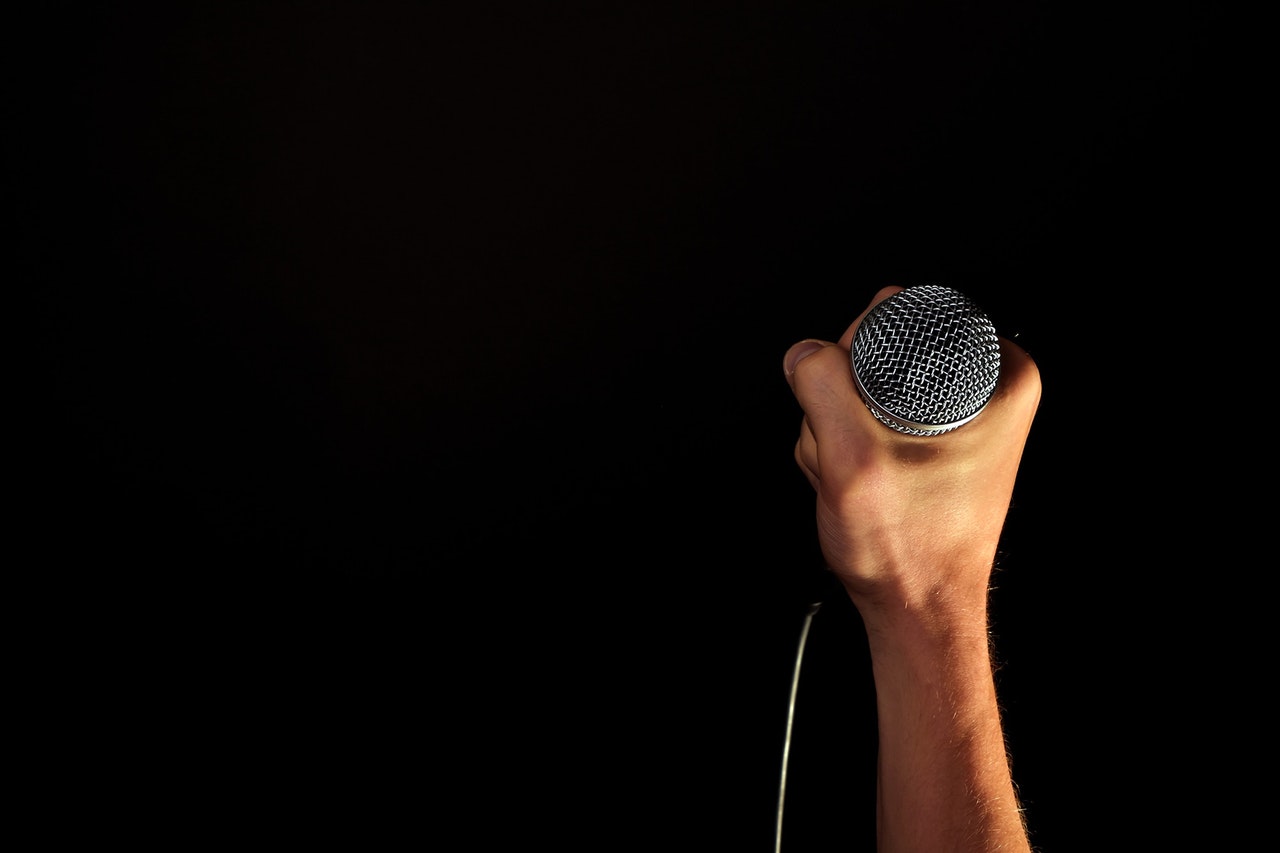
Every Monday morning we fire five questions at a C-suite technology industry interviewee. Today we’re pleased to be joined by Paperspace CEO Dillon Erb.
Biggest Challenge for your Clients?
One of the biggest challenges for our customers is bridging the disconnect between the promise of AI and the challenge of implementation.
