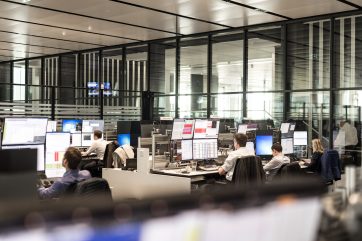
Any implementation of new technology, especially in the financial sector, must tread a careful balancing act on behalf of clients, the business and ever-watchful regulators. That’s the advice of Christoph Rabenseifner, Deutsche Bank’s strategy lead for technology, data and innovation, and it’s worth heeding. A veteran of the financial services industry, Frankfurt-based Rabenseifner has held a plethora of business and technology-oriented roles at the banking giant — including in the bank’s own one-time digital ventures entity.
With internal and external pressure to use generative AI, it’s on technology leaders such as Rabenseifner to ensure that this balance isn’t forgotten, especially when so many quick wins are apparently available for the technology’s adoptees. But with Deutsche Bank already using AI widely — from financial crime investigation to customer chatbots — Rabenseifner tells Tech Monitor about the role a confident long-term outlook has to play in his institution’s grand technology strategy, and where the CIO fits in all this. All are in the following interview, edited for clarity and length.
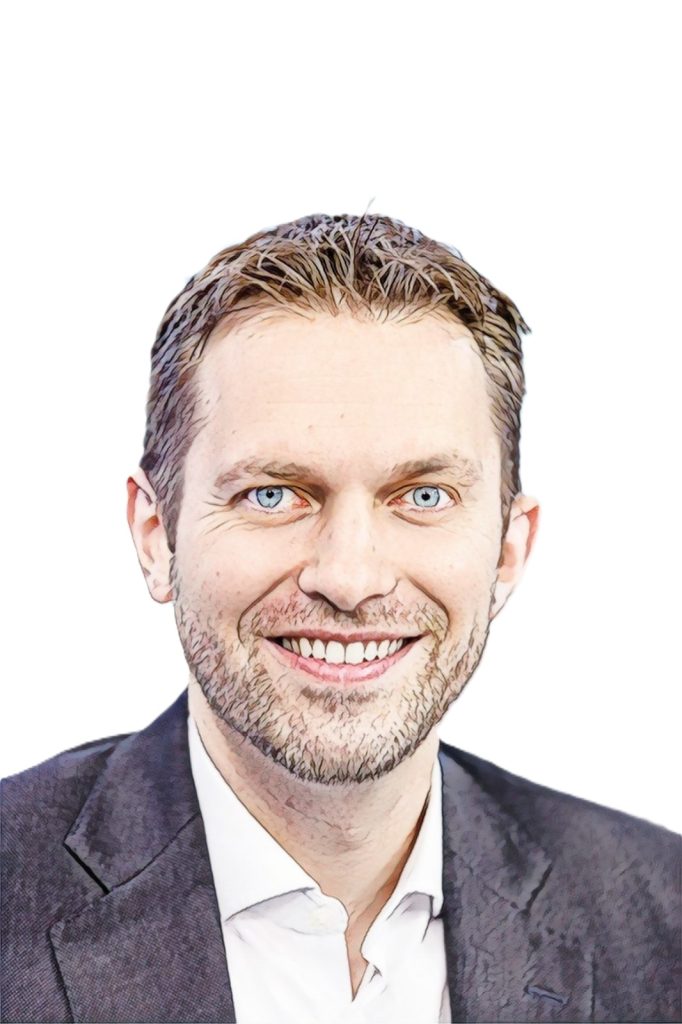
Tech Monitor: There is a lot of current hype around generative AI in the financial sector. But this is hardly banking’s first foray into the uses of such technology, is it?
Rabenseifner: The banking sector has long applied AI and machine learning to risk, trading and other processes. But generative AI, over the last few years, has created a new and tremendous momentum for the medium everywhere because it has made AI understandable for non-technologists. As a bank, we want to use that momentum. I’m 100% convinced that such tools will be an integral part of more or less every business line and product that we will create in the future – in large part thanks to the sheer amount of data we have available to train these models compared to other industries.
As far as using generative AI across the business is concerned, we’ve decided to proceed with centrally directed deployments executed by individual business units. This means the central team strategically steers AI but individual departments deliver business outcomes, benefits and productivity. And to do that successfully we need common architectures, systems, landscapes and applications.
