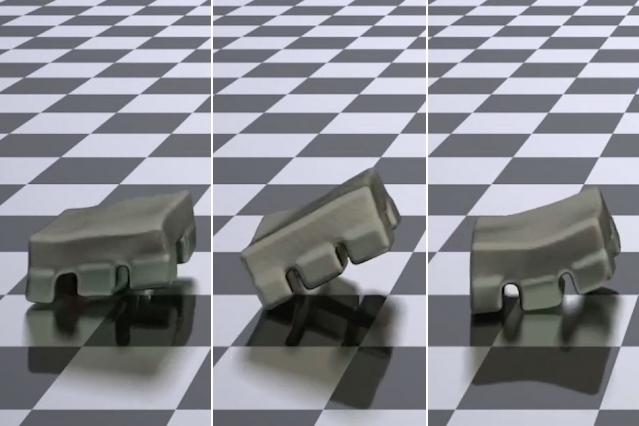
At the Conference on Neural Information Processing Systems in Vancouver next month MIT researchers will detail a soft robots control and design training simulation that beats traditional methods which can require 30,000 simulations, while the new approach needs only 400 simulations.
The field of soft robotics is somewhat new, but it could be instrumental in areas such as surgery where nano-scale robots are tasked with moving through our bodies internally without damaging soft tissue. Soft robots are typically made of elastic, highly pliable or even organic materials that open up opportunities for their deployment in roles for which conventional rigid robots are not viable.
They will also most likely become critical components in manufacturing and warehouse environments, with their flexibility.
The researchers at MIT have created a way to optimize the control and design of soft robots so that they can fulfil tasks that have proven difficult to complete in the past due to their heavy reliance on computational power.
Given that soft robots are extremely flexible and can stretch and manipulate their shapes, from a computational point of view this means that they have an infinite amount of ways to move; taxing for computers to programme.
Andrew Spielberg, first of author of the soft robot research and graduate at the Computer Science and Artificial Intelligence Laboratory (CSAIL) commented in a blog: “Soft robots are infinite-dimensional creatures that bend in a billion different ways at any given moment, but in truth, there are natural ways soft objects are likely to bend. We find the natural states of soft robots can be described very compactly in a low-dimensional description. We optimize control and design of soft robots by learning a good description of the likely states.”
MIT Soft Robotics Learning-in-the-Loop Optimisation
In order to train and develop applications for their soft robots the researchers created a simulation that trained 2D and 3D soft robotics to complete tasks like reaching an object and manipulating their form.
In the simulation a programme known as the ‘observer’ computes all the variables and oversees how well a soft robotic is completing its assigned task. Previously researchers had designed clusters of particles that held information that detailed the movement the robot could make, if the robot tried to move past those limitations it was overruled by the actuators resisting the movement. Yet manually setting all of these rules by hand is incredibly time consuming.
To get around this the researchers created a “learning-in-the-loop optimization” in which all parameters are learned over a single feedback loop within multiple simulations.
MIT notes: “The model employs a technique called a material point method (MPM), which simulates the behavior of particles of continuum materials, such as foams and liquids, surrounded by a background grid. In doing so, it captures the particles of the robot and its observable environment into pixels or 3D pixels, known as voxels, without the need of any additional computation.”
All of the information from the simulation is processed by machine learning which then creates a compressed low-dimensional representation of the particle grid that can then be used to serve as robot state representations. These can then be used by a controller to adjust the optimal stiffness of each particle optimising the robot’s movement. In future soft robots could be 3D printed with varying levels of stiffness designed for specific tasks.