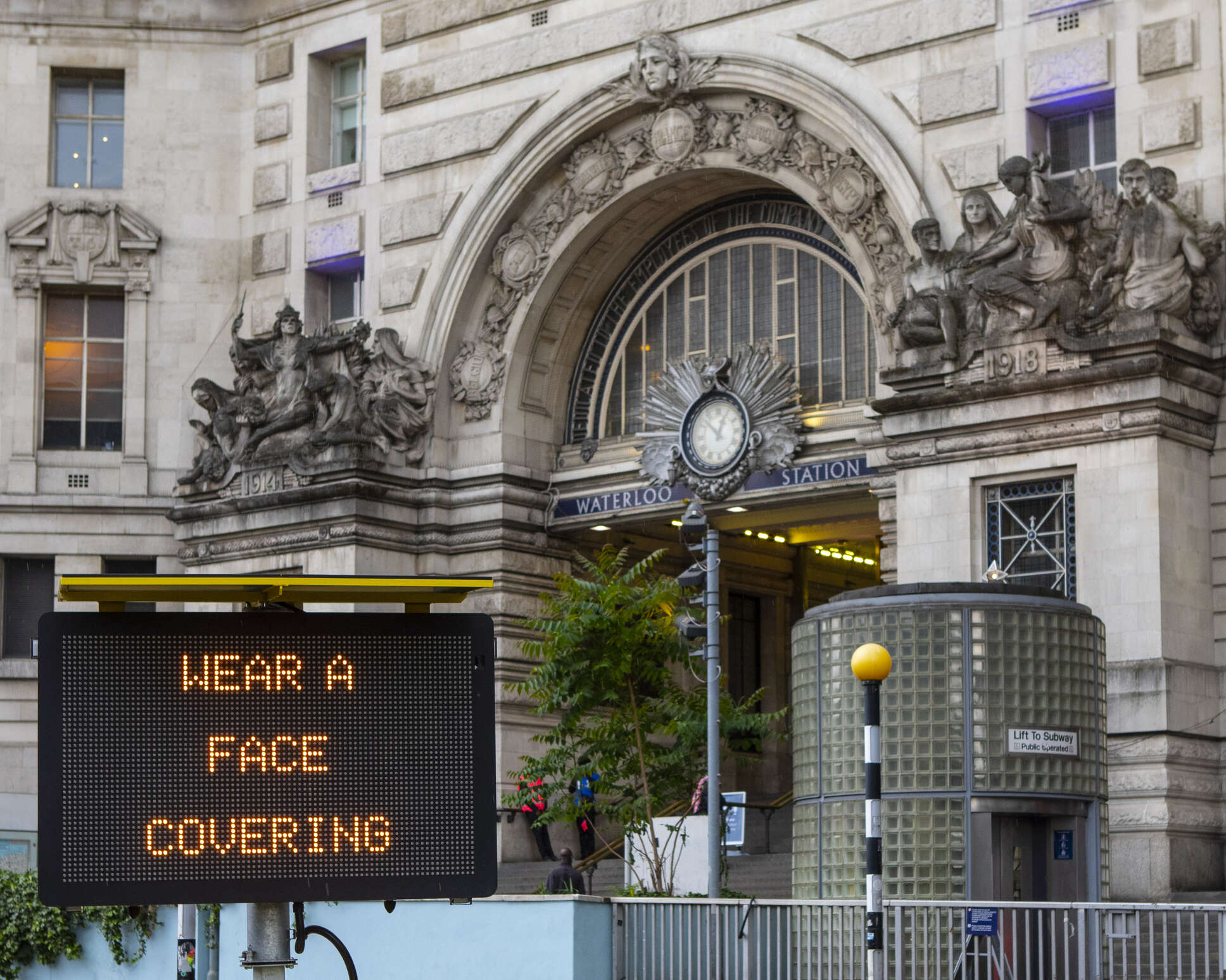
For local authorities such as the London Borough of Lambeth, the pandemic created an urgent need for data to help direct their emergency response. At Lambeth, this revealed shortcomings in how data projects were prioritised and delivered. Matthew Weaver, the council’s interim head of data at the time, tells Tech Monitor how he freed up the data team’s time to focus on what really mattered.

The pandemic revealed shortcomings in the way the London Borough of Lambeth delivered and prioritised data initiatives. (Photo by chrisdorney / iStock)
Transforming data at the London Borough of Lambeth
Weaver was brought in as interim head of data a little over a week before the pandemic struck in the UK. Lambeth’s previous head of data and analytics had recently left, and Weaver was tasked with addressing some operational issues and helping to recruit their successor.
His first step was to initiate a value-mapping exercise. “I spoke to all the key departments, listed all their pains and gains, then got all the main stakeholders in a room and ranked them by value and effort,” Weaver recalls. “How much effort is it? How much value does it supply?”
Another early initiative was to create a more transparent pipeline for the data analytics and insight team’s work. “I spoke to the data team’s [internal] customers and they said ‘They do a great job, but when something disappears into the pot, we never know when it’s coming out. There’s no visibility or transparency’,” Weaver says.
He therefore established a simple progress reporting tool that displayed the status, priority and stakeholders involved in all ongoing projects. This became especially critical in the pandemic, Weaver says. “From day to day, it was imperative that we understood exactly what people were doing and when things would be delivered.”