ML and AI are extremely powerful tools, and there’s a lot of promise within certain verticals — from computer vision to driverless cars to all sorts of frontier applications. But “operationalizing” it or making it part of an existing stack is very challenging, especially for companies that might not have a lot of in-house expertise or didn’t start off as an AI-first company. PWC recently noted that “A full 20 percent of enterprise organizations plan to implement AI enterprise-wide in 2019” yet only “15 percent had appointed a single enterprise-wide AI leader.”

The reality is a lot of companies have invested in Big Data — whether it’s infrastructure or maybe even Snowflake — which means they’ve also likely invested in modern development practices for existing revenue.
But for other components, the key learning piece is still relatively new, and it’s not altogether obvious how they all integrate with existing systems.
So what you wind up having are data teams with data scientists who, on one end, have built out prediction models and are pushing the cutting edge. On the other side, you have the DevOps folks who are putting systems together and releasing them at scale.
Currently, there are technologies in the application development world like continuous deployment and continuous integration. The paradigms for these technologies that have really pushed the development forward don’t exist in the ML world today. Without these types of tools, you wind up in a position where there’s a hand-off between the ML or data science team and the DevOps or systems teams — and that’s usually filled with a good amount of friction.
Technology that Excites You Most?
In the ML space there is no shortage of amazing new applications. One of the most exciting things for me is a new area called synthetic data where you can supplement existing data with data that is created algorithmically. This is critical because many companies still struggle with complete and clean data sets. Synthetic data opens up the possibility of doing even more sophisticated ML even if you don’t have the perfect data infrastructure piece in place.
The second one, and arguably the most interesting area that excites me, is research called reinforcement learning. The idea there is — and this relates to synthetic data — you can train ML agents to operate in unknown or unfamiliar environments where the rules for those environments aren’t known. For example, in the case of industrial robotics, it’s very difficult to test out a ML algorithm on a million-dollar robotic arm. The idea is within a simulated environment (a unity or a physics engine) you can do lots of testing where you can begin to build out very powerful models and intelligent ML applications without ever having to test those in the real world.
Reinforcement learning is a technique during which you don’t know the rules within the system you’re playing in. What you need to do then is to see how many times you will fail within a digital environment simulating the real world and then, through those failures and experiments, you can begin to generate a really sophisticated understanding of the world.
Another interesting example is there are lots of companies that are investing in driverless cars. That’s a potentially problematic technology to test early on. There are companies and researchers that are trying to train ML algorithms for driverless cars inside of driving simulations like video games. What’s interesting to me is that you can test out a lot of assumptions more quickly and easily within a digital environment than you can in the real world. That also lowers the barriers to entry. I don’t think we’ve figured out yet what the ramifications are for all sorts of other domains as well.
Greatest Success?
I am most proud of the team I have built at Paperspace. I get to work with some of the most brilliant minds on some really impactful technologies. We even have our own research arm called the Advanced Technology Group that plays a big role on how we develop our own internal best practices. We’ve had people there working in everything from novel, cutting edge machinery interfaces to low-level optimization within the ML space. Currently, we have researchers that are building out new ways of interacting with a cutting edge area called neural architecture search which is based on the idea that you can use an algorithm to build out an even better neural network. It’s a blast.
We also work with some really interesting companies. For example, we provide the underlying technology for a company called Medivis that provides an AR platform for physicians to create a safer environment for surgical procedures.
And let’s not forget the community. We are in a unique position where we get to bridge the academic side of ML development with the professional community. We partner with leading organizations like fast.ai and try to play a role in educating future generations of ML developers. And that’s just scratching the surface.
Worst Failure?
I would characterize failure as opportunity cost in my case. I jumped into starting a company pretty early in my career. Looking back, I now understand there were some good lessons I could’ve picked up had I spent more time working for someone before starting the company. I am reminded of this every day because running a business is no easy feat. One day you’re two people and the next you’re scaling a large engineering team distributed across the globe. That said, I have a lot of fun along the way. I’ve surrounded myself with smart, fun people to work with and couldn’t imagine being in a better place.
In Another Life, I’d Be…
If I wasn’t building tools for ML geniuses, I’d probably go back to school and try to become one myself. The mathematicians, statisticians and researchers — the ones building software to make the world a better place. I am fascinated by the technology and the fundamentals. What can I say, I am a geek at heart.