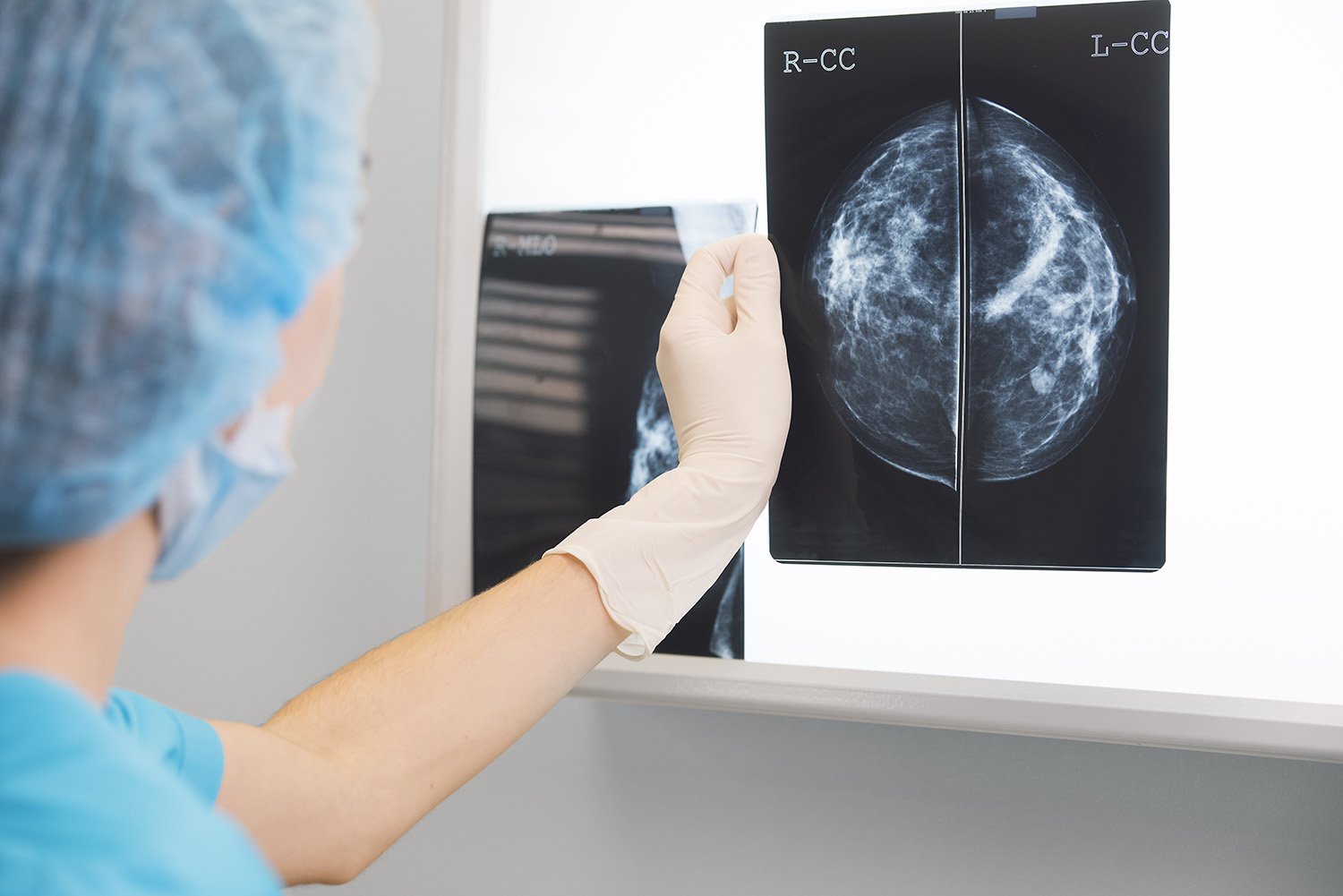
On the surface, an artificial intelligence (AI) that can detect breast cancer from a mammogram scan image more successfully than a human radiologist seems like a game-changing use of technology.
This is what Google Health proudly announced in January, publishing a paper in the Nature journal stating that its algorithm was more successful than six radiologists at identifying cancer from a large dataset of breast scans.
Screening for breast cancer is the first line of defence against the disease and, for many women, early diagnosis can literally be a matter of life or death. Interpreting the scans is a laborious and tricky task for radiologists, as the cancer is often hidden under thick breast tissue. So the idea that an AI could share or one day take over this workload is an alluring one, which caught the attention of the medical research community.

“When this Google AI paper got published I was very excited,” says Dr Benjamin Haibe-Kains, a senior scientist at Toronto’s Princess Margaret Cancer Centre, one of the world’s largest cancer research centres, and associate professor in the Medical Biophysics department of the University of Toronto.
“I do a lot of analysis of radiological images and work with a lot of radiologists, and we all understand that the current way of doing things is super-tedious and we need to do better.”
