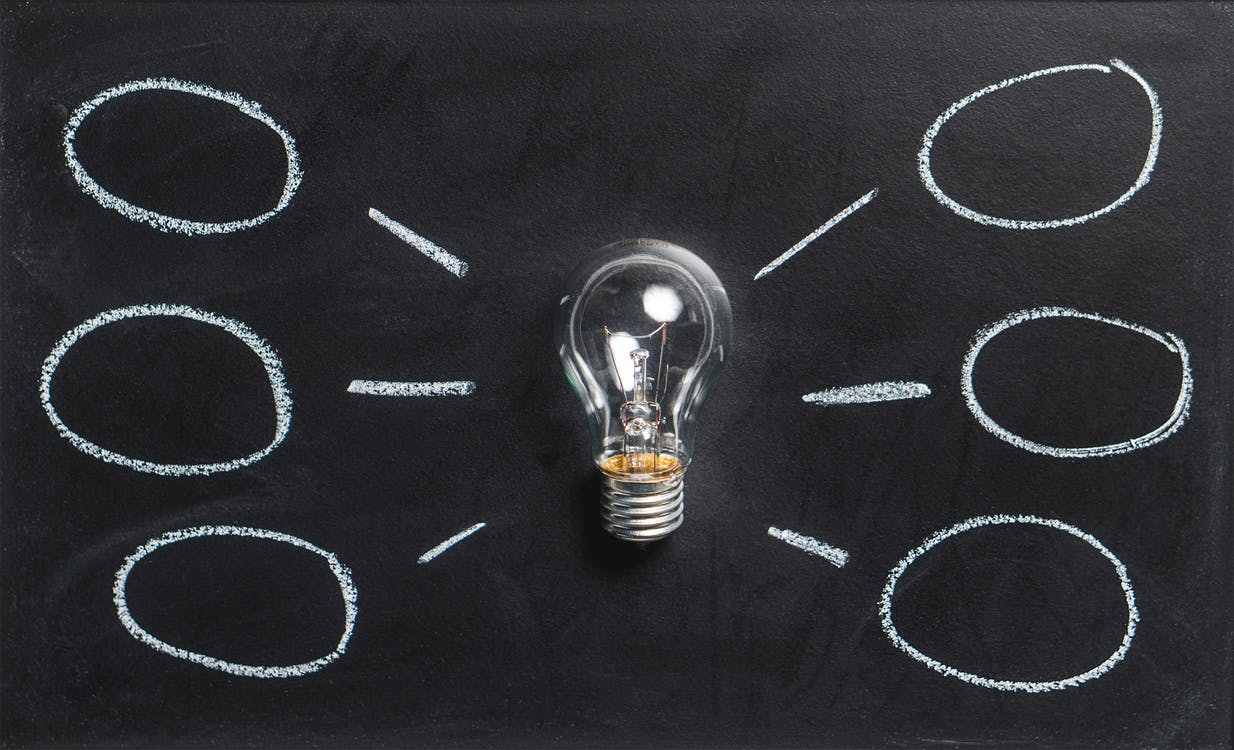
Elon Musk recently spoke out against the artificial intelligence and the threat it poses during a meeting of American governors, saying things like AI was the “biggest risk we face as a civilisation” whilst urging the US Government to adopt AI legislation.

Stephen Hawking warned in 2014 that: “The development of full artificial intelligence could spell the end of the human race,” in an interview in 2014. Two years later and Professor Hawking told an audience in Cambridge for the opening of the Centre for the Future of Intelligence that: “the rise of powerful AI will be either the best, or the worst thing, ever to happen to humanity. We do not yet know which.”
There are of course many proponents of AI as a way to improve business processes, lives and much more, such as Dr Scott Zoldi, Chief Analytics Officer, FICO, but it has to be built properly.
CBR’s James Nunns spoke to Dr Zoldi about the rise of AI, the positive role it can play, and how to avoid disaster by building it correctly.
