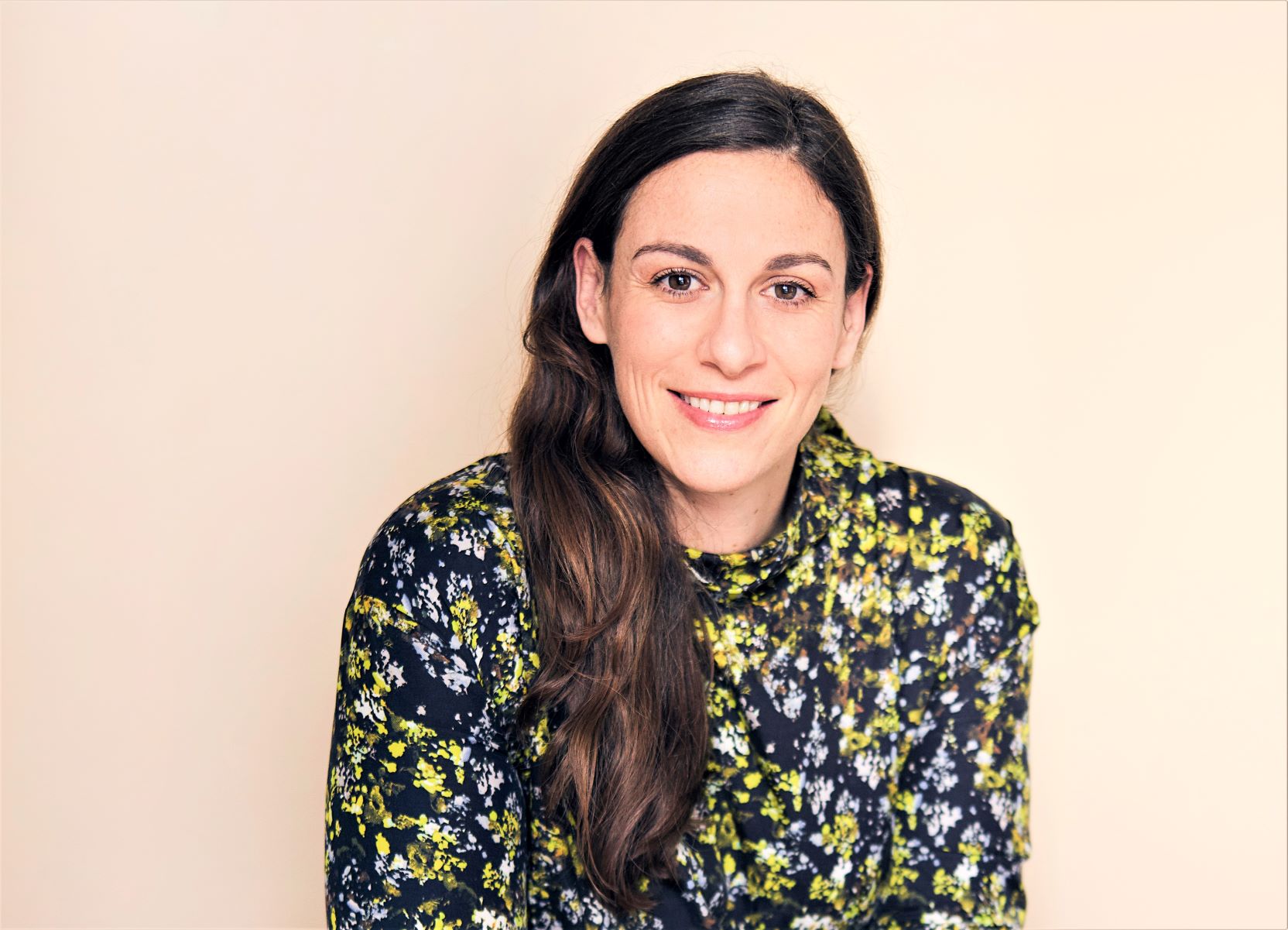
Carly Kind is the Director of the Ada Lovelace Institute, an independent research institute and deliberative body with a remit to ensure data and AI work for people and society. She writes for Tech Monitor as part of BCS president Rebecca George’s guest editorship.
Technology has been, at times, the hero of 2020 – enabling us to work, shop and connect from the confines of social isolation, accelerating Covid-19 vaccine efforts and keeping us sane with TikTok dances and cheesy memes. But it has also sometimes played the villain. A ‘mutant algorithm’ saw students take to the streets to protest against their A-level results, the groundbreaking text model tool GPT-3 returned racist and sexist results, and concerns about algorithmic bias continued to plague AI applications from advertisements on websites to facial recognition.

