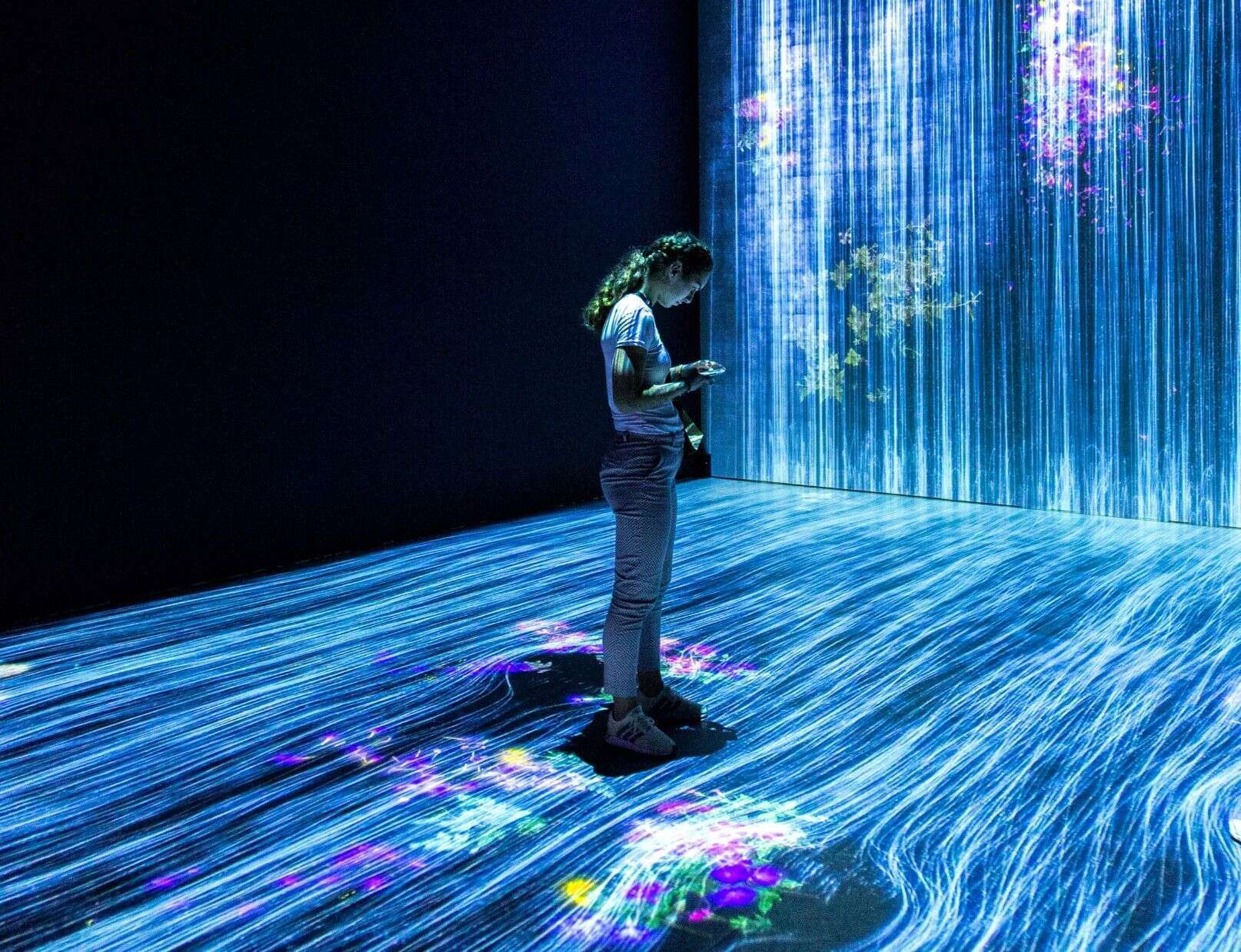
From accounts to recruitment, drug discovery to financial products, AI implementation offers the promise to business leaders of automated decisions, innovative products and reduced OpEx through efficiency gains.
The reality can be somewhat different. So where does the gulf between expectation and reality emerge? A new report from the Oxford Internet Institute (OII) published this month looks closely at why AI projects often fail.
The report, AI @ Work, analyses themes in 400 reports about AI from January 2019-May 2020, focusing on how they covered AI in workplaces.
“A Significant Evidence Gap”
The authors say they discovered a significant “evidence gap in how AI tools used and how people talk about what they are supposed to do.”
As Co-author Professor Gina Neff puts it: “Time and again, we see organisations making the same mistakes in the integration of AI into their decision-making: Over-reliance on the tech, poor integration into the larger data ecosystems, and lack of transparency about how decisions are made… the one takeaway that rings loud today is that AI systems often make binary choices in complex decision environments.”
As she told Computer Business Review: “As AI moves from the technology sector to more areas of our economy, it is time to take stock critically and comprehensively of its impact on workplaces and workers.
“The aim of this report is to inform a more comprehensive dialogue around the use of AI as more workplaces roll out new kinds of AI-enabled systems by looking at the challenges of integrating new systems into existing workplaces.”
The OII report identifies three broad themes as to why AI fails workers and workplaces. Here we take a detailed look at each one.
1) AI Implementation: The Integration Problem