The only problem was that when Dr Haibe-Kains went to look for the code and the model behind the algorithm, he could not find any details in the paper. This led to him and 19 colleagues affiliated to leading institutions such as McGill University, the City University of New York (CUNY), Harvard and Stanford to publish a rebuttal in Nature this month. The group contends that the absence of information about how Google Health’s AI works “undermines its scientific value”, restricting the ability to carry out peer review or spot potential issues with its methodology.
The ensuing debate goes to the heart of an issue affecting many of the AI systems which are playing an increasingly important role for businesses around the world; how much AI transparency should we expect, and how do we ensure developers lift the lid on how their systems operate?
No code, no model, no transparency?
Dr Haibes-Kains and his colleagues were prompted to act because they felt the Google Health research did not meet the usual standards for a scientific research paper. “I read the Google AI paper, got very excited, read the method section and thought ‘okay, let’s do something with this’,” he says.
“Then I looked for the code, there was none to be seen. I looked for the model, there was none to be seen. They are publishing in a scientific journal, so it is Google’s responsibility to share enough information so that the field can build upon their work.

“For us Nature is the holy grail, the best of the best, and if we want to publish in it we will be asked to share all our code, all our data and everything else. It seems that for Google that was not the case, which came as a surprise.
“As researchers we love this technology and want it to work, but with no way for us to test a model in our own institutions, we have no way for us to scrutinise the code and learn from it.”
He adds: “I’ve got into many heated discussions about this, and a lot of people will say it’s a great technology and we should trust the results. My response to them would be to ask how does this change the way you would treat patients? How does it help you in any way? It doesn’t because without the code you can’t recreate it. Even if you tried, using the information available, it would take at least six months and you still couldn’t be sure you had a result which was close to their model, because there’s no clear reference available.”
Software as a medical device
In response, the authors of Google’s initial paper say they have put enough information out there that “investigators proficient in deep learning should be able to learn from and expand upon our approach”.
They add: “It is important to note that regulators commonly classify technologies such as the one proposed here as ‘medical device software’ or ‘software as a medical device’. Unfortunately, the release of any medical device without appropriate regulatory oversight could lead to its misuse.
“As such, doing so would overlook material ethical concerns. Because liability issues surrounding artificial intelligence in healthcare remain unresolved, providing unrestricted access to such technologies may place patients, providers, and developers at risk.”
Google Health adds that commercial and security considerations are also important. “In addition, the development of impactful medical technologies must remain a sustainable venture to promote a vibrant ecosystem that supports future innovation. Parallels to hardware medical devices and pharmaceuticals may be useful to consider in this regard. Finally, increasing evidence suggests that a model’s learned parameters may inadvertently expose properties of its training set to attack.”
Commercial concerns a barrier to transparency?
Tensions between academic and commercial interests are nothing new of course.

“[Google’s] view is it’s not just the algorithm,” says Wael Elrifai, VP for solution engineering at Hitachi Vantara, whose team designs and builds AI systems for customers around the world. “They trained this particular one on more than nine million images, which probably cost many millions of dollars in computer time alone, not to mention the feature engineering and the testing and all these different things, This was a multi-million dollar effort, and they’re going to want some of that money back.
“There will also be security concerns about people getting hold of this code and changing it, because it’s sometimes the case with deep learning that very small changes can make a big difference – on self-driving cars we’ve seen stories where you make changes of one or two pixels on a stop sign, a change which is invisible to a human being and the algorithm interprets it as a 45mph sign instead. Elements of these AI systems are brittle.”
He continues: “In general, I’m with the academics on this one, because I’ve never heard of a scientific paper that doesn’t give you enough information to reproduce the results. That’s the fundamental idea behind any scientific paper and without that it’s just marketing.”
Does AI transparency keep customers happy?
Though companies such as Google may be reticent to fully show their working, customer demand may drive them to be more open.
Mohamed Elmasry has been working in AI and the development of commercial technologies for 16 years, and is now CEO of Tactful AI, a UK start-up which uses machine learning to augment the customer experience. Among other things, its system listens in on calls between businesses and their clients and automatically provides the call handler with information relating to what is being discussed.
He says: “In our conversations with customers, we’re starting to hear more interesting questions like where are you going to get your data from? How can you use data we already have? How long does it take to get the models to a state that I can use? What’s the cycle of improving the training?'”
“All of these type of questions have started being asked in the last few months and we’ve never seen that before,” Elmasry explains. “People are becoming more educated, not only because the level of adoption of AI has increased, but also because of the huge digital transformation we see everywhere.”
Elmasry says Google’s approach may reflect attitudes in AI development that have prevailed since the technology started to gain momentum.
“At the start of the age of AI, providers would talk about how we could help businesses and so on,” he says. “Back then it was a little difficult to explain to them the kind of transformation they needed to do at operational level in order to get the results we were actually quoting in our marketing materials and research.
“So I think most of the providers got into the habit of neglecting the technical information and focusing on what we had achieved instead.
“The problem with that is that when you get into the sales process with your customers, you face the challenge of helping them understand that AI is a very nice tool that can give them lots of good results, but equally they need to invest in changing how they operate, and spend time on data, training and labelling. You meet some big businesses and they expect that AI is just going to work straight out of the box without training it on their exact use case.
