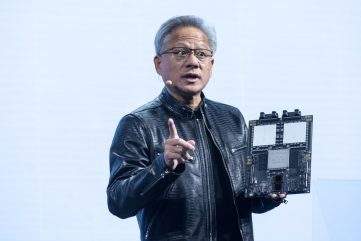
Jensen Huang had every right to feel chipper. Striding onto the main stage at Taiwan’s COMPUTEX conference in June, Huang represented a company whose graphics processing units (GPUs) had effectively made the generative AI boom possible. And there was more to come, Nvidia’s chief executive proclaimed, as he rhapsodised about how the firm’s chips could be found powering everything from AI accelerators and digital twins to bipedal robots.
“Physical AI is here,” said Huang. “This is not science fiction, and it’s being used all over Taiwan. It’s just really, really exciting.”
It seemed that Huang could do no wrong – not to the crowd, who burst in rapturous applause at the Nvidia founder’s pronouncements about its new state-of-the-art Blackwell chip, not to the ecstatic fan who asked the executive to sign her top two days later, and least of all among the GPU giant’s many investors. Later that month, the firm briefly became the world’s most valuable company, with a market capitalisation of over $3.34 trillion – an accolade indicative of the firm’s privileged position at the heart of the AI supply chain.
How time flies. Despite record sales of $30bn, Nvidia’s recent second-quarter results just weren’t enough to satisfy the markets, which sent the firm’s stock plunging southward by 6% in after-hours trading. As well as raising broader questions about whether we now find ourselves in the middle of an AI bubble, with too much invested in technology yet to yield the dramatic returns promised by its fiercest advocates, new doubts are emerging about the future of Nvidia itself.
Its rivals are certainly keen to stir the pot. COMPUTEX also saw rivals AMD and Intel plug alternatives to Nvidia’s GPUs just as capable of training the next generation of large language models (LLMs). Take, for example, the former’s Ryzen AI 300 Series processor, equipped – AMD claimed – with the world’s most powerful ‘neural processing unit’ and 12 high-performance CPU cores. Devices armed with these chips, it said, “offer more high-performance cores than any AMD-powered PC in its class.”
International competition regulators, too, also appear eager to cut the GPU giant down to size. Initially caught off guard by the generative AI boom, these watchdogs are keen to impose their will on a marketplace they perceive as being dominated by sweetheart deals between pioneering AI startups and established tech brands. In a recent speech to an audience in Washington D.C., CMA chief executive Sarah Cardell decried the formation of an “interconnected web” of 90 such investments and partnerships formed by a select number of big tech brands – Nvidia among them.
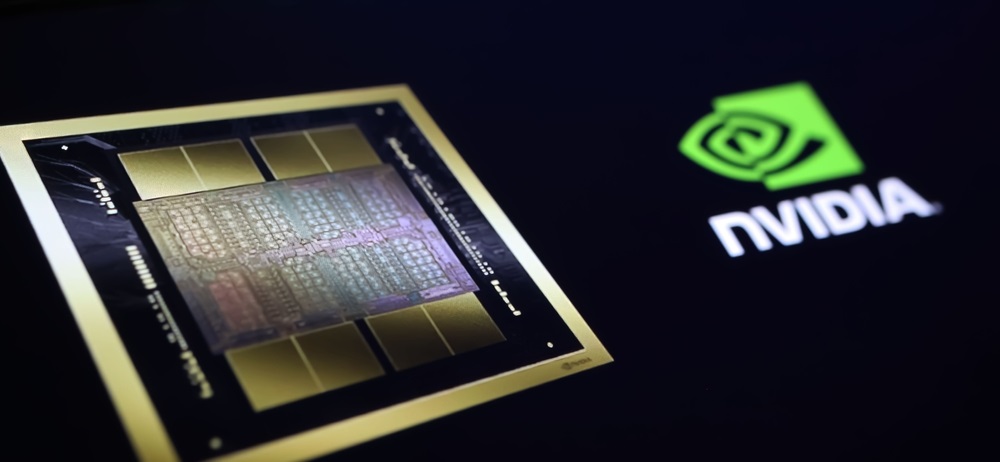
From games to AI gamesmanship
Nvidia’s ambitions used to be much smaller. Founded in 1993 by Huang and fellow entrepreneurs Curtis Priem and Chris Malachowsky, the firm was initially just intended as a means for the trio to flog graphics cards to the games industry. That changed six years later with the firm’s invention of the GPU, a chip that allowed multiple calculations to be made by a computer simultaneously instead of sequentially. It was an ideal feature for processing pixels and, as machine learning enthusiasts quickly discovered, for crafting ever more complex neural networks.
The startup soon began running with the idea. In 2006 it released its CUDA architecture to streamline the development of new AI models on its GPUs (and, critics have complained ever since, lock in customers to its chips.) Six years later, Canadian computer scientist Alex Krizhevsky brought AI developer fraternisation with Nvidia’s chips into the mainstream by creating ‘Alexnet,’ a convolutional neural network trained to recognise images on only two NVIDIA GeForce GTX 580 GPUs.
