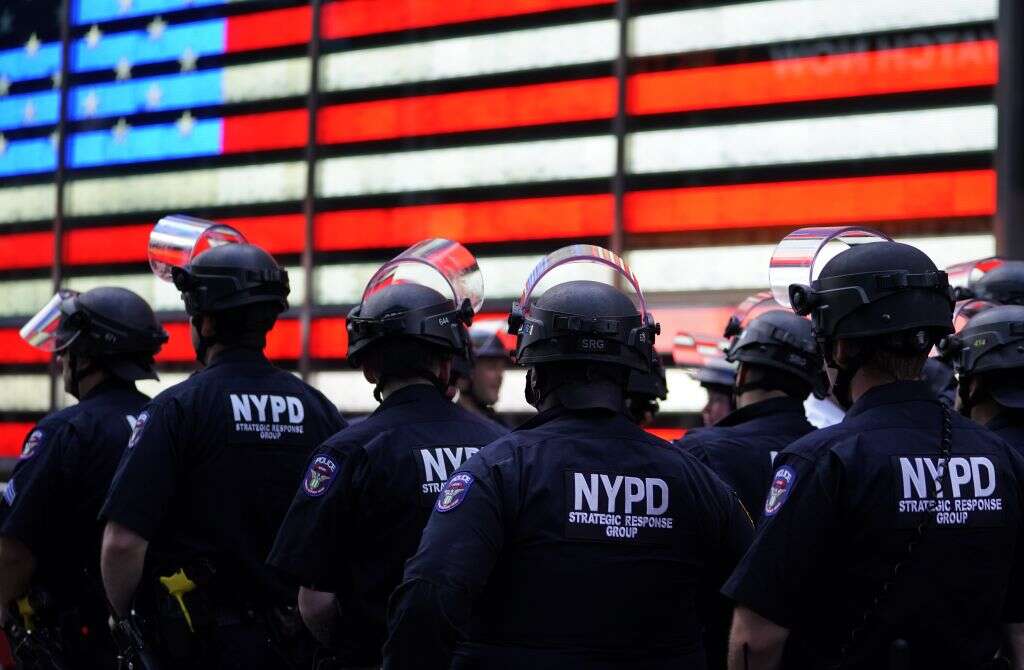
The pitch was simple. In August 2019, lobbyist Bryan Smith told a board of Utah’s police chiefs, municipal officials and emergency responders that his company, Banjo, could provide them new insights on where crime was occurring in real time. To be sure, that would require running huge data flows through its proprietary algorithm – CCTV camera feeds, 911 calls, emergency vehicle locations – but Banjo would achieve this without endangering the personal privacy of anyone caught up in this new surveillance dragnet.
The board was convinced. Armed with a contract allowing Banjo to operate in every county in Utah, by January 2020 the company began to receive data flows from around 70 municipalities, the state’s Highway Patrol, and Department of Public Safety. Any optimism among lawmakers as to Banjo’s effectiveness, however, was short-lived. In May of that year, the company’s CEO resigned after his past as a white supremacist was exposed, prompting the suspension of its contract with the state of Utah and an audit into its practices.
“It turns out that they had no artificial intelligence at all,” says Matthew Guariglia, a policy analyst at the Electronic Frontier Foundation. In fact, as the audit explained, Banjo’s ‘Live Time’ solution was doing nothing a human emergency response team couldn’t handle – a fact that the state should have verified, instead of taking the company’s claims at face value. Even so, the level of scrutiny around Banjo is rare, says Guariglia. Absent the full force of a state investigation, “we have no idea what’s happening inside these companies.”
The results of predictive policing solutions that actually use AI are often just as troubling. Such applications have been criticised for leading police departments to misallocate resources, sending patrols out to neighbourhoods already familiar with the wailing sirens of squad cars. Worse, since many of these algorithms rely on historical arrest data, such applications could entrench instances of racial bias in policing methods that have persisted for decades.
