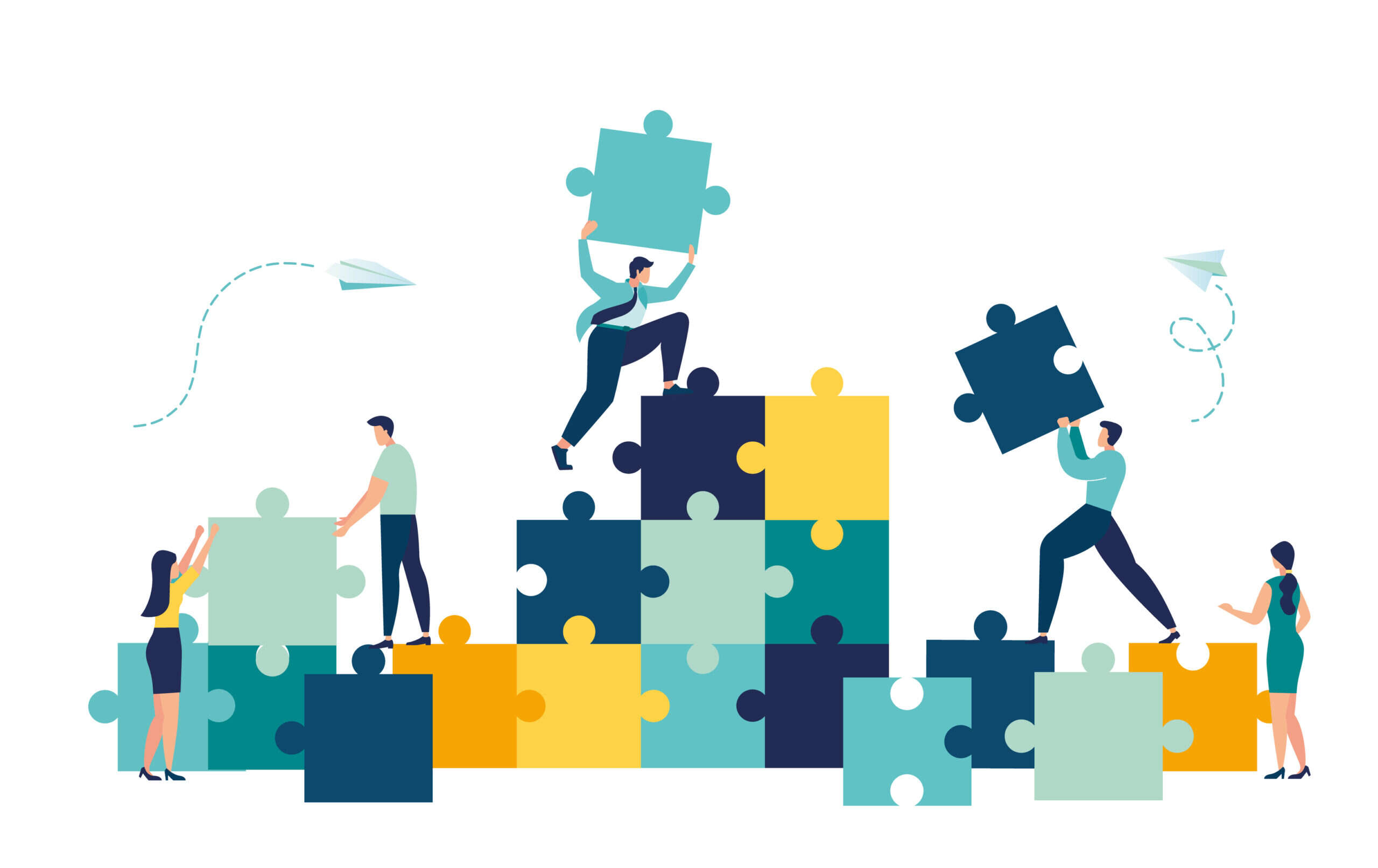
Insurers are seeking ways to better utilise their data and maximise business value by improving the accuracy and speed of their decision-making. However, as volumes of data grow within a business, so does the increasing need for frictionless and efficient access to data. Democratising data empowers people across the business to gain the necessary insights for their workflow without a heavy need for IT or data expertise.
