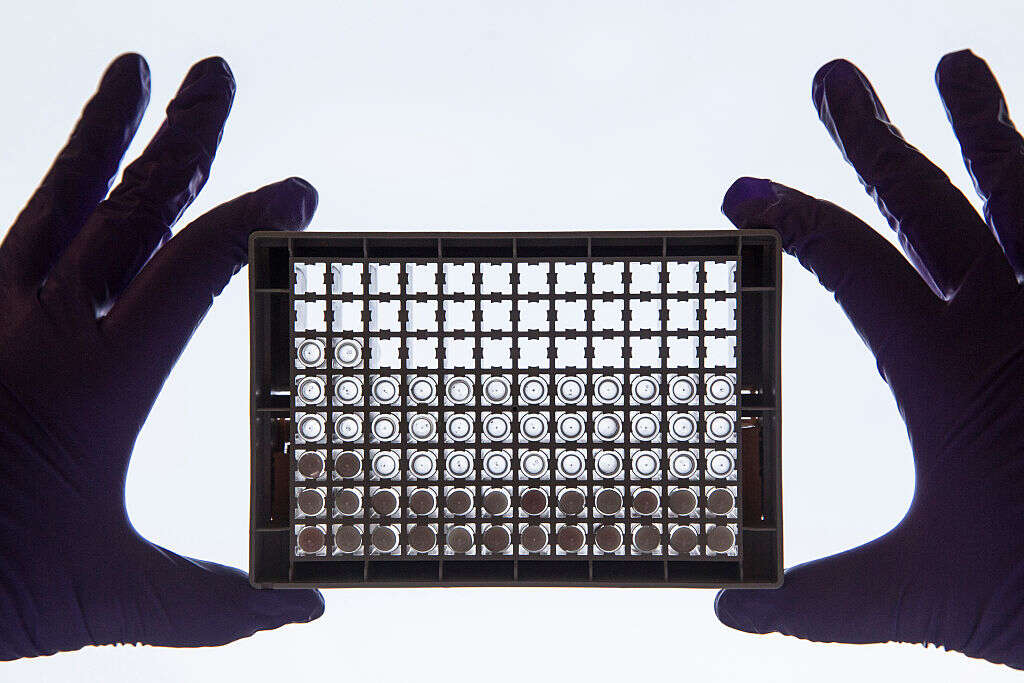
“Without the aid of statistics,” wrote the pioneering French surgeon Pierre Charles Alexandre Louis, “nothing like real medicine is possible.” That is as true today as it was in 1837. Effective diagnosis and treatment of disease rests on the quality of data a doctor can access, whether it is on anything from the patient’s symptoms, to the circumstances of their infection and their family history. Modern medicine, one could say, is an exercise in pattern recognition – something to which artificial intelligence should be ideally suited.
Robert Wachter certainly subscribes to this vision. An MD and professor of the department of medicine at the University of California, San Francisco, Wachter is a leading authority on the technological future of the healthcare profession. He believes that few tasks in modern medicine could not be improved by the judicious application of AI. Its promise, says Wachter, “is almost limitless.”
This conviction has been echoed repeatedly across scientific literature and the mainstream media. For years, the public has repeatedly been left with the impression that the dawn of AI in healthcare is just around the corner, leading to transformative improvements in diagnosis, workflow and cost reductions. And a few applications have emerged glimmering with possibility, from computer vision applications capable of diagnosing eye diseases, to detection tools for prostate cancer and algorithms specially tasked with containing and finding treatments for Covid-19.
There’s just one problem: few of these solutions have succeeded in jumping from medical journals into clinical settings. In practice, crafting effective medical AI applications has turned out “not to be easy in essentially every dimension you look at,” explains Wachter. From the poor quality of the datasets used to train relevant machine learning algorithms to resistance from healthcare practitioners, medical AI faces hurdles at every turn.
