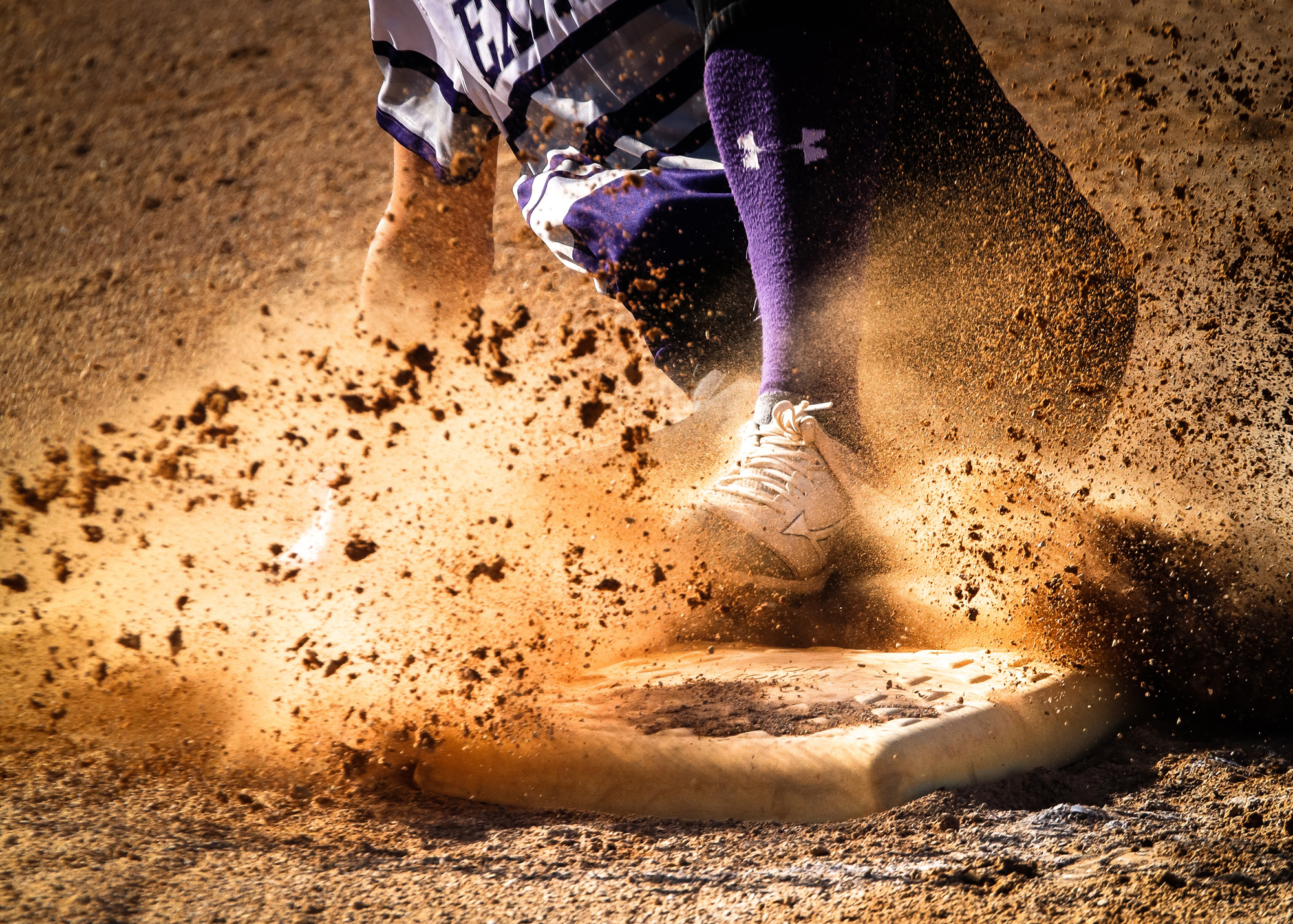
In industry-backed standardised tests Nvidia has smashed AI training records, while Google Cloud has become the first company to be faster than on-premise systems at large-scale, industry-standard ML training workloads, winning in three categories
In the latest round of the MLPerf test for AI training, Nvidia set eight records in AI training performance.
Nvidia were the only company to submit entries into all six categories in the tests using its DGX SuperPOD, a set-up that is powered by 1,536 NVIDIA V100 Tensor Core GPUs interconnected with NVIDIA NVSwitch and Mellanox network fabric.
The six categories tested in this round were: image classification; object detection light-weight; object detection heavy-weight; translation, recurrent; translation non-recurrent; recommendation and reinforcement learning.
In these ‘closed division’ tests everyone must use the same model and optimizer. As well as build restrictions, the model for the image classification is set to ResNet-50 v1.5. These specification requirements allow for comparisons between the tech.
When Nvidia set its DGX-1 server to the task of training an image classification model during the last tests seven months ago, it took eight hours. This week using a DGX SuperPod with the MLPerf set ResNet-50 training model for image classification Nvidia smashed this time down to 80 seconds.
Nvidia have been working to increase the performance of its hardware with monthly software upgrades and tweaks, something that appears to have paid off as its NVIDIA DGX-2H server saw increases of up to 80 percent in comparison to MLPerf submissions last December.
Dave Salvator Senior Product Marketing Manager at Nvidia outlined in a developer blog some of the key software upgrades that helped to reduce the training time: “A variety of DALI-related improvements accelerated the data input pipeline, enabling it to keep up with high-speed neural network processing. These include using NVJPEG and ROI JPEG decode to limit the JPEG decode work to the region of the raw image actually used.”
“We also used Horovod for data parallel execution, allowing us to hide the exchange of gradients between GPUs behind other back-propagation work happening on the GPU. The net result weighted in as a 5.1x improvement at scale versus our submission for MLPerf v0.5, completing this v0.6 version training run in just 80 seconds on the DGX SuperPOD.”
Google Cloud
Google Cloud entered into five categories and managed to set three records for performance at scale using its Cloud TPU v3 Pods, which are essentially racks of Google’s Tensor Processing Units (TPUs) 1
Notably its Cloud TPU v3 Pods trained models 84 percent faster than on-premise systems in three categories. Its highest achievements were in the Transformer model architecture and SSD model architecture.
Zak Stone Senior Product Manager for Cloud TPUs commented in a blog that: “With these latest MLPerf benchmark results, Google Cloud is the first public cloud provider to outperform on-premise systems when running large-scale, industry-standard ML training workloads of Transformer, Single Shot Detector (SSD), and ResNet-50.”
“The Transformer model architecture is at the core of modern natural language processing (NLP)—for example, Transformer has enabled major improvements in machine translation, language modeling, and high-quality text generation.”
MLPerf AI Records
The MLPerf, initiated in May 2018, is a collaboration of engineers and researchers working to build a new industry benchmark. The MLPerf benchmark is supported by a wide consortium of technology leaders such as Google, Intel, NVIDIA, AMD and Qualcomm.
It was launched as the pace at which machine learning and AI has been moving in recent years has made it difficult to get an accurate measurement of a company’s capabilities. This is compounded by the fact ML and AI can be sprawling terms that encompass a range of techniques making it hard to compare efforts in the field.
According to its mission statement: “The MLPerf effort aims to build a common set of benchmarks that enables the machine learning (ML) field to measure system performance for both training and inference from mobile devices to cloud services.”
MLPerf is support by over 70 organisations and this round of tests saw five companies submit to testing Nvidia, Intel, Goolge, Alibaba and Fujitsu.