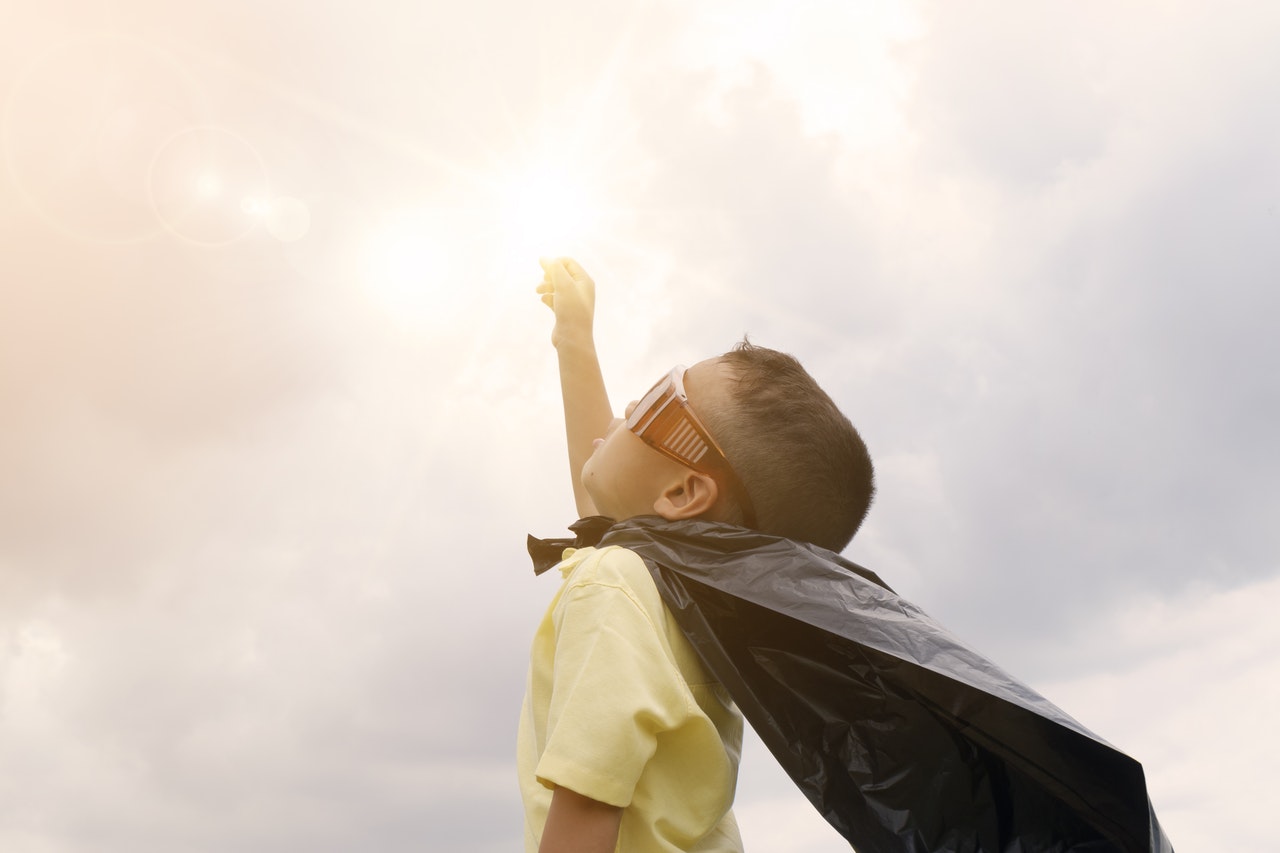
Microsoft Azure has added a Machine Learning managed service to its portfolio of cloud applications, priced at anywhere between £30 and £30,000 per month depending on instance, vCPU and RAM required.
Australian life insurance company TAL is already using the tool to improve assurance and Elastacloud, a London-based data science consultancy is using it to help utility clients predict energy demand, Azure said.
“We [use the tool to] support BSUoS [the National Grid’s ‘balancing use of system’ charge] Forecast with no virtual machines and nothing to manage. We built a highly automated service that hides its complexity inside serverless boxes,” it cited Elastacloud’s COO Andy Cross as saying.
TAL meanwhile has reportedly moved from being able to only randomly selected 2-3 percent of cases to reviewing 100 percent of cases. The service supports popular open-source frameworks such as PyTorch, TensorFlow, and scikit-learn.
(As Databricks CEO Ali Ghodsi earlier put it: “To derive value from AI, enterprises are dependent on their… ability to iteratively do machine learning on massive datasets. Today’s data engineers and data scientists use numerous, disconnected tools to accomplish this, including a zoo of machine learning frameworks.)
See also: Open Source Platform Aims to Democratise “Machine Learning Zoo”
Accessible machine learning is increasingly a driver for enterprises eyeing “all in” cloud migration. Azure rival AWS recently cited its SageMaker machine learning tool as contributing to an “all in” move to the cloud by customer Korean Air.(The airline is using maching learning to improve predictive aircraft maintenance systems and automate repairs, they said).
Azure Machine Learning: Deploy to Cloud or Edge
To simplify and accelerate machine learning, Azure Machine Learning has been built on design principles that include offering a “familiar and rich set of data science tools” and simplified deep learning frameworks”, Venky Veeraraghavan Group Program Manager, Microsoft Azure said in a blog post.
Experiment tracking and management of models deployed in the cloud and on the edge can be accessed from any Python environment running anywhere, including data scientists’ workstations, he added, pointing to DevOps capabilities.
“The service will provision, load balance, and scale a Kubernetes cluster using Azure Kubernetes Service (AKS) or attach to the customer’s own AKS cluster. This allows for multiple models to be deployed into production” Veeraraghavan said.
He added: “The cluster will auto-scale with the load. Model management activities can be done with both the Python SDK, UX or with Command Line Interface (CLI) and REST API, which are callable from Azure DevOps. These capabilities fully integrate the model lifecycle with the rest of our customer’s app lifecycle.