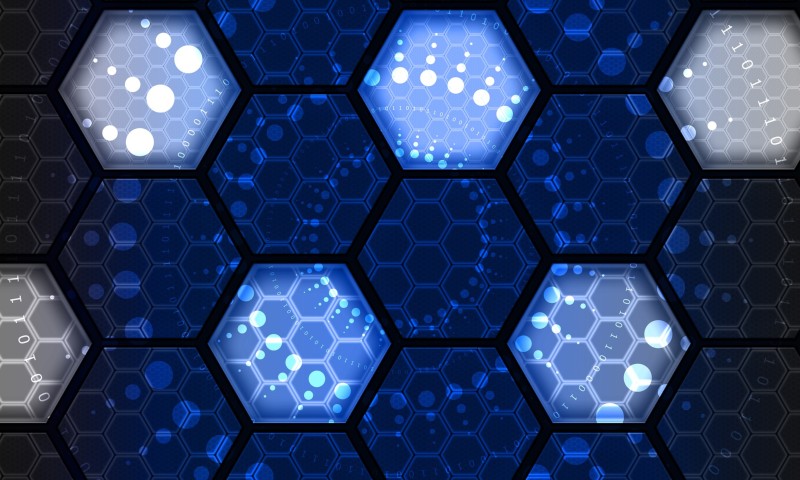
The pace of technology innovation seems to accelerate each day to meet the changing demands and needs of the average consumer. Customer’s expectation of what they need, when they need it, and how they need it, are moving at a phenomenal pace. We are at a time in our evolution where instant is everything.
This means when we’re asking for information, trying to execute a transaction or when we’re trying to decide, we need the data now! To meet that need, every business must ensure that its systems, processes, technologies are built to deliver on that expectation.
Interactions are becoming hyper personal – what you see on your screen correlates directly to your persona, to your behaviour, and to your expected or anticipated need. This mass-customised experience has to be delivered to millions of individuals.
That’s the challenge faced by every business today. The efficiency with which you can deal with data, the intelligence that you can apply to the data, and the speed with which you can represent, and predict what you can deliver to the end customer is the difference between winning and losing.
With so many organisations transforming their operational architecture towards adopting a cloud native approach for delivering fast-responding applications there are some key architectural considerations, which if ignored, will not allow data to flow at the speed required to maintain competitiveness. Each organisation needs to evaluate these key considerations to enable a successful transition.
Goodbye SOA, Hello Microservices
Many applications are still SOA-based and to meet today’s ‘speed of service’ the architectural mindset has changed, with microservices architecture gaining in popularity.
Instead of architecting monolithic applications, developers are able to achieve accelerated application delivery by creating many independent ‘services’ that work together in concert to deliver the customer experience. A microservice architecture delivers greater agility in application development and simpler codebases.
Once developed, updates and the scaling of individual services can be achieved in isolation. Services can be written in different languages and connected to different data tiers and platforms of choice. Such a componentised architecture demands a database platform that can support the different data types and structures and programming languages with ease.
Twelve Factor App
In the new ‘microservices’ era, application development has devised an approach called ‘the twelve-factor App’ written by Adam Wiggins and serves as an excellent guidance for building cloud native applications.
However, there a couple of factors (#4 and #5) that I would suggest need further examination when it comes to ensuring speed of delivery and data persistence.
– Treat backing services as attached resources: Backing services here refer to databases and the datastores for the most part. This means that microservices demand dedicated single ownership of schema and the underlying datastore.
– Strictly separate build and run stages: Separate build and run stages means the application should be executed as one or more stateless processes, and the state is often offloaded onto the backing service. This further implies that the databases and the datastores are expected to be stateful services.
Continuous Integration/Continuous delivery capability
Once the applications have been refactored as number of services it’s imperative that each service is deployable independently, requiring automated mechanisms for deployment and rollback, commonly referred to as continuous integration or continuous delivery (CI/CD).