When the pandemic struck, the council’s long-term strategic objectives were sidelined to focus on emergency response. To support this, Weaver established four principles for data delivery: speed, priority, compromise, and continual improvement.
Accelerating data delivery
“The first thing was everything needed to be done quicker,” he explains. “We couldn’t just magic[ally] accelerate things, so what we did change the tooling.” Improvements in speed derived principally from low-code tools – in Lambeth’s case, Microsoft’s Power Platform – which allowed non-specialists to make adjustments to data applications.
Previously, when the team delivered a new visualisation or report, “there would be a whole load of change requests and issues, which meant the team’s capacity went down over time,” Weaver explains. “We became a support team, with very little capacity for new development.” Low code, and the appointment of new staff to support internal customers, “gave the engineers their time back.
The approach proved its worth when the Borough had to introduce surge testing in response to the possibility of a new variant in a particular neighbourhood. “We used [data analytics app] PowerBI to visualise which streets had been processed and ones that hadn’t,” Weaver explains. “We had people on the streets with tablet devices [delivering tests] and they were calling in and saying, ‘This is great, but if we had a view on how many outstanding [test] kits there are in a particular area or postal sector, that would help’. We were able to put that filter view in place and redeploy the application in a matter of minutes.”
Another time-saving initiative was the introduction of data pre-processing using Python. “We were taking feeds from the NHS, the Department of Health and Social Care, from Public Health England, from Age UK, and the data was coming in with different timeframes,” Weaver explains. “We always had to cross-reference it, but the guys were doing that with every feed. So we started to automate all of those and build automation pipelines using Python. Once we’ve solved the problem, that problem then remains solved until someone decides to change the data. [In that case] we could make a small change and continue the automation.”
Setting priorities for data innovation
The second and third pillars, priority and compromise, helped to ensure that the data team was working on the most valuable projects that could be delivered quickly. New requests for functionality were mapped on a grid with ‘value’ and ‘effort’ on its axes.
Anything that was high-value but high-effort was either dismissed or broken down into smaller tasks which were assessed on their own merit. “One piece was broken into about a dozen pieces, and seven of them we were able to push to one side,” Weaver says. “We ended up with five smaller tasks, with about 40% of the total effort, which we were able to deliver during the pandemic.”
That prioritisation effort was accompanied by a new approach to engaging with the rest of the organisation that allowed the data team to challenge some of the requests they received. “Some senior stakeholders would ask for things and they would have just no value,” recalls Weaver. “We had to be really quite tough and challenge them.”
Some senior stakeholders would ask for things and they would have just no value. We had to be really quite tough and challenge them.
This, Weaver says, was the most difficult components of his new approach. “Local government has a strict hierarchy,” he says. “I couldn’t break that but I had to make it a little more flexible to [allow] people to challenge. You may be [dealing with] someone at a higher pay scale than you, but you are the expert with the data, not them.”
One approach Weaver took was that if anyone on his engineering team was pulled into a second meeting to discuss a request, he joined in their place. “My job was just to say ‘I’m sorry, but this won’t happen today, this may not happen this week’, and be really quite firm.” Weaver’s background as a mathematician helped in the regard, he says. “I was able to sometimes say, ‘mathematically, this just does not stand up’.”
These efforts helped Lambeth’s data capability to be laser-focused on value, Weaver says. “We became almost clinically precise [about] the things we had to do,” he explains. “People weren’t used to being challenged but, after a while they started to value the opinions we were giving. And people even came back and said ‘I did ask for that [but] I’m glad you said ‘no’.”
Another way Weaver boosted the value of the data team’s work, he says, was to get them involved in projects early on. “People think that data comes at the end of the line,” he says. “A business initiative gets delivered and then they say, ‘We’ve got some data coming out of it, can we draw some pretty graphs and make any sense of it?’ That’s really not the best way to do things.”
By getting involved in new initiatives early on, data experts are able to identify the project’s objectives and advise on how best to measure them. “Once we know the data you need, we can collect things that aren’t currently being collected to make sure we have the right information,” explains Weaver.
Focusing on what matters
In all, Weaver characterises his approach as “getting good engineers and buying their time back so they can focus on real problems.”
Freeing up data scientists from technical tasks and allowing them to focus on solving problems for the organisation was invaluable, Weaver explains. “Being a good data scientist is 50% about domain knowledge and understanding what you’re trying to achieve,” he says. “We were [previously] far too close to the technical coalface. We had to drag ourselves away from that, trust the tools and processes to help us more, so we could engage with end users and stakeholders to really understand how we can help.”
Being a good data scientist is 50% about domain knowledge and understanding what you’re trying to achieve.
Not only was this approach more valuable for the council, it was also more engaging for the team. “Rather than [working on a] small, focused challenge where they couldn’t really see what the big picture was, they started talking with the end goal in mind so they could trace it all the way back,” he explains. “And it gives you more sense of fulfilment when you know you’re solving a real business problem.”
Weaver has now left Lambeth, having helped to recruit a permanent successor. His initiatives were well-received by senior management and are still in place after his departure, he says, and his work has led to a wider value-mapping effort across the organisation. “It helps makes sense of lots of people shouting for priority,” he says.