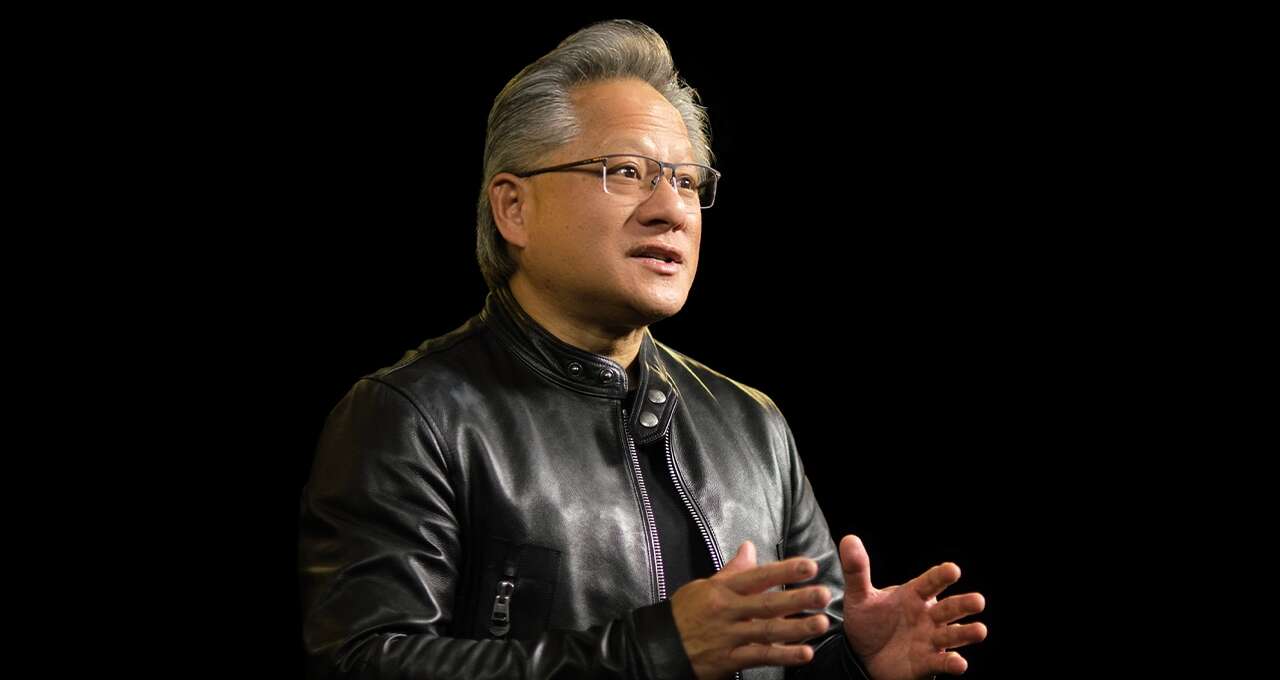
Jensen Huang getting carried away about an emerging technology is nothing new. This time last year, the charismatic and excitable co-founder and CEO of chip design giant Nvidia was telling anyone who’d listen about the potential of the metaverse (or the Omniverse, as Nvidia’s marketing department prefers to call it). Since then, the metaverse bubble has suffered a slow puncture, and Huang is back to evangelising about one of his favourite topics: artificial intelligence.
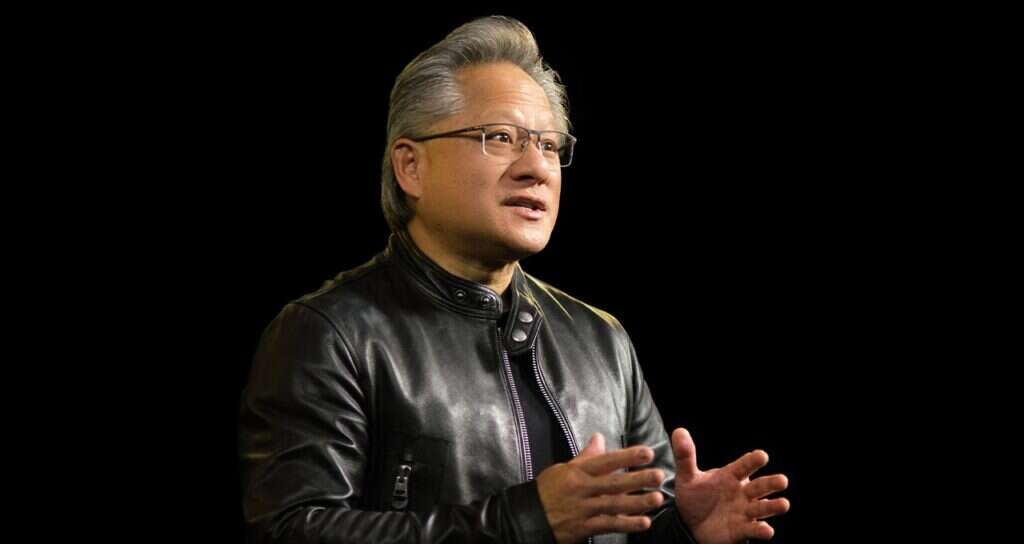
Describing the growth in power of generative AI systems like GPT-4 – the model that powers OpenAI’s tools such as ChatGPT – as a “new era of computing”, Huang told investors on his company’s most recent earnings call that AI was at an “inflection point”, stating that businesses have “an urgency to develop and deploy new AI strategies”.