It is, says Guariglia, a civil liberties crisis waiting to happen. Such systems “erode the presumption of innocence,” he says. “If police are deployed to an intersection where an algorithm tells them there might be a crime tonight, how are they supposed to regard any people who happen to be walking through that intersection? Anybody in that hotspot has, by definition, a higher presumption of guilt.”

From predictive policing to over-policing
Police have been collecting statistics on crime for over a century. But concerted efforts at using those figures to inform where, when and how to deploy police patrols to fight crime came much later.
“The rise of big data policing happens in the 1990s and early 2000s,” explains Guariglia, led by the New York Police Department’s Computer Statistics (COMPSTAT) system. Essentially a combination of database technology and pins on a map, COMPSTAT has been credited as a major factor behind New York’s falling crime rates in the late 1990s.
After similar systems were adopted by other police departments around the world, machine learning elements began to be added into the mix by private contractors in the early 2010s. Ostensibly, the addition of AI allowed police not only to map hotspots of criminal activity, but anticipate where it was likely to happen next.
The results have been mixed. In 2011, the Santa Cruz police began to use a system provided by PredPol (now Geolitica). At the time, it seemed a relatively inexpensive way of boosting the department’s effectiveness without hiring extra officers. “The feedback I’ve received is that there is appreciation that it has validated intuition or provided a new focus area that wasn’t known,” the department’s crime analyst told The New York Times, crediting the system with a 19% fall in burglaries. “The worst-case scenario is that it doesn’t work and we’re no worse off.”
Six years later, incoming police chief Andy Mills imposed a moratorium on its use, saying the system had done more harm than good. All it did, he said, was inform the SCPD where “to do purely enforcement,” leading the department to over-police certain neighbourhoods around the city without building productive relationships with the community that might have helped tackle the root cause of criminal activity. Last year, the city became one of the first in the US to ban the use of predictive policing applications outright.
In at least one case, this over-policing, primed by AI, has come close to being lethal. In 2013, Robert McDaniel received a visit from officers of the Chicago Police Department (CPD), who subsequently informed him that its predictive policing program – one of the few that tracks individuals – had told them that McDaniel was highly likely to be involved in a shooting incident, given his prior proximity to known gang members.
As McDaniel would later tell The Verge, the officers had no idea whether he would be the victim or the perpetrator. Regardless, the department placed him under surveillance. Its prediction about McDaniel became a self-fulfilling prophecy. Alarmed at the sudden increase in police presence around him, he was later shot and wounded by someone who, he suspects, believed he was an informant.
As with so many contentious applications of AI, the trouble lies in the data. “Predictive policing relies on historic data of arrest records,” explains Guariglia. The argument goes that this data is often reflective of where police departments choose to concentrate patrols: invariably poorer, majority Black or Hispanic neighbourhoods. As a result, not only do predictive policing systems end up laundering racially biased arrest patterns that have built up over decades, but stigmatise entire communities.
Even using alternative datasets to train predictive policing algorithms can be problematic. A study by researchers at the University of Texas at Austin and Carnegie Mellon compared victim reports with actual crime data from the city of Bogotá, in Colombia. Their hypothesis, explains the study’s co-author Alexandra Chouldechova, that relying on such data would “result in disproportionate allocations of police, by virtue of certain communities just being more likely to report crimes than others.”
The resulting model was wildly inaccurate, predicting 20% more hotspots in crime-ridden neighbourhoods than actually existed. The study is reflective of broader conclusions on how racial and socio-economic biases influence the reporting of crime. Richer, white citizens, for example, are more likely to report a crime taking place if the perpetrator is black, while those who are routinely involved in illegal activity themselves are less likely to report crimes against them.
Predictive policing doesn’t always have to be inextricably associated with racial bias. Camden County Police Department (CCPD) in New Jersey has received praise for its focus on community policing and de-escalation tactics, as well as its chief’s support of the Black Lives Matter movement. It has also operated a predictive policing solution supplied by CivicScape since last year, which relies on inputs ranging from a citywide surveillance network of CCTV cameras, ShotSpotter sensors and GPS tracking software.