“Here maybe Google is just trying to say we have a really promising future and we are going to achieve this and this, but decided not to get into the details of how everything happens because they felt they were addressing customers more than the research community.”
Can AI be truly transparent?
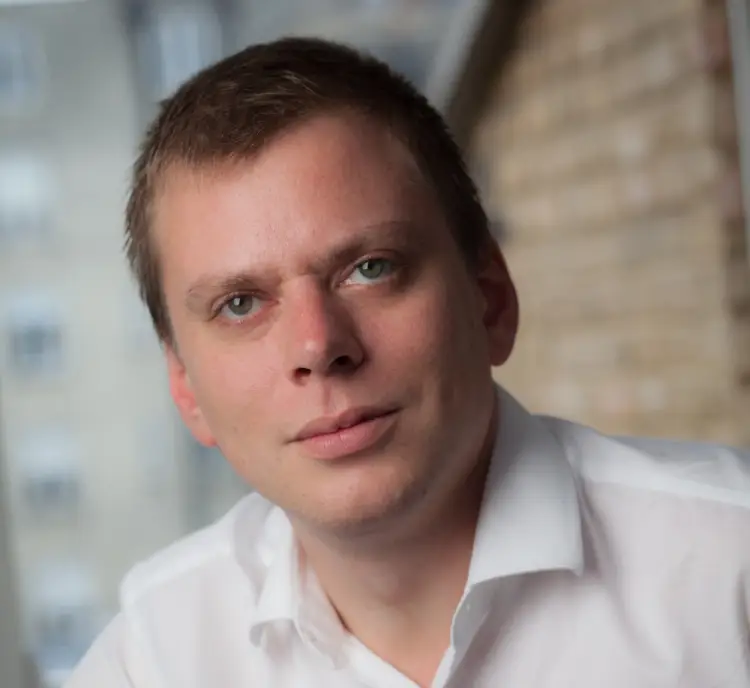
Adam Leon Smith is CTO of AI consultancy Dragonfly and editor of an ISO/IEC technical report under development on bias in AI systems. He told Tech Monitor that it is difficult to make deep learning AI systems (also known as deep neural networks), such as the Google Health AI, truly transparent.
“There’s an aspect of transparency that’s more dynamic, often called explainability,” he says. “That is knowing why an AI did what it just did, which is an unsolved problem with many deep neural networks.”
Leon Smith says this means there are always opaque parts of such systems, and does not expect a solution to appear any time soon.
“The problem is that the internal features space of a neural network is so different to how you would describe the business problem or the real-life problem it is addressing,” he adds. “It’s very, very esoteric.
“There are mechanisms that you can use to try and reverse engineer it and come up with statistical correlations and try and come up with a generalised relationship between certain inputs and outputs. I think the problem will ultimately be solvable, but it isn’t now.”
The future of AI transparency: standards and regulation?
In their paper, Dr Haibe-Kains and his colleagues call for greater independent scrutiny of models presented in research papers, particularly those pertaining to healthcare. They propose a mechanism through which independent investigators are given access to the data and can verify the model’s analysis, as has been used in other areas.
“Ultimately I think we should just be much more upfront and transparent about what a paper is about,” Dr Haibe-Kains says. “If the paper is about research, then there is no excuse [to not be transparent]. You have all those technologies that makes it super-easy to share the code, the models and even the data to a certain extent.
“If you’re in industry, you have a great product, and you want to tell the world, including the scientific community, how great it is, then just be up-front about it. Then it’s not about research, it’s about evaluation of a technology and you can say we are not going to disclose the full details because that’s not what is expected.”
Leon Smith believes greater standardisation is required to enable relevant parts of AI algorithms to be scrutinised by the companies investing in them as part of digital transformation projects.
He says: “When I talk to C-Suite people at companies who are selling AI products, they are bombarded with questions from clients who are trying to get their arms around this topic, and haven’t got a standard way to address it. So if they’re producing something where there’s a legal risk, such as under data protection legislation, they would benefit significantly from having one single checklist of things that they have to go through so they know which data they have to provide to their clients.
“I think the good players in the industry are certainly interested in this sort of thing. If you look at how we do it in areas like cybersecurity, we have established frameworks, procedures and standards that you will insist companies in your supply chain comply with. And you have independent auditors that will attest that a company is meeting those standards. I think that’s realistically the only way to go in terms of enforcing things in AI.”
Elrifai is in favour of greater regulation around the development of AI algorithms so that increased transparency would become a requirement. “The bottom line is we all subsidise Google very heavily one way or another so they do have certain responsibilities,” he says.
“We wouldn’t accept it if an arms manufacturer was assembling nuclear weapons and one of them blew up every six months. I think they need some guard rails in the way they operate, and it’s not crazy to heavily regulate some industries.
“In the UK, you have to offer the lowest price you can when selling to the NHS because you have a public rule that says health is special and has to be considered separately from the rest of the market. I think in areas like AI it’s okay to tell companies, such as Google, that they cannot just go in and maximise income.”