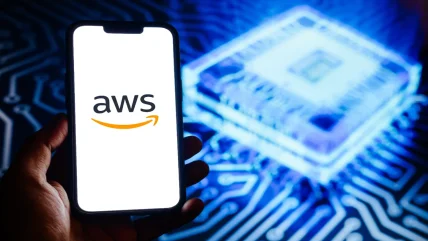
Amazon Web Services (AWS) has launched an initiative to offer free computational resources to researchers working with its proprietary artificial intelligence (AI) chips. This move by Amazon’s cloud computing division is aimed at bolstering the performance and capabilities of its Trainium chip, which serves as a direct competitor to solutions offered by Nvidia, Advanced Micro Devices (AMD), and Alphabet’s cloud division.
AWS is extending credits valued at $110m to facilitate researchers’ access to its cloud data centres for the use of Trainium. This chip, Amazon’s custom-built AI hardware, is designed to rival the leading AI chipsets currently available and to support a diverse range of AI development initiatives. Researchers from institutions such as Carnegie Mellon University and the University of California, Berkeley, are said to be leveraging these credits to advance AI model development. To further this effort, AWS is allocating 40,000 first-generation Trainium chips to support ongoing research activities.
In contrast to Nvidia’s AI chips, which are largely dependent on its proprietary Cuda software for programming, AWS plans to make the core specifications of Trainium, specifically its instruction set architecture, publicly available. This approach, claims the cloud giant, is intended to empower researchers with the ability to program the chip directly, thus bypassing intermediary specialised software layers and granting them greater autonomy in their computational pursuits.
Gadi Hutt, AWS’s business development lead for AI chips, further elaborated that AWS expects this open approach to appeal to large-scale enterprises seeking to optimise performance across extensive chip deployments. Customisation at this level could potentially generate substantial performance gains, particularly when scaling optimisations across thousands of units, thereby enhancing computational efficiency and processing throughput.
The $110m Build on Trainium initiative is focused on supporting university-led research into generative AI, as well as facilitating the creation of machine learning (ML) libraries, AI architectures, and performance optimisations. AWS will allocate substantial computational hours to researchers working on these advancements, enabling them to utilise large-scale distributed Trainium UltraClusters. These UltraClusters are composed of multiple AI accelerators operating cohesively to address complex computational challenges, thereby maximising Trainium’s potential for deep learning model training and inference tasks.
AWS Trainium offers hardware for deep learning, and Amazon plans to open-source the innovations developed under the Build on Trainium initiative to support broader research. The initiative also aims to improve AI accelerator efficiency and advance distributed systems research.
Debate over Amazon’s investment in proprietary AI hardware
To further drive innovation, AWS has committed funding for educational initiatives targeting students and for additional research projects in collaboration with leading AI research institutions. Moreover, the programme will feature multiple rounds of the Amazon Research Awards, through which selected researchers will receive AWS Trainium credits and gain access to Trainium UltraClusters to support their research efforts.
Public sentiment regarding Amazon’s decision to invest heavily in proprietary AI hardware is divided. Proponents argue that developing in-house AI chips is essential for reducing operational costs and achieving greater control over cloud infrastructure, ultimately distinguishing Amazon from its competitors while reducing reliance on external hardware suppliers. On the other hand, detractors point to the considerable financial risks, particularly given Nvidia’s entrenched dominance in the AI chip sector, which continues to be regarded as the industry standard.