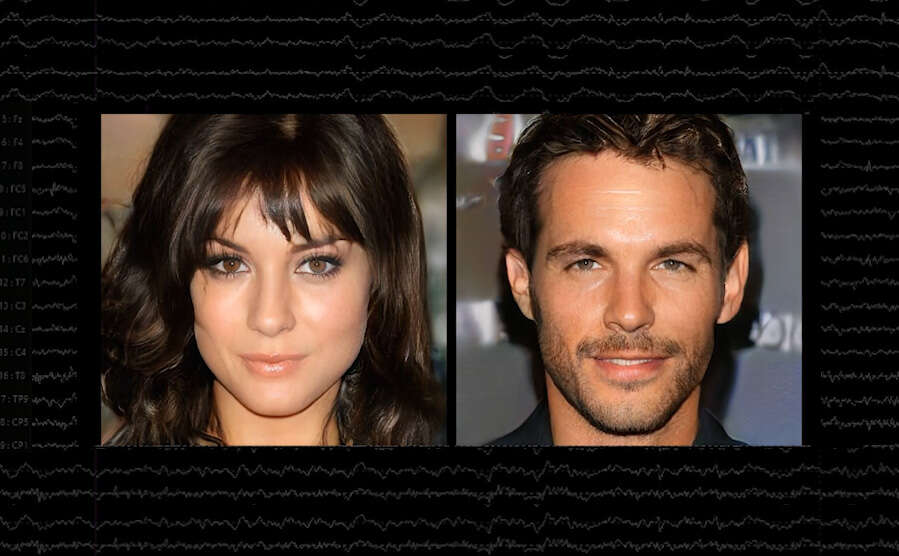
Beauty, so the saying goes, is in the eye of the beholder. It is a rare example of a proverb supported by scientific evidence. While abundant research has been conducted into the broader parameters of what society deems outwardly ‘beautiful’ – facial symmetry, for example, has broad cross-cultural appeal – it has been notably harder to shed light on what accounts for individual attraction. While we can certainly guess at the importance of the variables in the equation, such as personal upbringing or societal mores, our knowledge of how the brain chooses to parse that data to produce an emotional reaction remains dim at best.
Recent research from the University of Helsinki, however, promises to shed some light on this intensely subjective experience. By training a type of AI model known as a general adversarial network (GAN) to spot when a human subject felt attraction to an image from an electroencephalogram (EEG), a team led by cognitive neuroscientist Michiel Spapé successfully generated new pictures of faces that the test subjects found subjectively attractive – “a bit like ‘Weird Science,’ if you know this kind of awful, 80s movie,” he says.

The team’s work builds on previous research using computer vision, which attempted to predict attraction among individuals by varying certain known principles, such as facial morphology. These experiments, however, only provided more nuanced insights on widely held aesthetic preferences, and would inevitably dismiss individual variations in the results as noise.
By contrast, Spapé and his team found it more psychologically interesting to delve into the purely subjective by combining an AI with a brain-computer interface (BCI). Predominantly associated with research into overcoming paralysis or remotely controlling cursors on screens, their goal was to use a BCI “in a generative way,” using data from EEG scans to train their machine learning algorithms to recognise subconscious signs of attraction in test subjects and produce attractive images of faces tailored to their personal preferences.
Doing so required the use of a GAN, a type of AI composed of two neural nets: one designed to produce a novel image to a set of parameters, and the other to verify that the first filled the brief. Most famous for being used to produce new images from minimal input data, the GAN used by Spapé and his colleagues was first tasked with generating new pictures of human faces from a dataset of celebrities, before analysing the reaction of the test subjects to these novel images and then producing yet another, more ‘attractive’ set based on that neurological feedback.
It worked: in more than 80% of cases, test subjects rated the GAN-generated headshots as more attractive than the previous set of pictures. The experiment also succeeded in providing some additional evidence for how gender differences influence our sense of attraction. “The men basically seemed to find more or less the same stuff attractive to any other man,” says Spapé, while the female participants reacted better to a greater variety of headshots.
There are, of course, limitations to the study. The test subjects were all recruited around the Helsinki area, meaning that the results were subject to that community’s broader definitions of physical beauty. And while the GAN beat the odds in creating more attractive headshots from a dataset of celebrity faces, this also meant that that the inherent biases present in who society elevates to that status were reflected in the results. The paucity of persons of colour in the dataset, for example, was reflected in the low racial diversity of the headshots generated by the model.
Spapé and his colleagues are aware of these shortcomings, he says, and aim to expand their investigation into how the model works using larger datasets and wider and more diverse pools of test subjects.
From beauty AI to emotional interfaces
While the psychologist doesn’t believe that the experiment is likely to lead to EEG-aided dating apps, let alone an optimal definition of attraction for any individual, the experiment has nonetheless spurred new questions on how AI might be used to incorporate subjective experience into BCIs.
“Instead of having a machine that can move a cursor around on a screen, which is kind of the default BCI scenario, can we show, based on our tools, an emotional representation of what somebody is going through?” says Spapé.
The mechanism that his research has used to detect and visualise the subjective experience of beauty could be turned to fear, for example, or even our unconscious biases. And it could help people convey qualitatively complex information about their emotional states more quickly than mere verbal explanation – a boon, perhaps, for everyone from mental health professionals to those working in creative industries for clients who can’t quite make up their minds.
For now, however, the experiment has provided a rare opportunity to use the feedback from one brain to actively influence the course of another, decidedly artificial counterpart. “And if you get this kind of information, and feed it back into the GAN, you can get a little bit of humanity, to some extent, inside it,” says Spapé.