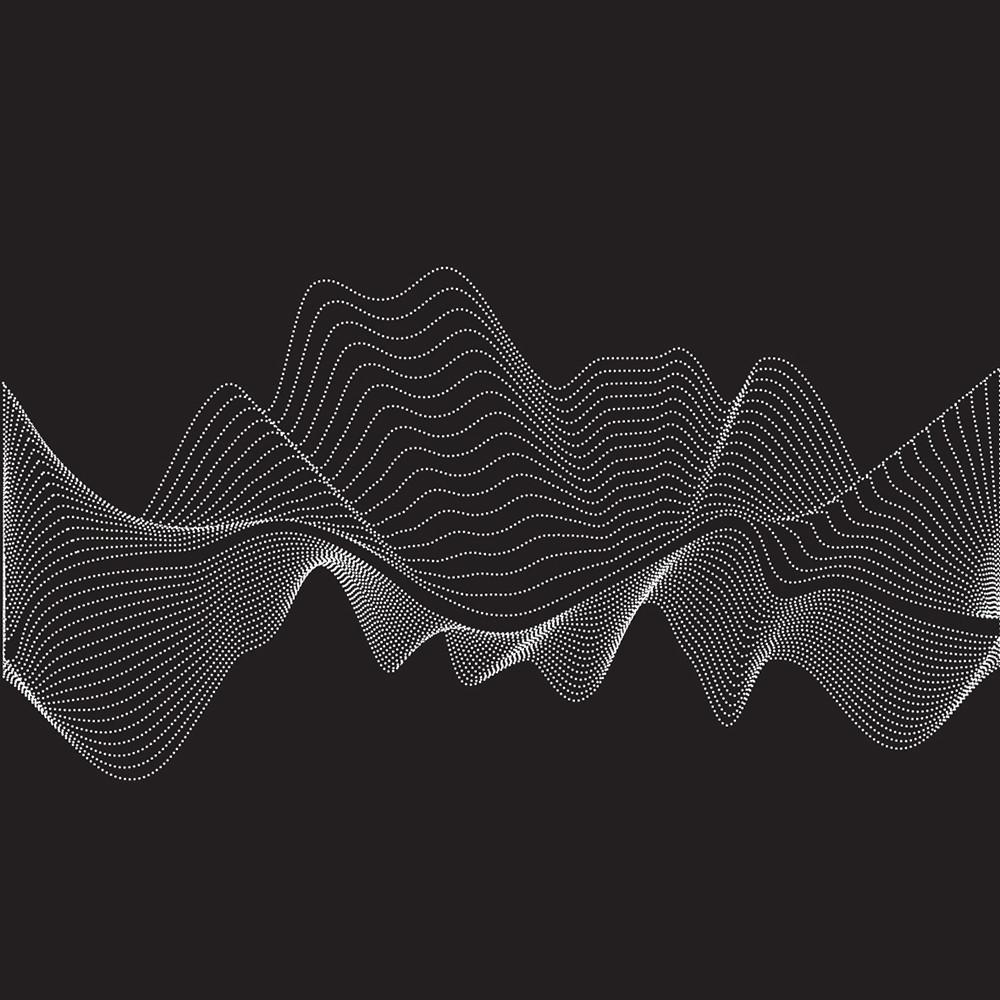
Apple’s expensive Shazam acquisition may not look quite so strategic now – despite EU regulators clearing the deal (widely reported to have been for some $400 million) earlier this month – thanks to a new sound search feature from Google.
The Shazam application allows users to identify songs through a small audio fingerprint and has some 100 million monthly active users.
The ubiquity of Google represents a serious challenge to its dominance in this market however, and with the search and advertising giant this month introducing a new “Sound Search” feature powered by some of the same deep neural net technology used in the Now Playing function on its Pixel 2 smartphone, Shazam faces an emerging heavyweight contender in the music recognition business.
Sound Search: “Hey Google, What’s This Song?”
In developing Now Playing, Google AI’s James Lyon notes in a recent blog, the company wanted to develop a music recogniser that uses a small fingerprint for each track in the database, allowing music recognition to be run entirely on-device without an internet connection.
He writes: “As it turns out, Now Playing was not only useful for an on-device music recognizer, but also greatly exceeded the accuracy and efficiency of our then-current server-side system, Sound Search, which was built before the widespread use of deep neural networks.”
With the goal of making Google’s music recognition capabilities “the best in the world” the company has now brought together the deep neural net capabilities behind its “Now Playing” feature with the server-side Sound Search.
(Users can play with the feauture through the Google Search app or the Google Assistant on any Android phone. Just start a voice query, and if there’s music playing near you, a “What’s this song?” suggestion will pop up for you to press. Otherwise, you can just ask, “Hey Google, what’s this song?”).
How Does the New Sound Search Work?
Now Playing miniaturized music recognition technology such that it was small and efficient enough to be run continuously on a mobile device without noticeable battery impact, Lyon writes.
To do this, Google used “convolution neural networks” to turn a few seconds of audio into a unique fingerprint.
This is generated by “projecting the musical features of an eight-second portion of audio into a sequence of low-dimensional embedding spaces consisting of seven two-second clips at one-second intervals”.
That fingerprint is then compared against an on-device database, which is regularly updated to add newly released tracks and remove those that are no longer popular, using a two-phase algorithm to identify matching songs: the first phase uses a fast but inaccurate algorithm which searches the whole song database to find a few likely candidates, and the second phase does a detailed analysis of each candidate to work out which song, if any, is the right one.
Quadrupled the Size of the Neural Network
James Lyons writes: “As Sound Search is a server-side system, it isn’t limited by processing and storage constraints in the same way Now Playing is. Therefore, we made two major changes to how we do fingerprinting, both of which increased accuracy at the expense of server resources:
“We quadrupled the size of the neural network used, and increased each embedding from 96 to 128 dimensions, which reduces the amount of work the neural network has to do to pack the high-dimensional input audio into a low-dimensional embedding. This is critical in improving the quality of phase two, which is very dependent on the accuracy of the raw neural network output.
“We doubled the density of our embeddings — it turns out that fingerprinting audio every 0.5s instead of every 1s doesn’t reduce the quality of the individual embeddings very much, and gives us a huge boost by doubling the number of embeddings we can use for the match.”
“We also decided to weight our index based on song popularity – in effect, for popular songs, we lower the matching threshold, and we raise it for obscure songs. Overall, this means that we can keep adding more (obscure) songs almost indefinitely to our database without slowing our recognition speed too much.”
Shazam may not be overly concerned, even if Apple is sweating a little.
The company’s R&D work in the area is extensive and when even its interns can write with this depth of knowledge, it may feel like there is room for both sound search applications in the world.