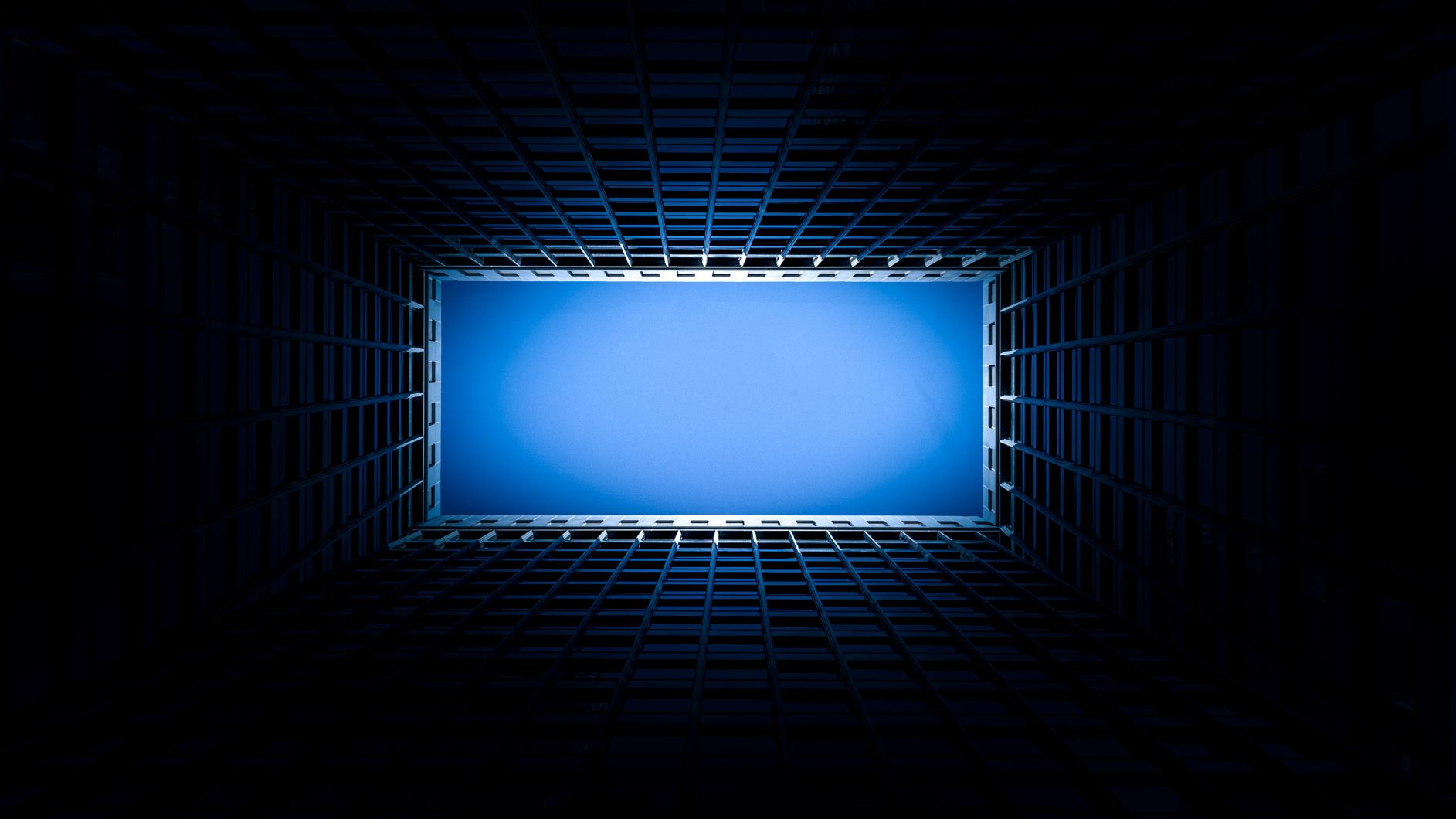
Today, neural networks dominate the landscape of AI and AIOps, the question I pose is whether this is justifiable and sustainable, writes Will Cappelli, CTO EMEA and Global VP of Product Strategy at Moogsoft. Let’s look at this commercially. Within the context of AIOps, neural networks have peaked in their ability to deliver effective and meaningful results. There are a number of limiting issues that relate directly to neural network algorithms, and it is my belief that these cannot be changed.
I would say that neural networks are faced with three big issues, and this ranges from single layer neural networks to multiple layer networks.
