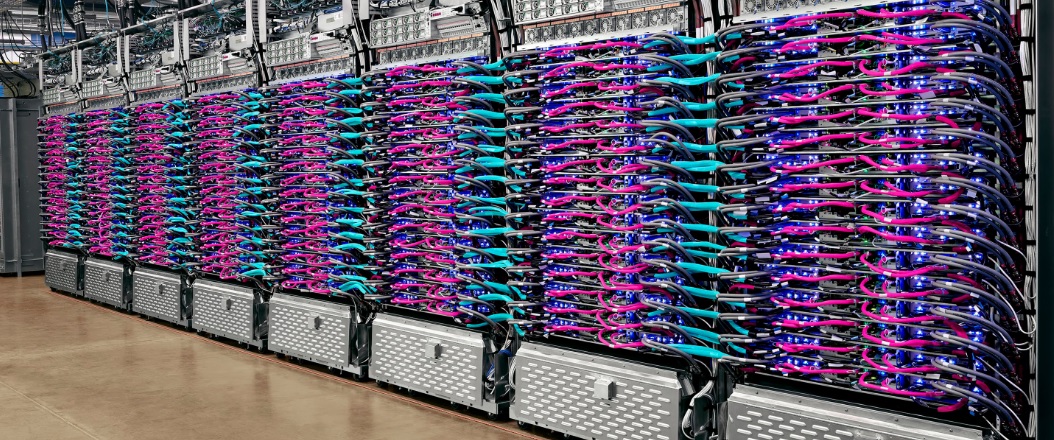
Google and Nvidia have both declared victory for their hardware in a fresh round of “MLPerf” AI inference benchmarking tests: Google for its custom Tensor Processing Unit (TPU) silicon, and Nvidia for its Turing GPUs. As always with the MLPerf results it’s challenging to declare an overall AI leader without comparing apples with oranges: Alibaba Cloud also performed blisteringly strongly in offline image classification.
The MLPerf Inference v0.5 tests were released Wednesday, and capture some of the impressive performance taking place in AI/machine learning, both at the hardware and software level. These performance improvements are rapidly filtering down to the enterprise level: as sophisticated customer service chatbots, models to predict investment outcomes, to underpin nuclear safety, or discover new cures for disease.
Read this: UK’s Atomic Weapons Establishment Buys 7 Petaflop Cray Supercomputer
The MLPerf tests are designed to make it easier for companies to determine the right systems for them, as machine learning silicon, software frameworks and libraries proliferate. (As an MLPerf whitepaper puts it: “The myriad combinations of ML hardware and ML software make assessing ML-system performance in an architecture-neutral, representative, and reproducible manner challenging…”)
MLPerf itself is also evolving fast: future versions will include new benchmarks like speech-to-text, and additional metrics such as power consumption.
New Smartphone App for AI Inference Benchmarks
The MLPerf Inference v0.5 tests contain five benchmarks that focus on three machine learning tasks: object detection, machine translation, and image classification.
There are two divisions, open or closed, the closed division requires each company to use the same model and reference implementation, so that hardware and software capabilities can be compared. To ‘foster innovation’ the open divisions lets companies use different models and training; apples, oranges, and passion fruit, as it were.
MLPerf is also aiming to make it easier to participate.
“MLPerf is also developing a smartphone app that runs inference benchmarks for use with future versions. We are actively soliciting help from all our members and the broader community to make MLPerf better,” said Vijay Janapa Reddi, Associate Professor, Harvard University, and MLPerf Inference Co-chair.
Nvidia Performs Strongly
Nvidia came out on top in data-centre focused scenarios, as its Turing GPUs provided the highest performance per processor when it came to commercially available products. Nvidia’s Turing beat out Intel’s Xeon P9282 and Google’s TPUv3 in both offline and server scenarios. In offline scenarios AI’s are given tasks like photo tagging, while in the server scenarios the tasks reflect work like online translation services.