What does this mean for the application of generative AI to business use cases? Is there flexibility in this approach?
There are some use cases which we allow to be operated by the business vertical CIOs but there are many cases where our central technology infrastructure team runs the case and we create APIs to link applications. We take a flexible approach.
Of course, this is not only because of the breadth of what we do but also because of the journey we are on. One of the earliest applications for AI at Deutsche Bank was document processing, which not only helps with internal productivity but is also easy to implement. However, we are now moving more broadly toward improving processes and introducing more sophisticated models – not something we will see imminently but with a long-term view.
Let’s focus on that long-term view: how are you ensuring that generative AI doesn’t end up being deployed as a quicker fix and has long-term strategic value?
Let’s consider the client-facing chatbot. It’s a no-brainer introduction to AI for every bank because of the potential efficiency gains: it’s more affordable than employing a human to perform the same function, frees its human counterparts to deal with more complex inquiries and likely increases the overall quality of the customer service provided. But we have to ask: is this chatbot ready to have more complex requests routed to it? Can I ask about complex transactions that would otherwise have to go through a relationship manager or investment advisor in the bank?
I think this latter application will arrive in the next few years but we have to make sure that, before we give that technology to our clients, it doesn’t cause them or their interests any harm. It is of the utmost importance that what we deliver to our customers is of the same level of quality they’ve always been used to. Some might call that approach risk-averse, but it’s one we have successfully pursued over the past three years, always focusing on internal testing before client rollout.
For example, we recently created a digital assistant to analyse annual reports of public companies and use that to provide information to our clients. But to use it effectively, we keep a human in the loop that takes that output and then delivers research to the client.
However, in a world where every bank has a digital assistant for research, there’s a danger that more or less every report will look the same. So then we have to ask ourselves what our edge can be, whether that lies in the greater reliability of the data we deliver to clients or simply that extra bit of knowledge that they simply won’t get from any other digital assistant. We also have to think defensively: do we have data access rights set correctly, for example, and have we avoided security issues? Does our data provide trust? Are we using trading data in the correct way?
How do you get to this more innovation-centric state of play?
It’s step-by-step. As you can imagine, every Deutsche Bank employee wants to use the AI tools that are available on the market — and you can boost your productivity by using them. However, these tools are like a Swiss Army knife: they can accomplish many tasks but none of them perfectly. Instead, when it came to creating our own digital AI assistant in partnership with Google, we needed a specialised approach, something that could sit on top of our applications and promptings. Again, in a manner that our non-technologist colleagues could use to generate more accurate, high-quality answers.
How important are such partnerships in manufacturing generative AI tools that truly work for you?
Tremendously important. Google is the most prominent partnership we have but all of our longstanding strategic partnerships give us early access to new tools, including generative AI applications. Every use case that we have for generative AI was kickstarted before those tools were made public, meaning our development team could experiment before those tools were finalised.
For us, in the financial sector, that’s really important. We’re so regulated that new tech applications can have a long development cycle. But front loading in the development cycle, with early access, has been so helpful – now, something that may have taken months in the beginning is now just taking weeks. For us, this isn’t so much a technology question — a developer can download an open source model and create a chatbot that could be 80% accurate easily — but about risk management, and whether we can manage that risk with early access in the development cycle. In reverse, this helps our partners create enterprise-ready products.
How do you ultimately define risk in your role?
It’s a tough balance. I’m pushing innovation; I want our colleagues to do more with generative AI. At the same time, I have to act like a control function that has to tell everyone to slow down at certain points. It’s an ongoing conversation and one that requires close contact to our actual control functions. It’s simply wrong to assume that, just because we are in a highly regulated industry like finance, we have solved every risk and control for AI.
Regulation is a good starting point for risk management but we need to have new processes to tackle the emerging challenges, too. This can be achieved by fostering a better understanding of what AI is within the bank and knowing that outputs are not always predictable. For good business outcomes, it means that everyone in the business has to have a full understanding of what AI can be used for. As a bank, we are not reactively giving access to common generative AI applications when people ask for it. I often find myself saying to those asking to use the technology in their role day-to-day, “You come to me asking for this application, but first you need to describe the problem. Then we can define which technology solution is best suited to solve it.”