JN: Artificial Intelligence has become one of the big buzzwords and has divided opinion as to whether it’ll be good for humanity or bad, what’s your take on it?
Dr Zoldi: Artificial intelligence will most definitely be good for humanity. Already we depend on it for safer air travel, detection of payment card fraud, and to navigate our automobiles. The applications of AI will continue to expand given the renewed interest in these technologies and easy access to computing power in the cloud and with a society confronted with masses of data being generated at unprecedented volumes.
Care must be taken to build AI models correctly and safely. Companies like FICO have been operationalising AI and machine learning for more than 25 years and joining this AI with people’s job functions successfully. Models of how to develop, govern, and monitor AI are critical to make the use successful.
JN: Tech companies seem to be throwing AI into all of their products, but is it truly AI? Are businesses using it? And what’s the impact been?
Dr Zoldi: Artificial intelligence is a very broad term of art — essentially it’s utilising machines to perform tasks that need human intelligence. This could include systems that auto-pilot an airplane or provide us traffic and navigation advice. It’s true that some companies may be jumping on the AI bandwagon by claiming that their systems include AI or machine learning — not all analytics are AI, for example.
Given our increasingly digital lives, many companies are using AI to process and quantify and classify the data they receive about us as customers or the systems around us such as the morning commute. The impact has been that in many applications it is clear that AI has positively impacted our personal lives. As an example, I sleep better knowing that the autopilot on an airplane is AI, and I am hard pressed to look at shutting off navigation on my phone and navigate streets (or worse traffic) with a paper map. In payment card detection, FICO’s AI neural network models have been protecting 2/3 of the world’s payment cards for the last 25 years, supplementing banks’ fraud operations and reducing fraud while reducing customer impacts.
JN: How do you develop an AI system that can do a job safely, efficiently, and doesn’t react catastrophically to something it doesn’t fully understand?
Dr Zoldi: Building AI correctly is a science, and not software code. Highly skilled data scientists who know the algorithms deeply should be building these AI. These scientists focus first on understanding precisely the data that will be used by the AI, what the quality of each data element is, and what could be anticipated challenges in the AI learning relationships in that data that might be undesirable. For example, in a fraud context, if there is a disproportionate amount of fraud reported in New York City in a unbalanced development data set, the AI may infer any transaction in New York City is likely fraud. Or the AI learns an undesirable relationship between velocity of spend and remaining available balance and likelihood of fraud, and consequently detects fraud cards but after nearly all the money is spent by fraudsters, and for good customers deny cards where legitimate use of the entire line of credit is warranted.
Read more: No longer just robots: the future of AI
One develops the model utilising train, test, and blind validation data sets to ensure that the model will not ‘overtrain’, meaning learns — that is, learn the specifics of the data set vs. generalising its learning. This is important for applications in the real-world where data will shift and change. (Incidentally, overtraining or ‘overfitting’ the development data set is something that can happen in non-AI modelling as well.)
Finally, the data scientist then must look at each ‘latent feature,’ the relationships that the AI is learning to make decisions. Many of these may be difficult to interpret by non-scientists given their mathematical representations but each latent feature must be understood as these are the driving factors behind the AI making decisions. When one does all this then the human understands the AI tool and how it makes decisions to ensure that it’s fit for purpose.
Even after this, one must monitor the AI in production to make sure it does its job safely and efficiently. The presentation of new unseen data is something that must be monitored both in terms of the data that is presented to the AI and also the scores/reasons that the AI produces. Some AI models are well generalised and can adjust to new situations, but if the situation or data presented to the model is completely different from what the model was trained on, that’s when you can get undesirable results, just like you can if a person who has been trained for a job gets into a situation they’ve never been trained for.
At FICO, we have refined something called auto-encoder technology that basically tells us when a model is encountering data that is far outside the spectrum of what was used to build the model. When this happens, the data scientists can consider redeveloping the model, and the human co-workers can look at the results the AI is generating to see if they should override or alter decisions.
JN: Should we trust AI to be in control of things like defence systems?
