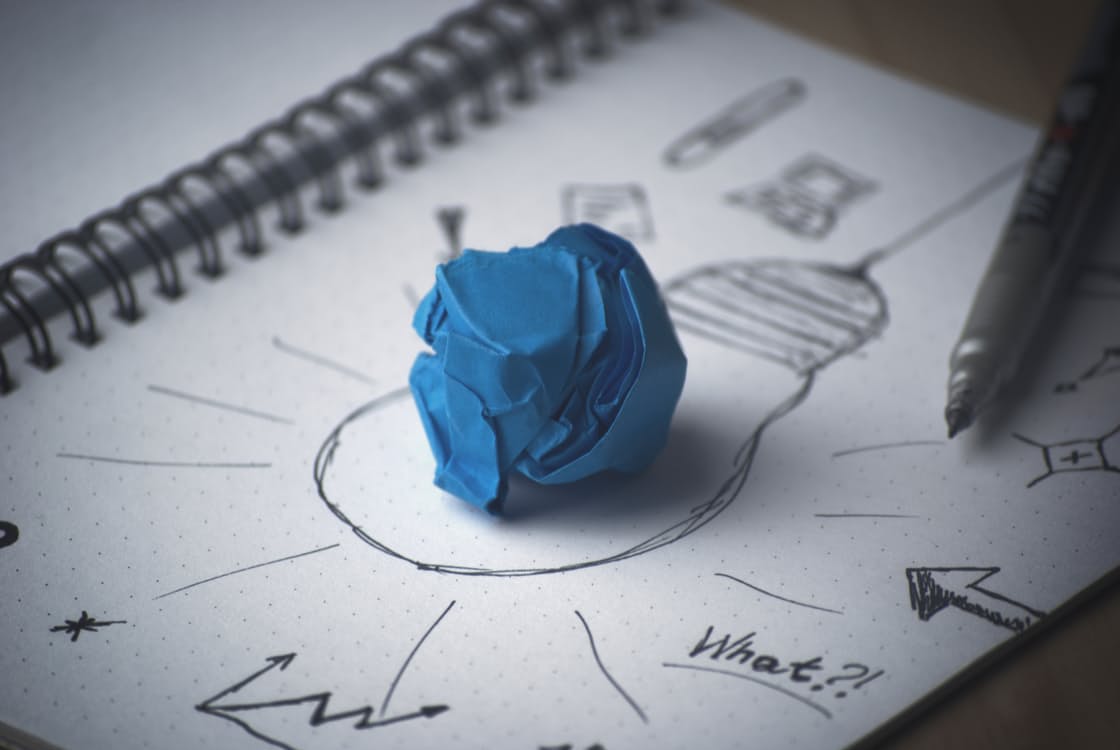
Over a beer with a customer recently, he told me his biggest frustration; ‘I don’t have a big data problem, I have a big data management problem!”

I was able to quickly reassure him he wasn’t alone; the explosion in big data has created a massive management challenge for every organisation. In essence, organisations are severely challenged with efficiently extracting value from the ever increasing volumes of data at their disposal. They know ‘there’s gold in them thar hills’ but they are having a hard task extracting it!
For many, the solution seems to be to invest in an ever larger army of data engineers and data scientists. But with both job titles commanding an average of $150k salary in Silicon Valley, while they can really help with the big data challenge, these people are expensive. And they’re also difficult to find because they are much in demand. So, getting the recruitment strategy right for bringing them on board is critical, both in financial terms but also from an operational efficiency perspective.
But, given they are so critical to your data strategy, how do you ensure you get the best out of your data scientists and data engineers?
First things first, let’s ensure we understand what the difference between a data scientist and a data engineer really is because, if we know this, then we know how best to direct them to drive value for the business. In the most simple of terms, data engineers worry about data infrastructure while data scientists are all about analysis. Boiling it down even more, one prototypes and the other deploys. Is one more important than the other? That’s a bit like asking whether a fork is more important than a knife. Both have their purposes and both can operate independently. But in truth, they really come into their own when used together!
Read more: Why data scientists are rock stars of the tech world
Let’s get more granular and explore what makes a ‘good’ data scientist, and what makes a ‘good’ data engineer…
* A good data scientist will apply scientific techniques (decision trees, regression, clustering etc) to perform descriptive, prescriptive or predictive analytics. And by doing so they’ll be able to tease insights out of the data quickly and efficiently
* They may not have a ton of programming experience but their understanding of one or more analytics frameworks is essential. Put simply, they need to know which tool to use (and when) from the tool box available to them. And just as critically, they must be able to spot data quality issues because they understand how the algorithms work
* A large part of their role is hypothesis testing (confirming or denying a well-known thesis) but the data scientist that knows their stuff will impartially let the data tell a story
* Visualising the data is just as important as being a good statistician so the effective data scientist will have knowledge of some visualisation tools and frameworks to, again, help them tell a story with the data
* Lastly, the best data scientists have a restless curiosity which compels them to try and fail in the process of knowledge discovery
So, let’s now characterise our ‘good’ data engineer…
- To be effective in this role, your data engineer needs to know the database technology. Cold. Teradata, IBM, Oracle, Hadoop are all ‘first base’ for the data engineer you want in your organization
- In addition to knowing the database technology, the data engineer has an idea of the data schema and organization – how their company’s data is structured, so he or she can put together the right data sets from the right sources for the scientist to explore.
- And your data engineer will be utterly comfortable with the ‘pre’ and ‘post’ tasks before data science will even occur. The “pre” tasks mostly deal with what we call ETL – Extract, Transform, Load. Often it may be the case that the data science is happening not in the same platform, but an experimental copy of the database and often in a small subset of the data. It is also frequently the case that IT may own the operational DB and may have strict rules on how/when the data can be accessed. A data science team needs a “sandbox” in which to play – either in the same DB environment, or in a new environment intended for data scientists. A data engineer makes that possible. Flawlessly.
- Turning to ‘post’ tasks, once the data science happens (say, a predictive model is built that determines ‘which credit card transactions are fraudulent’), the process needs to be ‘operationalised’. This requires that the analytic model developed by the data scientists be moved from the ‘sandbox’ environment to the real production/operational database, or transaction system. The data engineer is the role that can take the output of the data scientist and help put this into production. Without this role, there will be tons of insights (some proven, some unproven) but nothing put into production to see if the model is providing the business value in real time or not.
Ok, so you now understand what ‘good’ looks like in terms of data scientists and engineers but how do you set them up for success?
There are some simple steps that will drive value.
First, create the right operational structure to allow both parties to work collaboratively and to gain value from each other. Both roles function best when supported by the other so create the right internal processes to allow this to happen. Let this not become a tug of war between the CIO and the CDO – where the CDO’s organization just wants to get on with the analysis/exploration, while the IT team wants to control access to every table/row there is (for what may be valid reasons).
Next, invest in the right technologies to allow them to maximise their time and to focus in the right areas. For example, our approach is to embed analytics directly into applications and reporting tools so allowing data scientists to be freed up to work on high value problems.
Lastly, don’t skimp on talent; if you find the right people pay them well and then keep them incentivised to stick around. Because they’ll find the ‘gold’ in your data for you and will repay your investment many times over.
To conclude, effective data scientists and data engineers are the key to solving your big data management problem. Get your strategy right and your organisation will thank you for it!