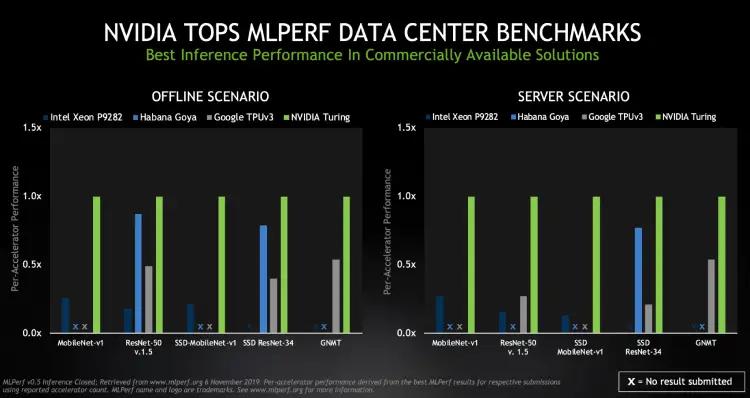
Nvidia took home the honours for Edge system on-a-chip benchmarks, but as with many of the MLPerf tests it wasn’t a full competitive field; only Qualcomm and Intel submitted results for the category alongside Nvidia. Nevertheless, in single and multi-stream scenarios Nvidia was head and shoulders above both competitors. (A single-stream task is the equivalent of a camera assessing tin cans on a production line, while mulit-stream tests how many individual feeds a chip can handle.)
Ian Buck, Nvidia’s VP of accelerated computing said: “AI is at a tipping point as it moves swiftly from research to large-scale deployment for real applications.
“AI inference is a tremendous computational challenge.
“Combining the industry’s most advanced programmable accelerator, the CUDA-X suite of AI algorithms and our deep expertise in AI computing, Nvidia can help data centers deploy their large and growing body of complex AI models.”
Google’s TPU v3 Still Image Classification King
With the ResNet-50 v1.5 image detection test we can see that Google’s TPU v3 suffered a significant drop when it moved from a Server to an Offline test, dropping from a Server time of 16,014.29ms to an Offline of 32,716.00ms. Yet image classification is a category in which Google is the undisputed winner at this point in time: its Cloud TPU closed division offline results for ResNet-50 v1.5 (image classification) show that just 32 Cloud TPU v3 devices are able to process more than a million images per second.
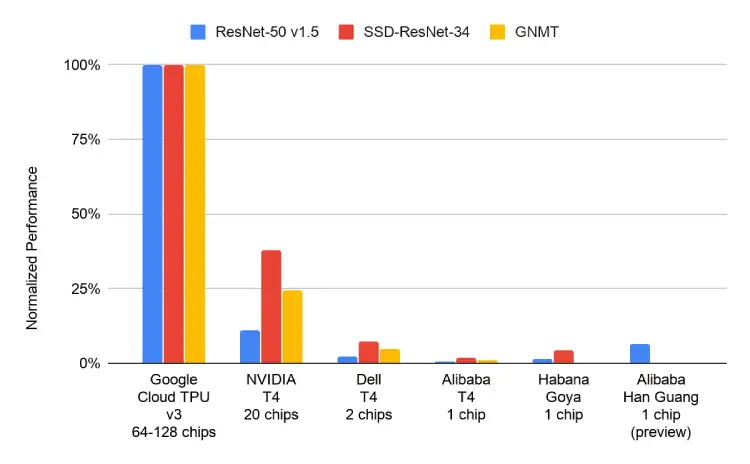
Commenting on that result in particular Pankaj Kanwar Google’s technical program manager for TPU stated that: “To understand that scale and speed, if all 7.7 billion people on Earth uploaded a single photo, you could classify this entire global photo collection in under 2.5 hours and do so for less than $600.”
Alibaba, Centaur Technology, Dell EMC, dividiti, FuriosaAI, Google, Habana Labs, Hailo, Inspur, Intel, NVIDIA, Polytechnic University of Milan, Qualcomm, and Tencent were among those participating in the latest round of AI Inference tests.
A full look at this round of MLPerf results can be viewed here.