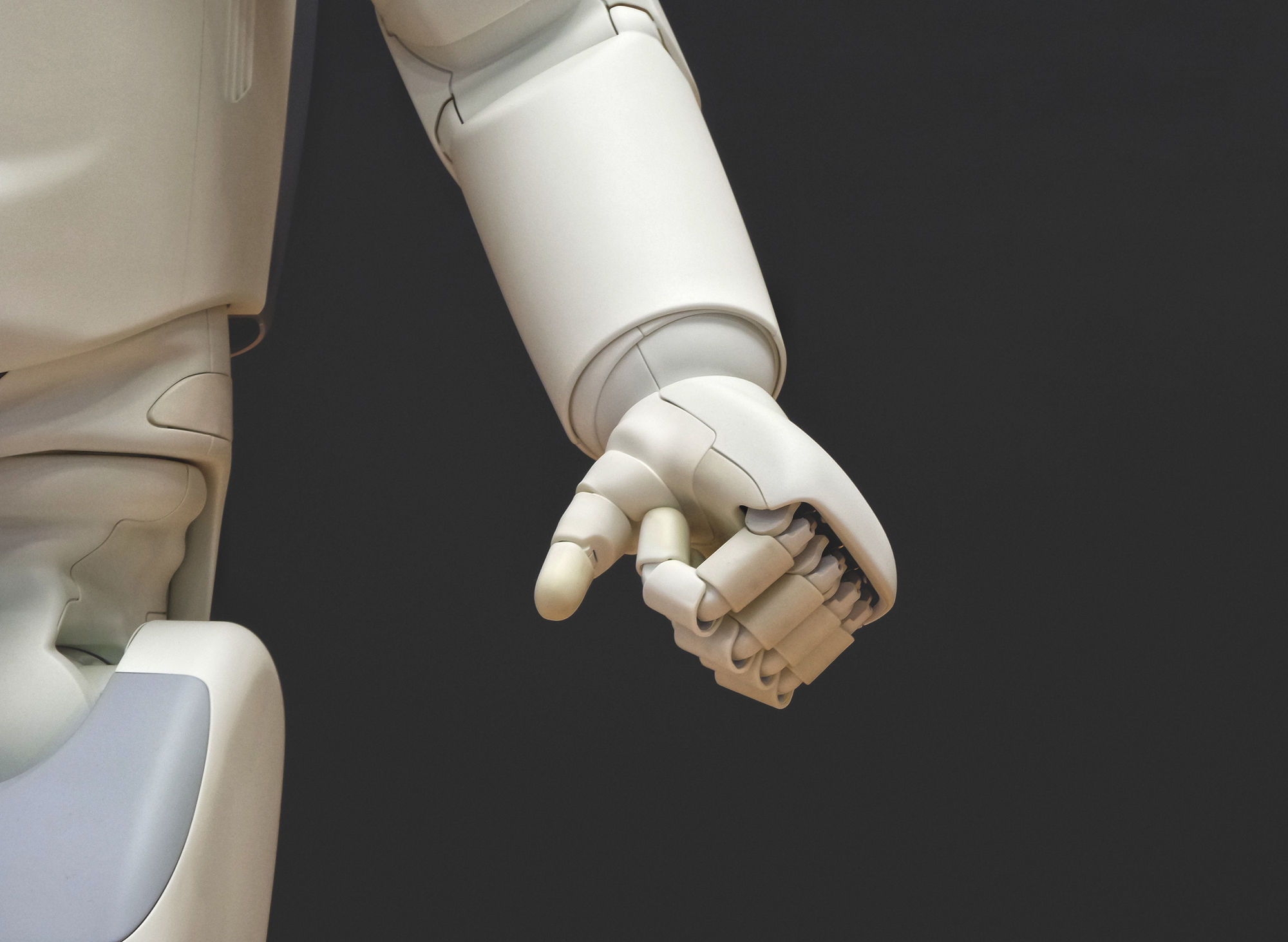
Researchers from MIT’s Computer Science and Artificial Intelligence Laboratory (CSAIL) have created a predictive AI that can create realistic tactile signals without actually touching an object.
The researchers at MIT used a web camera to recorded 200 everyday objects like household products, tools and fabrics.
They recorded these objects being touched by a tactile sensor called GelSight over 12,000 times. Those 12,000 video clips were then broken down into static frames which compile the 3 million image VisGel dataset used by the predictive touch AI.
The tactile sensor called GelSight presses a transparent synthetic rubber against the surface of an object, when it comes into contact a camera takes a photo of the now indented and deformed side of the slab of transparent synthetic rubber. This image is then analysed by an algorithm to give it values.
Yunzhu Li, CSAIL PhD student and lead author on the VisGel paper commented in an MIT researcher blog that: “By looking at the scene, our model can imagine the feeling of touching a flat surface or a sharp edge.”
“By blindly touching around, our model can predict the interaction with the environment purely from tactile feelings. Bringing these two senses together could empower the robot and reduce the data we might need for tasks involving manipulating and grasping objects.”
Robot-Object Interaction
With the VisGel dataset robotic arms used for picking up and moving objects will be able to judge the shape and amount of touch required to grasp an object. When the robot views an object it takes a frame of its video feed and then compares it to the dataset, thus given it a reference for touch and feel.
For instance if it was to view a knife it would be able to appropriately plan how to pick it up without causing a danger that it would drop it or damage its components by picking up the blade section first.
“This is the first method that can convincingly translate between visual and touch signals,” commented Andrew Owens, a postdoc at the University of California at Berkeley.
“Methods like this have the potential to be very useful for robotics, where you need to answer questions like ‘is this object hard or soft?’, or ‘if I lift this mug by its handle, how good will my grip be?’ This is a very challenging problem, since the signals are so different, and this model has demonstrated great capability.”