It was, explains Alex Jenkins, the biggest neural network so far and effectively created a whole new field of study in AI: deep learning. “And because they realised that they could build large-scale neural networks very early on,” says the Western Australia Data Science Innovation Hub’s director, “it resulted in their computer chips being in demand” among AI developers.
It’s a reputation that’s stuck, says Christopher Miller. “Nvidia has done more than any company to make AI possible,” argues Miller, a technology historian and the author of Chip War: The Fight for the World’s Most Critical Technology. “They are the world leaders in making it possible to train vast AI systems. Without them, the AI ecosystem would be substantially less developed.”
The advent of ChatGPT has only cemented its prestige. Since 2023, Nvidia’s sales have tripled, with tech companies regularly boasting about how many of the firm’s A100 chips power their latest LLMs. The introduction of the Rubin chip, Huang doubtless hopes, will cement Nvidia’s dominant position in the AI value chain, promising considerable cost and energy savings over and above its predecessors.
The Rubin won’t be the only AI chip in town, however. Despite Nvidia’s head start in the market, says Jenkins, “it’s unlikely that a single company is going to be able to service the requirements of the whole market.” Nvidia’s largest rival in this respect, he adds, is AMD. Founded in 1969, the latter has increased its shipments in the last year, putting it in a more fortunate position than Nvidia which, says Jenkins, is simply “struggling to meet the demand” for new AI chips.
Even so, AMD still has to overcome several challenges before it can reach level pegging with its rival, explains the analyst. “Their chips are quite capable but their driver stack is not nearly as mature as Nvidia’s,” says Jenkins. AMD also have to convince the burgeoning startup and research market for GPUs that they are a safe bet compared to their more established rival.
“If you’re an AI researcher, you don’t want to be messing around debugging – you want software that you know will work,” says Jenkins. “Even though the AMD chips are very powerful, they don’t have the software maturity that Nvidia has.”
Other rivals lurk on the sidelines. Intel, once the undisputed king of the semiconductor market, has ambitions to claw back market share with the release of its Gaudi AI accelerator – a chip that, though it commands a paltry 1% of demand, according to Bank of America analysts, the firm claims has earned it $2bn in orders.
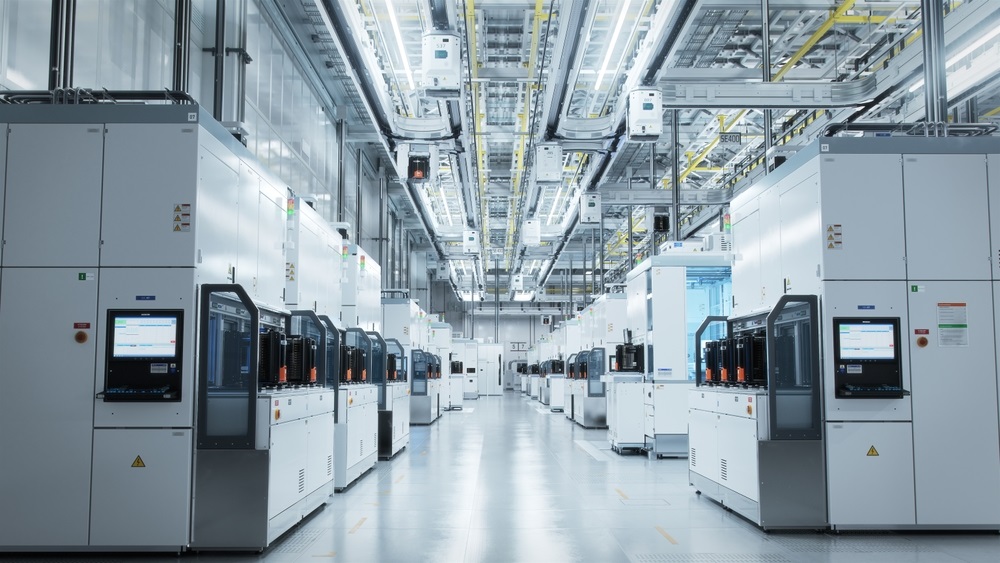
Home depots
Startups, too, are emerging to challenge Nvidia’s GPU dominance. An estimated $6bn was invested in such companies by venture capital funds last year, with firms like D-Matrix and Cerebras Systems promising leaner and meaner AI chips than those designed by the industry establishment.
It is impossible to predict which one of these upstarts, if any, might challenge Nvidia in the future. A more potent threat, indeed, may lie from the firm’s largest customers, many of which have established in-house chip laboratories to design alternatives to Nvidia’s vaunted GPU range more easily tailored to the nuances of their own software. These include Amazon, which recently developed its own AI chips Trainium and Inferentia for use in its endless AWS server farms, and Google, which recently highlighted its success in using its so-called ‘Tensor Processing Units’ to power Gemini, its flagship LLM.