That hasn’t stopped Big Tech from taking an interest. Amazon, Google, Apple and Microsoft have all devoted significant time and resources to disrupting the healthcare market using machine learning algorithms, collectively investing some $3.7bn into the sector in 2020. Indeed, these corporations seem undeterred by the trail of flawed and discontinued tools that result when Silicon Valley visits the clinic, from an advisory tool for oncologists developed by IBM and internally criticised for being ‘unsafe and incorrect’ to Google’s shaky attempts to automate the detection of diabetic retinopathy. Despite these failures, there’s little sign that Big Tech will cease trying to make medical AI a reality; the size of the global healthcare market alone was estimated to be worth some $8.45trn in 2018.
None of this investment will produce results, says Dr Leo Anthony Celi, unless these companies take a long, hard look at some of the more systemic problems afflicting research into medical AI applications. For Celi, the principal research scientist at the MIT Laboratory of Computational Physiology (LCP), it’s high time that the field pays attention to how human biases are being baked into these programs. “I’m actually very glad that there is this death valley” between research and clinical application, says Celi. “Because we’re not ready.”

A radiologist in Brazil uses an AI assistant to help diagnose lung conditions related to Covid-19.
(Photo by NELSON ALMEIDA / AFP)
Data deficiencies
The promise of AI lies in its ability to automate complex, if mundane, tasks. Its ability to do so, however, is dependent on both the data used to train the application and the quality of the information it is using to make a decision. ‘Garbage in, garbage out’ is an all-too-familiar problem for those working in healthcare AI.
That’s because so much of medical data is recorded in ways that machine learning algorithms find difficult to parse. Health records in the UK and the US, for example, are often a mishmash of handwritten notes and database entries (in the former, some 71% of the social care sector is unable to readily access digitised medical records.) And while both countries have made progress in shifting to electronic records systems, any machine learning algorithm designed to use these databases as ground truth would have to contend with reams of extraneous information contained in multiple systems that often don’t talk to one another. As a result, there are potentially “a huge number of inaccuracies” to weed out before such a corpus can be used to train an AI application, says Wachter.
All this limits the potential of machine learning algorithms, not least in diagnosis and treatment recommendations. Even so, says Wachter, AI applications can still make a positive difference when it comes to parsing scans.
“It’s easy to imagine how [an AI] would be as good as, if not better than humans, in the areas that really involve processing visual data,” he explains. Even though analysing CAT scans and MRI readouts form a relatively small part of what healthcare is all about, he explains, “if somehow we could snap our fingers and AI read all the mammograms, read all the CAT scans, you’d save a fair amount of money, and you’d move things along quickly.”
Numerous experimental studies have shown precisely how this can be achieved. However, few have worked as well in clinical settings as they have in the laboratory. What also worries researchers like Celi is the ability of some of these applications to spot things that should be extraneous to the scan itself. One paper by his working group at MIT recently found that it was easy for AI applications to detect the race of a patient without access to substantiating clinical data. “And that is, of course, problematic for us because we know that computers at some point are going to use these sensitive attributes to make decisions, even if they’re not related to the prediction,” says Celi.
The paper’s working hypothesis is that the medical equipment used to originally capture the scans in the dataset was optimised for a white male population. Most X-ray machines and CAT scanners are “made in the US or in Germany, and of course, their test subjects are white individuals,” explains Celi. This means that the performance of the application will always be skewed whenever it encounters female patients, or those of colour.
Healthcare AI: baked-in bias
This points to a more fundamental problem with AI in healthcare, argues Celi. Researchers in the field have been too focused on improving the accuracy of models in controlled laboratory settings, he argues, and less on how the ground truth defining that accuracy has been moulded by the racial, economic and gender biases of society at large. Their impact is already being felt across healthcare, with algorithmic assessments leading to Black patients in the US receiving a lower quality of care than their White counterparts, in addition to being placed further down transplant lists.
“It’s no surprise that these algorithms are going to be prejudiced and biased, because the way we practice medicine is tainted with human biases,” says Celi. “We need to pause and truly understand how the computers are making decisions. Because, chances are, the computers are going to learn from humans.”
