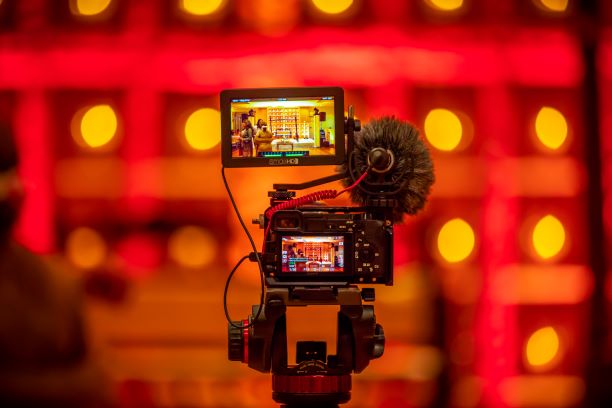
Enterprises with a large media archive often struggle with the challenge of transforming existing video archives into business value, particularly given the challenges of content discovery at scale: content categorisation is often flawed and manual tagging is expensive, error-prone and scales badly.
Microsoft thinks its upgraded product is the solution – and it’s a poster child for AI.
“Multi-modal topic inferencing” in Microsoft’s Video Indexer tool takes a tripartite approach to automating media categorisation: transcription (spoken words), OCR content (visual text), and facial recognition; operating under an innovative supervised deep learning-based model. It can even recognise moods.
What is Video Indexer?
Video Indexer is a cloud application built on a raft of Microsoft Azure tools, including Media Analytics, Search, Cognitive Services – such as the Face API, Microsoft Translator, the Computer Vision API, and Custom Speech Service – and more.
It’s designed to help business users extract insight from videos using and provide services ranging from keyframe extraction to sentiment analysis; visual content moderation (like detecting “racy” visuals) and brand identity recognition.
Video Indexer: Shift from Keyword Extraction
Oron Nir, a senior data scientist in Microsoft’s Media AI division said: “[This tool] orchestrates multiple AI models in a building block fashion to infer higher level concepts using robust and independent input signals from different sources.”
The technique is a step-change from Video Indexer’s previous keyword extraction model, which pulls out and categories only according to explicitly mentioned terms.
Multi-modal topic inferencing, by uses a “knowledge graph” to cluster similar detected concepts together. In practice, it does this by applying two models to extract topics.
As Nir explained in a recent blog: “The first is a deep neural network that scores and ranks the topics directly from the raw text based on a large proprietary dataset. This model maps the transcript in the video with the Video Indexer Ontology and IPTC.”
“The second model applies spectral graph algorithms on the named entities mentioned in the video. The algorithm takes input signals like the Wikipedia IDs of celebrities recognized in the video, which is structured data with signals like OCR and transcript that are unstructured by nature.”
He added: “To extract the entities mentioned in the text, we use Entity Linking Intelligent Service aka ELIS. ELIS recognizes named entities in free-form text so that from this point on we can use structured data to get the topics. We later build a graph based on the similarity of the entities’ Wikipedia pages and cluster it to capture different concepts within the video.”
For facial recognition, it can now automatically identify over 1 million celebrities – such as world leaders, actors and actresses, athletes, researchers, business and tech leaders across the globe. Now if those legacy media archives could just be got off those piles of magnetic tape in a dusty cellar somewhere…
See also: Did Amazon Just Kill Tape Storage?