There are lots of benefits to such an approach despite the initial costs, argues Jenkins. “They’re not going to be subject to as much scrutiny as they don’t have to worry about making it work for everyone,” he says. “They can develop these chips exactly for their own requirements.”
While its data centre market share is being undercut by its own clientele, Nvidia also faces some unwelcome attention from regulators. Multiple competition watchdogs in the US, UK and France have signalled their displeasure at the GPU firm’s outsized influence in the AI value chain and seem determined to clip its wings. As recently as June, news broke that the US Department of Justice was engaged in monopolistic behaviour.
“It’s not surprising that antitrust regulators are investigating Nvidia,” says Miller, though other major tech brands, he adds, are receiving just as much attention for a variety of imagined ills. And there’s no guarantee that these probes will achieve anything. “The AI landscape is changing rapidly,” says Miller. “Most regulators are only just beginning to devote time to understanding the space.”
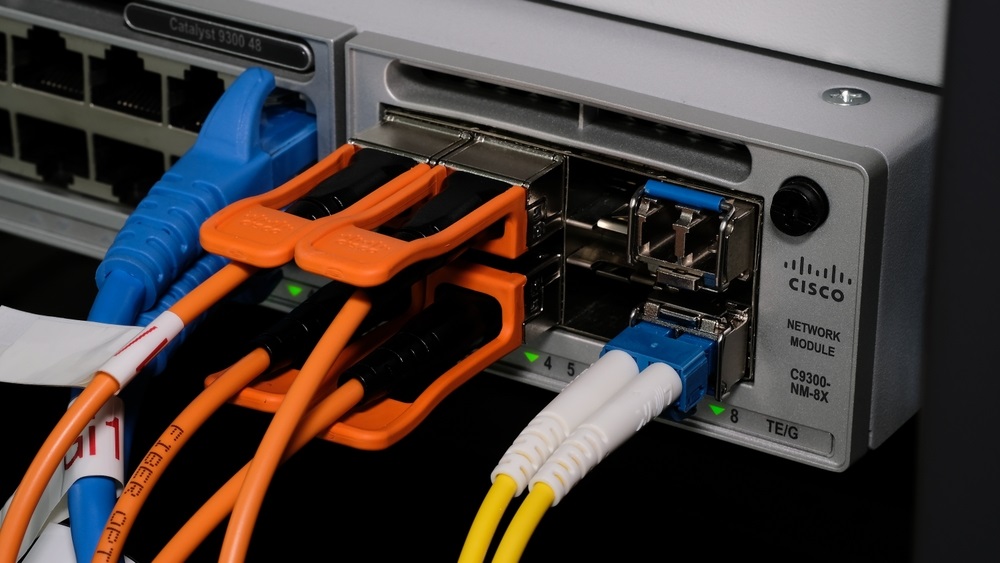
The Cisco comparison
In the end, though, the end of Nvidia may come not from the bang of a judge’s gavel or the declamations of rivals but from the quiet disinterest of a changing market. Much of the firm’s current value, after all, rests on the premise that demand for generative AI services will continue to remain strong over the next decade. That’s no sure thing, with multiple companies now beginning to question whether the returns they’re currently seeing on generative AI deployments were really worth the time and money spent on them last year.
If market sentiment toward AI finally reaches what Gartner terms the “trough of disillusionment” typical of most product life cycles, that could rebound harshly on Nvidia’s bread and butter: spending on data centre GPUs. For the firm to sustain its astronomical valuation, Futurum Group’s Daniel Newman recently told Vox, clients like Salesforce and Oracle would have to continually offer AI-powered services that effectively bind them to annual GPU contracts with Nvidia. If that doesn’t end up happening, said Newman, “that central thesis of standing up these massive megawatt data centres all over the world full of GPUs becomes a bit of a risk.”
That doesn’t preclude a more mundane future for Nvidia, say other analysts. More than a few have begun comparing the firm to Cisco. It, too, was feted as a sure-fire stock bet, selling a product deemed essential for the continued growth of a technology with boundless potential. In Cisco’s case, however, building state-of-the-art internet routers wasn’t enough to prevent its valuation from cratering when the Dotcom Bubble burst at the turn of the century.
It’s not a position taken by Cisco’s own chief executive during that period, John Chambers. “The speed of change is different, the size of the market is different,” Chambers told the Wall Street Journal in June.
For his part, Miller is optimistic that the AI boom has some time to run yet. Growth in AI-powered services “surpassed almost everyone’s expectations,” says the historian, with the “application of AI in different segments of the economy… still in its very early stages.” And for the moment, many of Nvidia’s clients seem to agree. Despite market disquiet, seemingly motivated by the firm committing the grievous sin of failing to exceed expectations rather than simply meet them, Huang’s brainchild continues to make hand over fist – $30bn in the past three months, to be exact.