However, Huang added that he believes many companies face “an insurmountable obstacle” in getting access to the resources and skills needed to make AI work, which is why, he says, Nvidia is getting into the services business.
Nvidia AI Cloud will allow customers to buy AI services directly from Nvidia, deploy pre-trained generative AI models and utilise one of the company’s DGX AI supercomputers. Full details are expected to be released at the company’s GTC developer conference, opening later today. “With our new business model, customers can engage Nvidia’s full scale of AI computing across the private and any public cloud,” Huang said.
Nvidia’s long-standing bet on the potential of AI means it has been one of the companies to benefit most from the ChatGPT boom, with its powerful graphical processing units (GPUs) being used to train systems like GPT-4, as well to run AI workloads on the servers of the hyperscale cloud providers.
But with some of its major customers working on AI chips of their own, could the data centre dollars be set to dry up? And might AI-as-a-service be a lucrative new revenue stream, as it seeks to keep its edge in the new era of computing?
Nvidia’s bet on AI pays off
Founded in 1993 by Huang, Chris Malachowsky and Curtis Priem, Nvidia has established itself as the world’s premier provider of GPUs, a type of chip which sits on top of the CPU to increase a system’s performance.
Initially focused on servicing the gaming industry, where Nvidia is still a major player, about ten years ago the company identified another potentially profitable use case for its technology, and started to develop GPUs to be used for AI workloads.
“We saw early on, about a decade or so ago, that this way of doing software could change everything,” Huang said in an interview with CNBC earlier this year. “And we changed the company from the bottom all the way to the top and sideways. Every chip that we made was focused on artificial intelligence.”
This proved to be one of Huang’s better decisions. Nvidia’s top performing AI GPU, the A100, now sells for around $10,000, and systems that train large language AI models use thousands of the chips at a time. The AI supercomputer built by Microsoft for OpenAI to use to train its models, for example, features 10,000 of Nvidia’s GPUs. Such deals helped Nvidia’s data centre revenue exceed those of the gaming division for the first time in 2020, with enterprise-related revenue now accounting for the bulk of the company’s income.
Overall, though, Nvidia's revenue has stalled, with the company being hit hard by the global economic slowdown. Despite this, the last quarter saw it perform slightly better than market expectations, based largely on sales of AI chips. It recently launched the successor to the A100, the H100, which it hopes will help it continue this growth.
With the number of LLMs and related tools on the market growing by the day, Nvidia is unlikely to be short of takers for its GPUs anytime soon, says Alan Priestley, vice president analyst at Gartner. "The demand for high end performance is driving their business at the moment," he says. "We're still on the ramp of this generative AI trend and nobody really knows where it's going to end.
"It takes stupidly large amounts of resource to train these things, and that's where Nvidia is making its money. Its product is good high performance, general purpose compute, which is capable of doing a lot of different things at the same time - and that's what we need right now."
Its medium to long-term prospects are less clear, Priestley says. "Once we understand what we want to do with AI, and what the applications of it are, you don't need general purpose any longer," he explains. That is when you need application-specific chips. "Google has already done that with GPUs, and we're going to see that happen more and more," Priestley adds.
Here Nvidia has to contend with some of tech's biggest names wanting a slice of the AI hardware action. In October, Google – an Nvidia customer – released its latest Tensor processing units, which are optimised to train and run AI and machine learning models. Amazon's market leading cloud platform, AWS, also works with Nvidia to offer GPUs to its users, but also has its own in-house silicon. AWS pairs its Graviton range of CPUs with its AI-optimised Tranium chips for deep learning workloads, and its recent announcement of a partnership with AI start-up Hugging Face, seen by many as an attempt to imitate Microsoft's close relationship with OpenAI, trumpeted the fact that users could build LLMs using Tranium.
