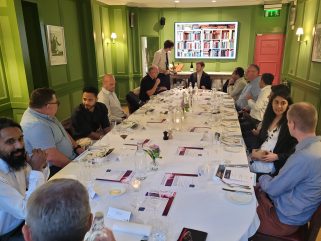
A recent Tech Monitor article invited readers to ‘Meet the CIOs that regret investing in AI’. According to the piece, by next year at least 30% of GenAI projects will be abandoned after proof of concept. Meanwhile, it quotes a survey of 1,200 IT professionals that suggests a quarter of respondents regret having invested in AI so quickly.
Which begs the question: have we reached GenAI’s trough of disillusionment?
Judging by the select group of senior IT leaders gathered for the latest Tech Monitor executive roundtable – convened in association with Lenovo and Intel® Xeon® Processors – the answer is a firm ‘no.’ At this London-based event, the technology practitioners present remained bullish about the potential of GenAI. Many are at a relatively early stage in their deployment but most, if not all, identified huge value – productivity gains and market leadership potential – from current and future GenAI projects.
Not that they lack misgivings. Some are struggling to corral their data, failing to get it into the kind of shape that will allow them to feed the large language models that will underpin future opportunities. Others are worried about security and ethics, excessive energy use, hallucinations, and a lack of in-house skills. One attendee is struggling to turn disparate AI projects into a coherent plan that he can take to senior leadership. “We have seven to eight on the go,” he asked, “but how do I take these individual initiatives and turn them into a strategy?”
Among the use cases attendees shared, many were familiar – an HR chatbot to onboard new starters and answer employees’ most common policy and procedure-related queries; an email sentiment checker that cautions an impulsive line manager against pressing send on an unnecessarily aggressive message; writing software that produces – at speed – formulaic and templated news stories that require little independent fact-checking; and tools that summarise huge amounts of research material found in legal offices, investment banks, insurance firms and elsewhere.
In most instances of GenAI, attendees insisted on having “a human in the loop” to sense-check the work of the AI. As has been noted previously, hallucinations are a feature of GenAI, not a bug (a model that learns the patterns and structures of input training data builds its ‘best guess’ response one word at a time.). It would seem that hallucinations will not deter organisations from using GenAI but will encourage them to put guardrails in place. Human augmentation appears the most likely approach.
The intelligence of AI is not yet fully formed. An AI learns from the data we feed it and then applies it with “brute force”, to borrow a phrase from one attendee. It will spot anomalies but can’t yet offer reasons for those anomalies. In other words, it can answer the ‘what’ but not the ‘why’ – or at least can’t answer the ‘why’ with anything approaching certainty. That will come, noted one attendee, with the introduction of artificial general intelligence. Until then, it was argued, AI will fail to pick up the nuances of questions asked. While, as humans, we can detect sarcasm or understand that the words not spoken matter as much as those spoken, this remains beyond the AI. For now.
A related concern is the ‘black box’ syndrome where the complexity of GenAI is such that it is impossible to reverse engineer the decision-making process. Coupled with the biases inherent in some of the data used in training sets, there was a shared concern that if an organisation couldn’t explain away the judgements AI makes, it must avoid AI-only decision-making.
A more practical challenge attendees face is getting their data into shape so it can be put to work. Cleaning data and extracting it from organisational silos are common challenges. Even more fundamental for one large insurance company represented on the night was getting information off the printed page and into digital form. Finally, data must be sufficiently regimented before it can be used in large language models. These are no small challenges for even the most well-resourced companies.
The final topic of the night: environmental sustainability. Illustrating the energy intensity of GenAI, Goldman Sachs recently asserted that processing a ChatGPT query requires nearly 10 times as much electricity as a Google search. To drive more sustainable behaviours, one attendee urged organisations to be more selective in their use of GenAI, picking and choosing projects with care. Another said that firms need to bring greater efficiency to deployment especially when it comes to training models, the most energy-intensive part of the GenAI process.
A third participant floated the idea of displaying a real-time energy consumption counter next to each query. Much like the calorie count that appears alongside the dishes on a restaurant menu, the stark figures in front of the user might encourage different behaviours. However, an instinct other than guilt appears to be driving behaviours right now. And that instinct, asserted one attendee, is FOMO – fear of missing out.
‘Resilience and Growth: How to Stay Ahead with AI’ – a Tech Monitor roundtable discussion in association with Lenovo and Intel® Xeon® Processors – took place on 18 September 2024 at Quo Vadis, London.