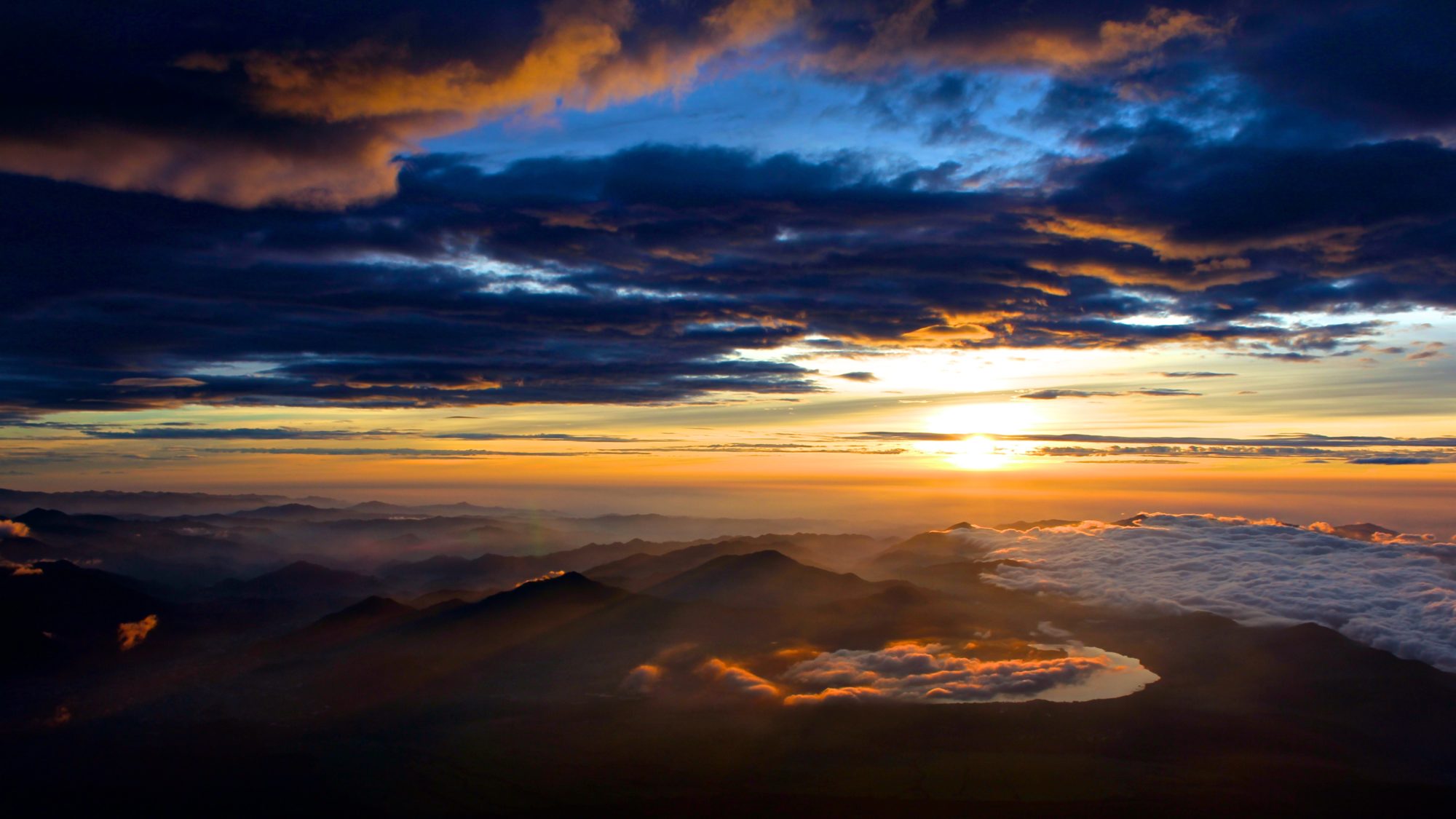
Google Cloud Platform (GCP) has added an additional five petabytes (5PB) of data storage for public datasets to its BigQuery enterprise data warehouse, which already hosts over 100 machine learning-ready public datasets.
The Google Cloud Public Datasets programme, launched in 2016, works with public data providers to store copies of high-value, high-demand public datasets in GCP to make them more accessible and discoverable.
It currently hosts some 3PB of data including Landsat data from the United States Geological Survey (USGS), along with Bitcoin blockchain transactions, GitHub Activity Data and Human Genome Variants.
The additional storage will be available for the next five years.
Shane Glass Program Manager at Google Cloud Public Dataset Program said in a blog: “We also continuing to curate and host datasets in BigQuery so users can leverage BigQuery Machine Learning to analyze data with machine learning using standard SQL queries… so that our users can JOIN their private data and the world’s public data with as little time and effort as possible.”
Public Datasets
Public datasets on the Google Cloud Platform provide a resource of contrasting datasets that are freely hosted and maintained. These datasets can be accessed and analysed using varying analytics software. Researchers can use open source software like Apache Spark or they can use Google Cloud Dataflow or BigQuery.
Google BigQuery is an enterprise data warehouse which allows people to conduct fast Structured Query Language queries on Google clouds infrastructure. Users can access BigQuery by using a web user interface or by access it through a command-line tool.
See Also: Google Cloud Announces Collaborations with Accenture and GitHub
The offering is fully managed, so companies do not have to setup any resources prior to using it such as virtual machines or disks.
BigQuery ML lets users utilises machine learning to create and execute learning models which can be used to analyse the large date sets held on site. Currently BigQuery ML supports two types of models; binary logistic regression and linear regression.
On Google Clouds blog they state that: “BigQuery ML democratizes the use of ML by empowering data analysts, the primary data warehouse users, to build and run models using existing business intelligence tools and spreadsheets. This enables business decision making through predictive analytics across the organization.”
Shane Glass added: “We are particularly focused on making available datasets that can support BigQuery’s new GIS capabilities like BigQuery Geo Viz.”