The problem of bias, Celi adds, has been compounded by the sector’s prioritisation of computer science expertise over and above medical knowledge and workflows. “When you look at submissions to machine learning papers, what you see is that the authors are still 99% computer scientists,” he says. “I think a lot of our investments are going to waste, because we’re not taking advantage of different perspectives, different expertise, banding together to address these problems that we’ve had for the last fifteen years.”
Even so, it’s not as if the medical community has been clamouring for more involvement in machine learning studies. If anything, doctors have been historically sceptical of the benefits of AI in healthcare, to the point of dismissing the idea that outside experts could make a positive contribution. “It’s very, if I may, arrogant,” explains Celi. “We think that people who are not in the field don’t understand the problem well. And we have kept them out.”
[Doctors] think that people who are not in the field don’t understand the problem well. And we have kept them out.
Dr Leo Anthony Celi
Some of this resistance is understandable, says Wachter, given the high standards for success in medical practice. Nevertheless, he adds, AI is often held to a different standard to human practitioners. “We tolerate errors by humans all the time,” says Wachter. “We don’t say we need to close down the radiology department because someone missed a finding. It’s just the nature of the beast.”
There is also an element of fear among doctors – of not only losing one’s job, but the potential of malpractice suits arising from mistakes made by an AI. It is still unclear where the blame would lie in such a scenario. Additionally, says Wachter, the rise of social media means that “there are now many, many megaphones to amplify the consequences of a technological ‘miss’.”
One way to convince doctors that AI should be welcomed is by emphasising that machine intelligence is unlikely to replace human expertise for a long time to come. Indeed, it is argued – often by new entrants into the marketplace – that machine learning applications work best when they act in a purely assistive capacity. “How I think about AI doing very well is in augmenting care, augmenting the physician’s decision making,” says Nirav Shah, chief medical officer at digital health company Sharecare.
The best way to convince a doctor that a technical innovation is worthwhile, after all, is pointing out how and why it can improve their workflow. Ignoring this factor has “been a large part of why AI hasn’t taken off” in the sector, says Shah. “If you can integrate AI into clinical workflows in ways that are additive, easy, lower cost and patient-centred, it’s going to make a big difference.”

Advocates argue that AI is best deployed in healthcare in an assistive capacity but that requires an understanding of doctors' workflow-related pressures. (Photo by Dan Kitwood/Getty Images/Cancer Research UK)
AI in healthcare: looking ahead
Proponents of medical AI applications also have to overcome the suspicion of patients themselves. Not only are people less likely to sign up for free diagnosis sessions if supervised by a machine, a recent survey found, they are even willing to pay more to be treated by a human doctor.
Shah believes that the key to overcoming this suspicion is making AI applications relevant to their lived experiences. This can be partly achieved by weaving machine learning algorithms into digital healthcare tools on smartphones, he says. Not only would this allow patients to take back control of certain aspects of their care, but also allow for the creation of much larger clinical trials – and more relevant medical data for research into medical AI applications generally.
That process could be accelerated by the deepening involvement of big tech companies like Amazon and Microsoft in medical AI. “We need patients engaged with their own health,” says Shah. “And what are these companies really good at? They’re all really good at keeping your attention. That’s their superpower. If we can get that for the greater good of each individual on their own health journey, that would be amazing.”
Celi is also cautiously optimistic, albeit for different reasons. “The biggest change that we’re seeing now is [that] we’re allowing people who are not typically part of the healthcare ecosystem to come in and provide a different perspective,” he says – particularly those computer scientists, sociologists and engineers whose expertise was previously siloed in the laboratory. “I think there’s definitely more of the other disciplines coming in and working with people [on the] inside.”
There are two projects from the US National Institutes of Health – the ‘Bridge to AI’ scheme and the ‘AIM-AHEAD’ initiative – that Celi finds especially promising. The former promotes data sharing across the entire research community, while the latter aims to diversify the current corps of engineers, medical practitioners and computer scientists that currently make up the field.
Until such projects begin to erode the systemic problems of bias afflicting the field, it seems that the progress of AI in healthcare will continue to be haphazard. Even so, says Wachter, progress is inevitable. “I can’t see an endgame where AI has not transformed medicine,” he says. “I just can’t tell you if that endgame is five years away, or fifty.”