Dr Zoldi: AI is superhuman and can make decisions much faster than humans, which can be critically important to defence systems. We struggle to keep up with trivial amounts data presented to us, so you can imagine how challenged we would be to deal with the enormous amounts of data presented in an area like cyber defence. AI must be leveraged here to detect abnormalities and alert defence officials to the 0.000001% of data that must be carefully looked at by humans. In a situation where there is a massive attack, AI can be used to respond to these attacks at a speed and accuracy that humans can’t match, which improves a nation’s defence and ability to protect its civilians.
JN: Should we have strict guidelines on what AI should and shouldn’t be used for?
Dr Zoldi: My view is that AI should be considered for all applications. Instead of deciding where it should or should not be used, let’s determine the governing principles around AI model acceptance, AI monitoring, and processes around use of AI.
As an example, proper development of the AI model is critical. AI models should be built by expert data scientists who follow all the various regimen around proper development, vs. simply going to open source algorithms and running code. AI should be forced to be explainable both to the data scientist who is carefully designing the inputs, examining the internals, and the outputs, and to the analysts that use these models – this involves AI producing reasons for each prediction/decision. Finally, governance around model acceptance and AI production monitoring is critical. Industries like financial services have done this for years – all areas of use of AI need to adopt these principles and formally govern the acceptable use of these models.
JN: What would be the worst case scenario should an AI system go wrong?
Dr Zoldi: The worst case scenario occurs when an AI is improperly built and the data is changing. When a AI is improperly built, no one may be monitoring the relationships it’s learning during its training, and this may lead to spurious or unwanted relationships driving decisions. This often happens when companies naively think that throwing all of ‘Big Data’ at AI will make for better models – it often makes for models where the relationships are very difficult to disentangle.
Read more: Why the explosion of AI can no longer be ignored by businesses
Then when the data is changing after the model is in production, it may produce inaccurate or unreliable decisions. It’s critical to monitor the data for anomalies or shifts compared to the data on which the AI was trained. When the data changes too fast, governance and monitoring polices should hit the ‘shut off values’ on the AI.
JN: What safeguards should be put in place to help foster the growth and development of AI but to keep jobs and systems safe?
Dr Zoldi: Safeguards include ensuring that organisations are not falling for the AI hype. Believing these strange new self-learning models are without faults is naïve. AI is superhuman, but generally when it is working on data and situations that are routine and they are designed to detect. Understanding that AI must be built based on best practices, and produce explanations for each score and decision, will allow companies to implement governing principals around acceptance or rejection AI. When in production, the data must be monitored for situations where the AI may not be reliable. Finally, with all these checks and balances in place, one should not lose sight of the fact that the human jobs are to be supplemented by the AI, and therefore a continual feedback loop between human and AI is critical.
Any new technology that can automate or change a human task can potentially have a disruptive effect on the workforce. But most technologies that have replaced jobs have also led to job creation. I believe AI will have the same effect. People may focus at first on the loss of jobs in certain sectors, but it’s important to note that today — after all the technological transformation of the 20th and 21st centuries — unemployment in the most technological societies is not at record levels.
JN: What’s the future of AI, is there a limit as to how far it can go?
Dr Zoldi: I don’t see a near-term limit to AI, as long as we focus on responsible development and use. We need to make sure that AI models are properly developed (vs. throwing algorithms at data blindly), governed by the organisations that use them, and continually monitored. Many of these steps will run at human time scales so that we properly and responsibly utilise the AI to improve our businesses and lives.
At FICO, a large amount of my research today is in explainable AI. AI that will provide valid explanations is a hard problem. In the future, new AI algorithms will need to better explain how they produced a score or reached a decision. This is critically important for regulations like GDPR, but also for all the governance practices around AI model adoption and use.
AI will also start to explore the edges of curiosity to continue to drive adaptive technologies, such as we use in fraud detection today. We need to allow AI to adjust and learn within acceptable governed tolerances. This is how we will solve more problems faster in a fast-changing world.