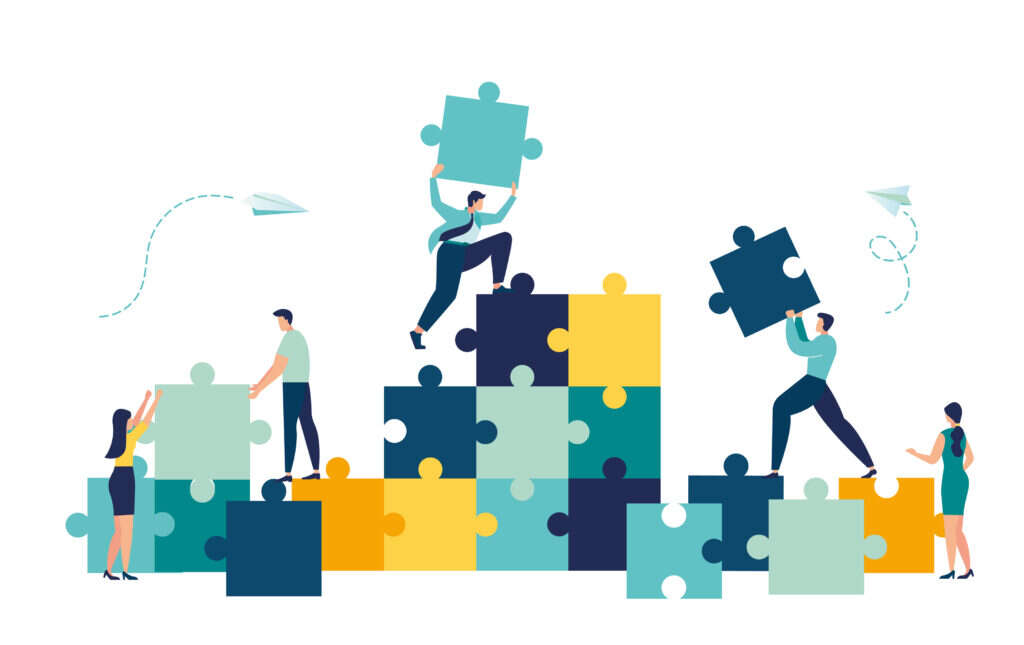
To eliminate complications within that transformation process, businesses must achieve a culture of collaboration – from how that data is accessed across a business to how outcomes and insights are derived from it. However, insurers of all sizes and tenures face challenges in aligning on these strategies, obstructing their abilities to fully harness the collective intelligence that data democratisation permits.
Convex Insurance, which reached a milestone earlier this year by surpassing $3bn in gross written premium (GWP) in less than four years, demonstrates how data smart democratisation with guardrails through platforms like Dataiku and Snowflake is a solution for businesses to get more from their data, moving beyond spreadsheets to capture enhanced value and reinforce valuable long-term processes.
Data democratisation from the ground up
“We’ve learned valuable lessons from within and outside of our industry and we’ve been able to build our Data Universe from the ground up,” says Steve Perry, head of data delivery at Convex Insurance. “We did this by utilising Snowflake to curate, conform or produce the data for business functions to consume, and now we’re looking into using that data more powerfully with Dataiku, which we’ve been using for just under two years.”
“Many organisations are hindered in their modernisation journeys by the overwhelming complexity of overlapping data assets and data pipelines, but Convex could create a single data platform to curate and house information for all of their business units and functions to use,” says Sully McConnell, head of insurance at Snowflake. “Bringing all workloads – data engineering, analytics, collaboration, AI, ML and so on – to a single copy of data helps democratise that data while simultaneously simplifying the environment.”
Convex Insurance utilised Dataiku initially within the business’s actuarial team to fulfil reserving processes, which involve evaluating, reviewing and estimating unpaid claims, in which accuracy is critical for insurers. This required considerable automation and access to data across the business, collating it in one place to analyse and produce results from the data reliably. The high level of usability and ease of the Dataiku platform proved to be successful for the actuarial team as a function.
