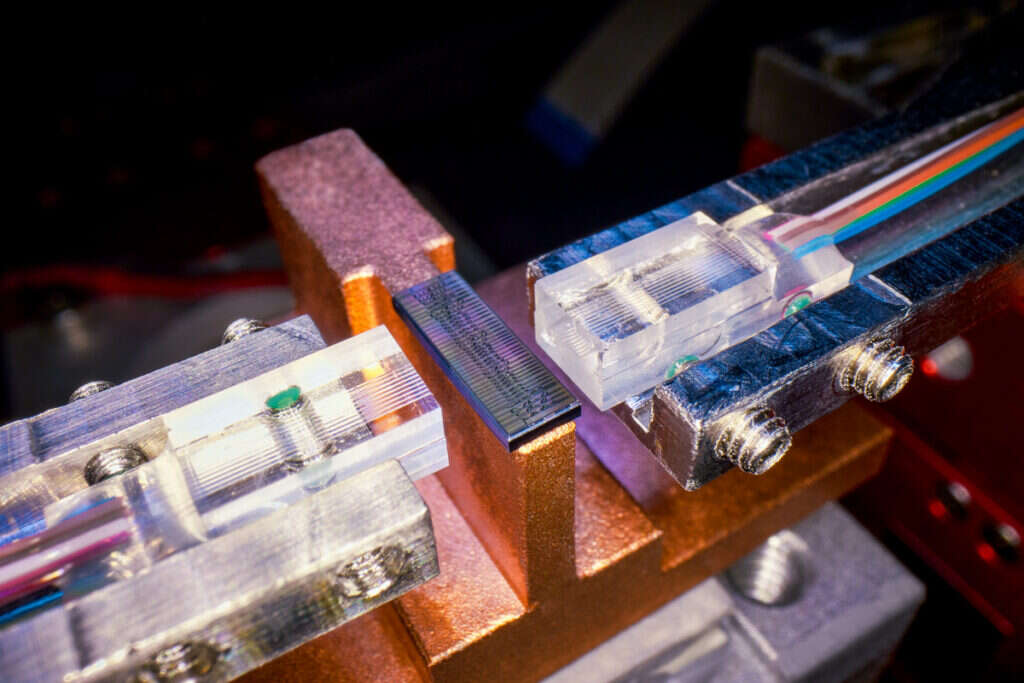
Hopkins does concede that the field still lacks generalizable templates for mapping a problem onto an algorithm and generating a solution, a problem also shared with classical ML. “There is still some ‘magic,’ if you like, some experimentation, around how you map the problem to the input parameters,” he says. But, “once you’ve got that working, then exploiting the technology becomes very easy”.
What’s more, he argues, recent studies demonstrating quantum advantage in machine learning problems like classification are nothing to be sniffed at. Take the detection of credit card fraud, an ongoing research area for IBM, explains Hopkins. Quantum computers should allow the user to make “better, more accurate decisions based on a higher dimensional dataset,” he says. “We’ve shown that in theory, we’ve shown that in the lab, and we’re getting closer and closer to showing that in reality.”
But how ready are quantum ML models for primetime? Not very, says Schuld, who maintains that we won’t know whether we’ll have quantum machine learning algorithms capable of solving more than a handful of practical problems until the underlying hardware improves. “I think this is, by now, uncontested,” she says. Most people Schuld talks to, she adds, have “come to the conclusion that unless we have got these proper machines that don’t have errors,” there’s a limit as to what can be realistically accomplished using quantum machine learning. Needless to say, she adds, that’s “a bit sad in terms of practical applications”.
Hopkins disagrees. “We are trying to apply [quantum ML] already,” he says, joining up with multiple clients to explore practical applications for such methods on a timescale of years and not decades, as some have ventured. And the hardware is getting better and better, he argues, citing IBM’s roadmap for scaling its quantum technology using processors with ever-larger qubit counts (Intel and Google have their own approaches.) While it’s not likely we’ll see a quantum computer capable of training a ChatGPT-type model any time soon – “You’re not going to fit that on a quantum computer with only 433 qubits,” says Hopkins – sufficient progress is being made each year to expand the possible number of quantum ML experiments that could be run.
He also predicts that we will see quantum ML models become more generalisable. Schuld, too, is hopeful that the quantum ML field will directly benefit from recent and forthcoming advances on the hardware side. It’ll be at this point, she predicts, when researchers can begin testing quantum ML models on realistic problem sizes, and when we’re likely to see what she describes as a ‘smoking gun’ revealing a set of overarching principles in general quantum ML – one that reveals just how much we do and don’t know about the mysteries of applying these algorithms to complex, real-world problems.
“I think we’re building the foundations at the moment, so that this spark can happen, so [that] it’s not just aimless search,” says Schuld. “But that’s what has to happen.”