Lack of Actionable Insight
The first issue, and to a degree the most significant in terms of its application to AIOps, is that the results that neural networks generate — i.e. what they tell you — are derived from mathematics which are not understood and are largely unknown.
When you examine any kind of statistical algorithm there are always two dimensions to it – one is the result the algorithm generates and then there are the processes, which we call statistical inference, which provide the user with some idea of how accurate the result is, what it is based upon, and what needs to be modified to make the result more accurate. In essence, this highlights a trade-off between algorithm and inference in relation to statistics.
In the case of a neural network statistical algorithm, we simply don’t have as yet, and maybe never will have, a statistical inference component. Therefore, once you have the result there is no methodology to go back and understand how accurate the result is, or how the result has been derived. Even mathematicians can’t make sense of it.
It may be that future developments take place and we get a better grasp of what is going on which is possible, especially as it’s a very hot research area because of the commercial popularity of neural networks. But, for now, that insight is not available.
For AIOps this is a real killer, one of the biggest blockers to the aggressive deployment of AIOps technologies is a basic lack of trust in the results. It takes a long time for the business decision maker to become sufficiently conformable with the outcome of an AIOps algorithm. As long as this basic insight ability is lacking, it will limit the attractiveness of the use of neural networks on a broad scale. This is the most fundamental point in regard to the limitations of neural networks.
Square Peg in a Round Hole
Issue number two. Neural networks are currently being deployed everywhere; it’s become the hammer to which everything is a nail. There’s an awful lot of funding available and neural network technology is consequently applied to every conceivable problem. The truth of the matter though, is neural networks were originally developed to create hierarchies of classification – its raison d’être is about identifying classes of things and then identifying classes of classes of things and so on up a hierarchy. If you’re interested in classifying images for example, neural networks are perfect. If you are trying to classify different types of genes, again neural networks would be a good fit.
Admittedly with AIOps, there are some issues you could label classification problems, but the most central problem that tends to occur relates to whether or not one event has caused another event (diagnostic problems), or if an event occurs being able to anticipate other events taking place in the future.
When you look at these types of problems one of the first things you notice is that you’re trying to relate things which are dissimilar to one another. For example, maybe you’re interested in whether or not a given server is transgressing a usage threshold which has an impact on latency for an end user application. There’s no classification going on, you’re not saying that a server threshold transgression and user latency increase are in some sense the same thing. You’re trying to determine how these two very different occurrences relate.
In all honesty, neural networks are not suited to solving this type of problem. From an economic perspective, when you distort the underlying logic to try and solve a problem you have to spend more time developing the algorithm, your chances of error increase and you’re adding significant cost to the solution.
Too Slow for IT Ops Use Cases
Issue number three. Even if we assume neural networks were intelligible and suited to the task at hand, there is the issue that the neural network algorithm in order to work takes a significant amount of time to do the job. It’s an algorithm that needs to train for significant periods of time, in order to achieve its result. One of the things that is required in an IT Ops scenario is to deliver results as instantaneously as possible.
There’s no question that your methodology improves over time, but you don’t want to be in a situation where a significant amount of time has to transpire between initiating the workings of the algorithm and its delivery of results. In many IT Ops use cases you can’t tolerate response times of more than one minute – neural networks can take weeks before they deliver anything useful. The timescales are all wrong for extensive deployment of this type of technology.
What’s the Alternative?
There are alternate techniques which will discover patterns in data much more rapidly than neural networks. You can take large data sets and establish an equation or curve without evoking the machinery of neural networks. There is even a lot of classical statistical methodology which just by being automated can achieve the same results more rapidly. To generate pattern discovery there is no need to rely on neural networks.
However, pattern discovery is only one piece of the puzzle. There are algorithms which select the appropriate data to be analysed which you need to do before you find the patterns in the data. Then, once you’ve discovered the patterns, there is a third step of using automated logical inference to infer all of the various consequences that may be hidden in those patterns. The fourth step is using another set of algorithms to communicate to various agents. And then finally there are the algorithms which are associated with robotic automation which respond to what has been communicated.
AI and AIOps use a multidimensional approach. If you focus exclusively on neural networks you are collapsing the five dimensions, I’ve just described, to one. And then you’re collapsing that one dimension to one technique that has the three issues previously stated.
Conclusion
In regard to neural networks you have the issue with basic intractability, you have an issue with mismatch and finally you have an issue with inherent latency. All of which, prevent the widespread deployment at scale of neural networks in IT Ops management environments.
It is my conviction that even though there is a lot of investment currently being made in neural networks, both within enterprise and vendor communities, its bearing on AIOps and the real-time business community will ultimately be limited.