CivicScape has received qualified praise for publishing its source code (the application ultimately being adapted to clients’ needs). Even so, CCPD's approach, in which private contractors monitor surveillance networks, is one that many still find troubling. “When you try to get ever-better data to inform your decision-making through increased surveillance… you’re typically giving private contractors access to a lot of information that citizens didn’t really consent to sharing with private actors,” says Chouldechova. “We tend to live our lives in the open, and we don’t want to be necessarily tracked.”

The politics of predictive policing
Whatever its usefulness, the future of predictive policing in the US is likely to be determined by politics. Protests against police misconduct and calls to ‘defund’ departments have led US lawmakers to push for reforms that eliminate inequitable policing practices. Guariglia hopes that this will lead to new restrictions on predictive policing applications, although for the moment he is unsure whether the public has fully acknowledged its flaws.
“I think part of the problem is that many people just aren’t aware of how the technology functions,” he says, comparing the learning curve surrounding predictive policing to that of facial recognition. “Almost two dozen cities across the United States, including large cities like Boston, San Francisco and New Orleans, have banned the use of facial recognition. And predictive policing is right behind that.”
Municipal support for predictive policing also seems to be receding. Santa Cruz – the first city to institute a predictive policing program –banned its use outright last year; New Orleans and Oakland have followed suit.
But rising violent crime rates across the US could blunt progressive police reforms which, rightly or wrongly, have become associated with the controversial Defund movement. The recent election of Eric Adams, an ex-police officer who has resisted calls for NYPD budget cuts, as the Democratic mayoral nominee for New York City was seen by political commentators as a sign that the pendulum has swung back in favour of increased funding for law enforcement in all its forms.
Indeed, predictive policing has always offered forces a cost-effective way to appear tough on crime without increasing headcount, argues Guariglia. Whether or not it has an impact, “it sounds very good in a press release for a weary public who wants there to be less crime,” he says.
[Predictive policing] sounds very good in a press release for a weary public who wants there to be less crime.
Matthew Guariglia, Electronic Frontier Foundation
Even if public support were to increase for predictive policing, there remains the question of whether such programs enjoy legal standing. In April, a group of Democratic senators led by Sen. Ron Wyden of Oregon petitioned the Department of Justice for clarification on whether such systems were compliant with civil rights legislation. The case for police aerial surveillance – another potential data source for predictive policing programs – is also being tested. Earlier this month, a US appeals court ruled that law enforcement in Baltimore could not conduct warrantless aerial surveillance under the Fourth Amendment, following on from a similar ruling in Michigan.
These rulings are in keeping with historical rulings on Fourth Amendment cases, says Georgetown Law senior associate Clare Garvie. “Our right to privacy in the US is very much protected by building inefficiencies into law enforcement activity,” she says, a trend that could help to shut off predictive policing algorithms from accessing certain data flows in the US.
Such constitutional protections are weaker, however, in many other jurisdictions experimenting with predictive policing. Predictive policing programs have already been adopted in the UK with varying effectiveness, from the London Metropolitan Police’s Gang Matrix – instructed in February to remove the names of a thousand young, Black men from its register – to West Midland Police’s National Data Analytics Solution, the trial of which introduced so many errors that it was ditched for being ‘unusable.’ Interest in similar applications has also been witnessed among police services in Canada, Japan, the Netherlands, Denmark, China, Spain, Italy, Brazil, South Korea, South Africa, France and Germany.
Even so, Guariglia is hopeful that the poor results that come from the deployment of such systems will speak for themselves. “There’s a reason why a number of police departments who have signed up for predictive policing programs, which are exorbitantly expensive, have cancelled their subscriptions,” he says. “It seems like a lot of departments don’t get a tonne out of it.”