How serious is Nvidia about services?
Faced with this growing competition, will Nvidia look to turn the tables and present a services offering to rival the hyperscalers? Tracy Woo thinks it's a possibility. The Forrester senior analyst says it's unlikely we'll see Nvidia launch a full-blown cloud service ("the start-up costs to compete with the hyperscalers would be $1bn at least"), but she does feel there could be mileage in an AI-specific offering.
"No one is going to go in and try to compete with the hyperscalers across the board," Woo says. "But you will have people trying to go and chip away at certain segments of the market because they can't necessarily dominate everything.
"What Nvidia is trying to do with its cloud is figure out whether there are there special use cases where users only want the specific compute capabilities, rather than a more general offering. That might be specialised infrastructure to power machine learning or AI capabilities."
Alongside this, Woo says Nvidia's partnership with Microsoft, the only hyperscaler not to have launched its own AI chip yet, is significant. The companies announced a "multi-year collaboration" in November which will see Microsoft build a powerful AI supercomputer on its Azure cloud platform using Nvidia GPUs and software.
For Microsoft, Woo says keeping Nvidia close could be a way to keep pace with its Amazon rival. "AWS doesn't want to be beholden to anyone else," she says. "While it's still using Intel for the bulk of its chips, it's going to be looking to develop and make those itself. Microsoft is at an interesting point in its decision making, which is: 'do we continue to build out and create infrastructure services that can match AWS toe-to-toe?'"
Given that the cost of that is likely to be significant, Woo says a long-term collaboration with Nvidia makes sense for both parties. "This could be potentially a very fruitful partnership where they can both benefit," she says. "Nvidia gets to insert itself into the narrative of data centre, and Microsoft doesn't necessarily need to compete with AWS by building its own infrastructure. It can lean on one of the largest players in AI infrastructure capabilities instead."
Nvidia offers services in other areas such as the metaverse, where its cloud-based Omniverse platform enables businesses to create 3D environments. It has worked with BMW, for example, to build a digital twin of a factory where scenario planning and materials testing can be carried out virtually.
But Gartner's Priestley believes most of the company's forays into services are likely to remain in partnerships like the Microsoft tie-up, rather than as stand-alone offerings.
"Nvidia is standing up Omniverse services as a product at the moment, but I'm not sure that's its long-term future," he says. "They're more interested in selling the hardware to run it. I think [the services] are more targeted for developers and the specialised market to get the thing going, but eventually that service is more likely to be stood up by Adobe or Dassault or one of the other companies in the design space.
"Nvidia's model has been to supply the product so that someone else can supply the services on top of that, and the bulk of its revenue is still coming from selling products."
Beyond AI: what does the future hold for Nvidia?
When Huang delivers his GTC keynote this week clad, no doubt, in his trademark leather jacket, he may have more than AI on his mind. Nvidia has been caught up in the tech trade war between the US and China, which has seen Washington impose sanctions on semiconductor companies wanting to sell to Chinese clients.
Current export rules prevent Nvidia marketing the A100 to the Chinese market, from which it derived $5.7bn in revenue out of a total of $26.9bn last year. The firm has sought to get around this with a new datacentre GPU, the A800, which offers a chip-to-chip data transfer rate of 400 gigabytes per second, compared to the 600 gigabytes per second on the A100, sales of which are banned under the new regime.
As such, Priestley expects Nvidia to be able to weather the latest US sanctions on China with aplomb. He says that while the A100 is banned, this may lead to Chinese businesses buying a higher volume of less powerful chips to compensate. While the restrictions are significant, he adds, Nvidia are "selling a lot of high-value chips into the hyperscalers to train these large models, which could offset them. They said at one point they were going to take out a $400m contingency against loss of business in China, but we haven't heard anything about that in subsequent reporting, so I don't think the sanctions so far have had much impact.
"I suspect there's been a reconfiguration [of Nvidia's China business], and if that means Chinese clients need to buy 1,500 GPUs to get the desired result, rather than 1,000, they'll just buy them."
In terms of new opportunities, Woo expects Nvidia to join the other tech giants in vying for share of the nascent edge computing market.
"Jensen has talked in the past about how Nvidia expects the edge to be a place it can provide market value and differentiation," she says. "It's not like the cloud market where everything is established and we know who the main players are.
"Everyone is trying to converge at the edge and solve the 'last mile' problem that cloud providers have, which is getting physically closer to their end users and providing them with local compute and processing. This is potentially an area where Nvidia can reinvent itself and provide new value for new markets."
Read more: Nvidia launches generative AI and supercomputing cloud services as part of ‘new business model’
.