Gina explains that problems often begin when the cost and time of AI implementation start to unexpectedly mount up.
“We found lots of stories about the frustration of projects that take so many more resources than anyone ever anticipated,” she says.
“Another issue is that AI is often sold as something that will scale very quickly and that can move from one kind of analysis to another or from one part of a company or an organisation to another. A lot of these integration challenges are about trying to get a product that works well for one part of the organisation to work well somewhere else.”
Peter Whale is a former director of product management at Qualcomm who has spent much of his career working with AI. He now heads up the AI special interest group for tech membership organisation CW, and says quality of data is often something which hinders successful integration.
“Algorithms have got a bit better in recent years, but actually the biggest change is the fact we have a lot more data that really powers AI,” he says.
“The conversation you have with the business in terms of what a successful integration of an AI system looks should be around the quality of data available, not the quantity.
He adds: “If you want an AI system to make a decision between A or B, and in your organization you have a fuzzy definition of what A and B are, then you find people use different criteria for making decisions. So that’s where the business process piece comes in and you have to be clear about how you’re collecting your data and how you interpret it.”
2) AI Implementation: The People Problem
The OII report identifies an over-reliance on AI as another key factor in the failure of projects, and Gina says this can lead to staff becoming frustrated.
“Several of the pieces that we pull out in the report describe projects where the people working in the organisation simply come not to trust the outputs of the AI system,” she says. “That ends up costing businesses time and money.”
“There’s a lot of work to be done in the AI skills gap, not necessarily in preparing the workforce to be able to design and implement AI projects, but more importantly on the ground. Companies need to ready the their staff to work with AI systems, to be able to be critical and really push back if they see problems or challenges with the outputs.”

Bill Mitchell, head of policy at the British Computer Society, the UK’s charted institute for IT. Though a computer scientist himself, he is well aware that organisations need other skill-sets to achieve successful AI implementation.
“You do need some data scientists, but the clever people who come up with the clever ideas are not going to be the ones who implement these systems; they’re not the engineers or the managers,” he explains.
“It’s about having teams who can do all these things together, so you’re going have to up-skill some of your existing staff or it just won’t work.”
Bill recommends companies consider putting staff through apprenticeships such as the AI Data Specialist course launched last year.
He says: “It makes sense to invest in more apprentices around data analysis, business information systems and business analysis too, because those are also the kind of people are going to make sure you’ll manage these systems and adopt them properly.”
3) AI Implementation: The Transparency Problem
“Companies need to know where their data are being processed, what’s happening to that data, which has often been entrusted to them by customers, and who is involved in the work,” Gina says. “For many businesses, these are mission critical questions that too rarely get asked.”

Wael Elrifai is VP for solution engineering at Hitachi Vantara, which provides a wide range of IT solutions to customers around the world. His department develops new AI and machine learning products for clients.
“People think AI is magic,” he says.
“They think data goes in and answers come out, that just not the case.”
Transparency is a major problem across many branches of machine learning.
Wael believes more needs to be done to explain to customers why algorithms come to certain decisions, to improve trust and enable successful AI implementation.
“On transparency I would take a slightly different tack to the Oxford study,” he says. “What I’m interested in is why did the computer make the decision it did? Why did it decide to give this person an extended jail sentence or deny that person credit? That’s a big issue right off the bat, because I see companies not understanding that some systems are going to lack transparency, especially those based on deep learning.
“The issue with deep learning in particular is that it’s not using discrete variables that mean anything to us. So when we peer inside it, we actually can’t tell why it made such a decision. There’s a lot of research going on into making that less opaque, which will help.”
Looking to the future, Wael believes companies need to have serious discussions around their values before deploying AI in their business.
“Human beings are really bad at communicating what we want,” he says. “This matters for basic AI, and more so as we move towards advanced general intelligence (AGI). Our language is imperfect, and robots don’t understand that. So for example, if I ask a machine to find a cure for Covid-19, it will want to run a lot of experiments, which might mean infecting half the people on the planet.
“This will be a big problem when it comes to AGI, but it’s also a problem for business people now dealing with data scientists and trying to specify what they want. Context matters and value alignment matters.”
You can read the full OII report here [pdf]
Now Read This: The $300 ‘Degree’ from Google Divides the Tech World