“We say data democratisation with guardrails, but all we’re describing is the idea of development and production,” explains John McCambridge, director of FSI business solutions at Dataiku. “In this model, there’s a development space where experimentation occurs, and a production space for polished, reliable solutions. That is simply data science best practice being applied to the broader data transformation space.”
Sharing data collaboration best practices
While the initial use case for the actuarial function was a success, the longer-term challenge for Convex Insurance was looking to develop these capabilities into a robust and reliable process that the business could depend upon. From issues in versioning to building new models, many of these challenges could be reduced by sharing best practices from a data engineering standpoint, while making it palatable and reasonable for a non-data engineering or non-data science team to leverage it effectively.
“The main strength of Dataiku is that it is incredibly user-friendly. You can build data pipelines, run experiments, and so on. But there is a difference between a useful tool and something that works reliably every time that you can trust as part of your business processes,” says Perry. “We can bring our subject matter experts and skilled data engineers together to bridge that gap, play to our strengths, and work collaboratively to produce something the business can depend upon, that provides value over time.”
A collaborative approach
Convex Insurance is fulfilling this collaborative approach by hiring data engineers who are skilled in Dataiku and embedding them into business functions to work directly with subject matter expertise. Even with an effective, highly intelligent team working alongside such platforms, which allows teams to adopt new data pipelining approaches without having to become data engineers or data scientists, this does not always result in an airtight process ready to be put into production.
“I think the starting point is acknowledging that there isn’t a superior version of Excel. To automate and enhance business processes beyond Excel and reduce reliance on manual, low-value work, you need to transform the underlying processes, which unlocks new possibilities,” says Perry. “You’re not simply using a different tool, you’re creating a different process. Then, you democratise data and tooling by orienting people around the same consistent data sources to produce consistent results.”
Building trust in data
Some of the challenges in data transformation are finding the right data, using it consistently, retrieving it from the right sources, and trusting this enough to slowly remove the manual labour involved. This requires executing a fully collaborative process that utilises the same reliable data and sources to produce consistent results that bolster data quality over time.
“If you were to ask, could we eliminate the hand-cranking entirely? The answer often is yes, but not to immediate effect,” says McCambridge. “So, what do we do in the interim? Do we keep dealing with the bad process? No, you iterate, isolate that problem, solve it, and gradually improve the project.”
“The partnership model that we’re working to implement is fully collaborative,” adds Perry. “The end state of embedding resources directly within business processes is that those data workflows and projects can come back into our centre of excellence and their BAU team and be managed by the data team. This means that those business functions do not need to budget for those specialist resources in the long term.”
Data democratisation needs accountability
Traditionally, this would be harder to achieve, as technology teams would often have demands requested of them on a project in a siloed scenario. This process can be time-consuming as projects are transferred back and forth between teams based on feedback and review, and accountability becomes lost in the process.
“As organisations strive to become ‘data-driven’, it is important to consider the cultural elements required to make that journey successful, and accountability is certainly one of them,” says McConnell. “As organisations attempt to right-size and distribute accountability during these large transformation initiatives, investments in data literacy are typically one of the critical success factors.”
“One of my favourite sayings is democracy without accountability is chaos,” says Perry. “Everyone wants to have control of everything. If you’re not able to be accountable, then you won’t get to where you need to.”
“For data democratisation to work, it’s not just the responsibility of one team to make people comfortable or effective,” adds McCambridge. “Investing in the correct technology, such as Dataiku and Snowflake, helps to give the people in your organisation the best shot at success, but it must come alongside training and enablement, and a broader sense of bringing people together effectively.”
A collaborative approach means that accountable teams work together at the heart of the data project, meaning that handover and ownership when they occur are not total, as teams help to facilitate and manage tasks while remaining in connection with each other. This does not mean teams take ownership of everything entirely, but it allows workflows to be managed and fulfilled collaboratively in a partnership.
Stakeholders across teams of different responsibilities and expertise can then apply their knowledge to any process at the right step, helping insurers develop solutions around their data at a much quicker pace, from which valuable decision-making can be successfully made at speed.