The true value of microservices cannot be fully realised without an accompanying mature CI/CD strategy to allow the business to get new features and personalisation options into the market as fast as possible.
In a microservices architecture it means that database instances must also be able to easily spin up and spin down on demand as new features come online. With the help of the correct cloud native platform and supporting data platform, microservices become easily deployable.
The cloud native platform should handle the management of the services running on it; Your database should handle the data scaling and monitoring, adding of shards, rebalancing, re-sharding, or failover in the necessary event. The combined database and cloud native solution offloads the operational burden of monitoring the database, and the platform, allowing companies to spend more time developing and deploying quality software.
Multi-Cloud and Hybrid Deployment Models
As Enterprises adopt a multi-cloud or hybrid cloud + on-premises strategy it becomes imperative for the application code to be independent of the platform it’s expected to run on.
Traditional approaches to data access and data movement are time prohibitive. The legacy approaches involved creating replicas of the data in the primary data store in other operational data stores and data warehouses/data lakes, where data would be updated after many hours or days, typically in batches.
As organisations adopt microservices and design patterns, such delays in data movement across different types of data stores impedes agility and prevents organisations from forging ahead with their business plans.
High Availability of Data for Data Delivery
When you break a big monolithic application to microservices with each having its own lifecycle, how do you ensure data availability? The cloud native app developer should choose the data store based on the Recovery Point Objective (how much data will I lose), Recovery Time Objective (when an event failure occurs, how long will it take for the service to come back), high availability characteristics, installation topology and failover strategy. Single node database instances affect not just failure scenarios but client-downtime events, such as version upgrading, impacting availability.
Get the Database Fundamental Requirements Correct
Incrementally migrating a monolithic application to the microservices architecture typically occurs with the adoption of the strangler pattern, gradually replacing specific pieces of functionality with new applications and services. This means that the associated datastores also need to be compartmentalised and componentised, further implying that each microservice can have its own associated data store/database.
From the data perspective this means:
- The number of database instances increases with each microservice – again referencing back to spinning up/down on demand.
- For these microservices to communicate with each other, additional HTTP calls, over something like a convenient-to-use REST API, are needed – demanding flexible extensibility across any platform and language. In many cases microservices simply publish events indicating changes, and listeners/subscribers update the associated applications.
Until recently, sub-millisecond response times were reserved for a few specialty applications. But, in today’s world of the microservices architecture, this is a must-have requirement for all applications. This latency requirement necessitates the highest-performance, most scalable database solution that’s available.
For data replication, batch mode used to be a popular approach. But for today’s ‘real-time’ applications, replication with event store and event sourcing are getting a lot more traction.
In microservices apps that are loosely coupled, and need to share data, there is a need for active/active data replication with tunable consistency. Many organizations employ active/active deployment models for many reasons such as:
- Shared datasets among microservices that are being continually updated
- Seamless migration of data across datacenters so user experience is not impacted
- Mitigating failures scenarios and failover to a second datacenter to minimize downtime
- Handling high volume of incoming traffic and distributing load across multiple servers with seamless syncs and
- Geographically distributed applications (like a multiplayer game or a real-time bidding/polling application) where data needs to be in sync across geos
This is where in-memory databases like Redis Enterprise come to the fore and are disrupting traditional systems architecture. They reside in RAM at the heart of where the processing happens. If you can put the data in the same space as the processing takes place, you’re able to then deal with large volume of data at speed.
The system is expected to deliver a response within 100 milliseconds to request from an app, and this means the database latency must be in the sub-millisecond level. That’s what Redis Labs really focuses on and with that speed, comes the instant customer experience that we all expect. Additionally, Redis Enterprise can be persisted and the workloads can be distributed between RAM and Flash – thus delivering the required durability and cost effective operations.
With the correct consideration of the topic areas discussed, organisations will be able to transform their monolithic legacy application to an agile, scalable microservice architecture that will deliver real ‘instant data’ customer experience that’s at a competitive advantage.