After years of debate about the ethical principles applicable to AI and automated decisions (there were at least 76 ethical codes and guidelines published between 2016 and 2019), we are starting to get a clearer picture of the practical challenges of ensuring the ethical development and deployment of AI. These include:
- The problem of algorithmic bias and discrimination, which sees automated systems delivering differential outcomes for minority or underrepresented groups, and entrenching societal biases which underpin the datasets used to train learning algorithms.
- Ensuring justice and fairness in algorithmic systems that struggle to take account of cultural or societal context.
- Building up public trust and confidence in AI and algorithmic systems, overcoming the power asymmetries between individuals and technologies, and the need to ensure human review of and appeal from automated decisions
- Balancing the need to enable individual privacy and informational control, on the one hand, with incentives to collect and retain data for the development and improvement of AI and algorithmic systems, on the other.
There is still no clear solution for how public or private sector entities developing or deploying AI can clear these hurdles. As with previous industrial shifts, the growth of automated technologies will necessarily involve trial and error as we develop a collective societal settlement on the right and wrong ways to integrate and use automation and algorithms in our societies.
We are beginning to witness some companies, practitioners and policymakers attempt to translate ethical principles into practice. Some have been more successful than others. Moves by Amazon, IBM and Microsoft to stop selling facial recognition software to US police forces were widely regarded as important indications that companies are starting to see ethics as critical to their bottom line. Other attempts have faltered. The Ofqual A-levels algorithm evidenced the complex challenge of giving effect to notions of fairness and equality in technical systems; and the novel establishment of an Ethics Advisory Board to oversee the development of the NHS Covid-19 Contact Tracing App was undermined by its ultimate disbandment.
Despite these early false starts, there are a range of emerging options on the table to facilitate ethical development of AI and other new technologies. Risk assessment and impact assessment tools are being developed to aid researchers and technology professionals to think through the potential ethical impacts of their work at each stage of development.
Omidyar Network’s Ethical Explorer is one such tool; the Open Data Institute’s Data Ethics Canvas is another. McKinsey’s ‘Derisking AI by Design’ approach and Rolls-Royce’s forthcoming AI ethics framework are two private-sector attempts to move beyond principles to the implementation of ethics assessment and mitigation. In the public sector, it is possible the UK might soon follow Canada in mandating algorithmic impact assessments (AIAs) for public sector procurement of AI tools. The use of AIAs in the context of healthcare applications of AI and data-driven technologies is also likely to be an emerging area of research.
Building trust in AI through independent assessment
In public deliberation initiatives held by the Ada Lovelace Institute, we’ve found consistently that public trust and confidence in new technologies is dependent on independent review and assessment of contentious applications. As such, external auditing of algorithmic and AI systems, including technical quality assurance, holds real promise as a mechanism for ensuring ethical compliance while also shoring up the public legitimacy of new technologies. The development of external audit or regulatory inspection regimes for algorithmic systems and AI, in the manner of financial services regulation, would also create an opportunity for the UK to be at the forefront of an AI ‘audit market’, according to the government’s Centre for Data Ethics and Innovation.
The appointment of an ‘Ethics Officer’ is becoming an increasingly popular practice in some industries, but the effectiveness of an internal ethics champion in changing practices remains unclear. A recent survey showed that because most companies prioritise metrics framed around efficiency, engagement, productivity and short-term profitability, measuring and articulating the long-term benefits of ethical decision making (particular when such decisions may come at the expense of short-term product success) impedes the ability of ethics officers to impact decisions.
The study showed that for ethics to really be embedded in a company’s practices, we will need to see cultural change. The people responsible for designing, developing, testing, implementing, and assuring algorithmic systems should understand not only the systems themselves, but the social context in which they will be deployed.
That means ensuring tech development pipelines are diverse – in race, gender, ethnicity, socio-economic background, lived experience, and perspective – and interdisciplinary. It means that responsibility and ethical action should become linked to employee performance, and employees should not fear retribution or harm for internally raising ethical issues. It may also mean that we need to think about the professionalisation of data science, computer science and IT itself. Perhaps a professional accreditation scheme in the matter of accountancy or law is a proportionate ask of the people who will be designing and executing the infrastructure of our online and offline lives.
This year may have shown us the potential of algorithms and AI to improve our lives and our societies, but it has also drawn out the complexities of these new technologies. Translating principles into practice is at the top of the agenda for any organisation wanting to develop and deploy this tech, and we now have some concrete mechanisms – such as impact assessment, audit, and ethics officers – through which to begin to make progress.
Ultimately, however, achieving ethical AI is a process, not a destination, and the more people we can bring into that process – from corporate leaders to computer science students – the more likely we are to reach a sustainable settlement of ideas and practices.