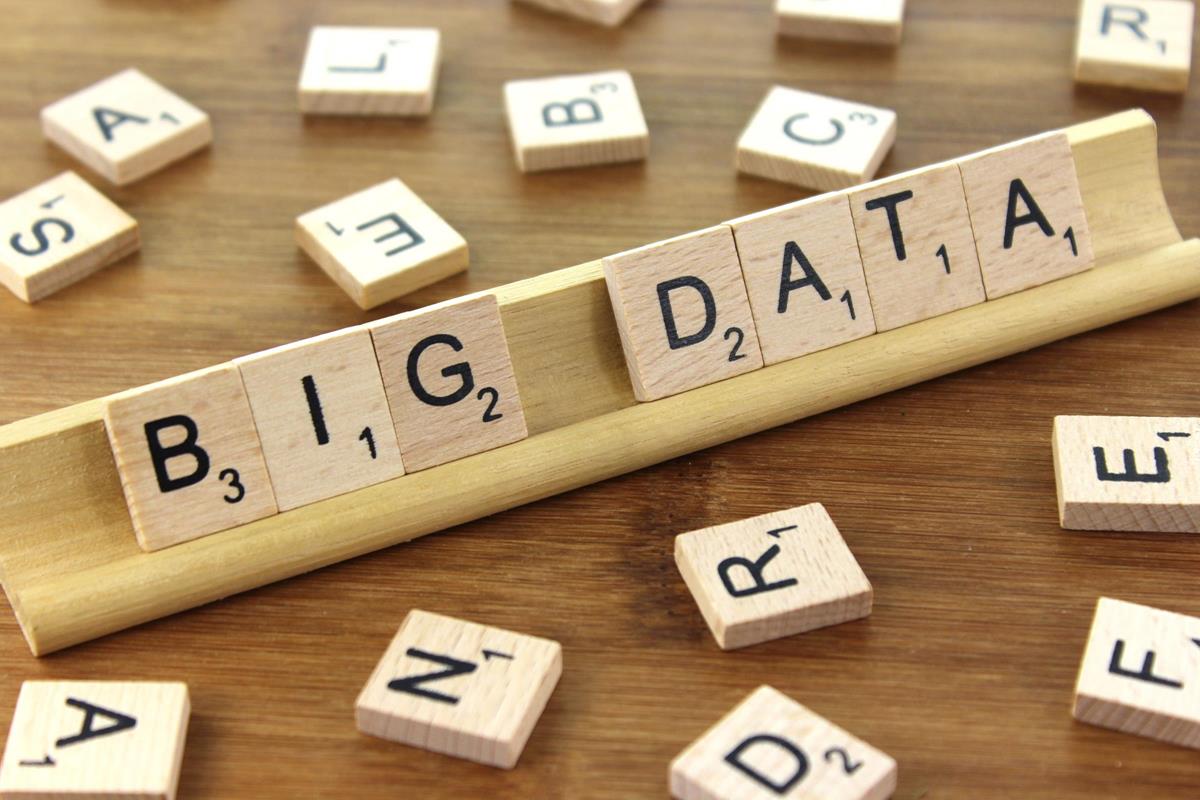
With numerous reports on the exponential growth potential of trends such as IoT, machine learning and cognitive app development, there is no doubt the world is becoming increasingly connected and apps are becoming more intelligent.
They know more about users and their behavioural and consumption trends and continue to make great strides in being able to cater for needs and preferences before users explicitly express them.
As an example, B2C organisations such as Facebook, Sephora and Uber are using voice powered assistants and chatbots that are capable of interfacing with a consumer and predicting their needs. When these AI technologies talk to the consumer they are able to: recognise who they are from previous purchase history, understand voice commands and new requests, then predict and serve relevant real-time service or product suggestions.
These cognitive first consumer applications use predictive analytics and machine learning to take the Internet of Things to the next level, allowing consumers to enjoy the ease of use, efficiency and productivity benefits of shopping, ordering and scheduling in such a way.
This is exciting but nothing too new. Predictive analytics and machine learning have long been discussed in fields like marketing and advertising where analysing a constant stream of data and automating responses on the right platform, at the right time, has been used to target and engage with consumers. However, now we are seeing the variety of predictive applications and new use cases.
Cognitive first applications and business
Today there are a lot more consumer examples for cognitive application development and predictive analytics than for business and when B2B examples are found, they are typically the reserve of those who have the resources to scale their solutions and tackle platform and data integration challenges, such as GE and Siemens.
However, the great potential for both B2B and B2C cognitive application and predictive analytics is for everyone not just the few who can afford it today. Take, for example, manufacturers who supply washing machines to huge hotel chains. The software in washing machines has been available for years and has been used to gather data that could be used for predictive analytics – to tell when individual machines may break down and/or need repair. Now that the IoT is a reality, manufacturers can use this information to offer timely and relevant additional customer services based on sophisticated software that can truly interrogate, interpret and use data.
For the customer, diagnosing a fault in a device or appliance before it happens can be cost and time effective. This means that predictive maintenance and condition-based monitoring services can prove critical for the organisation’s business model, especially when it comes to eliminating costly machine breakdowns and unplanned system downtime. In addition, addressing a potential issue with a product before it becomes a problem can do wonders for a business’ brand equity.
Take, for example, a business using Microsoft Surface Pro machines for its day-to-day operations. The recall of the Surface Pro AC power cord earlier this year due to overheating would clearly have had impact on how the business operated. The employers would have likely experienced problems with the machines and the added pressure of getting the replacement cords would have been created on the company. Predictive analytics can identify these issues ahead of time, allowing businesses to address them before they reach the public eye. In this way they can eliminate the need for reputation-damage-control.
The challenge: drowning in data
Companies can often be overwhelmed by the sheer volume of data which limits their capabilities to process and effectively use it. As data multiplies with the advent of the Internet of Things, the ability to handle, integrate, model and construct data becomes increasingly difficult, especially for organisations that do not typically sit within the ‘technology giant’ bucket. This is because they are still struggling to unite data collected from software sensors with data from systems of record and layer predictive analytics and machine learning on top.
Most importantly, the ability to locate and interpret the right data to provide precise answers for predictive scenarios can be like searching for a needle in a haystack. Corporations have hired thousands of data analysts to do this, but SMB and large companies simply do not have the budget to do the same.
Think Cognitive-First
Businesses must therefore overcome these challenges by implementing a powerful development platform and integrating it with AI technologies – machine learning and predictive analytics.
To do so successfully, organisations will also need to ensure their technology platforms cater for both their current and future needs and can integrate with modular based AI technologies that enable advanced machine learning). This would enable data gathered from traditional analytics software to be used repeatedly and for future, predictive context rather than just for a one off process. Machine learning must also be able to manage hundreds of different data variables, model and structure data trains so that they can provide precise insights across multiple different user case scenarios. They will also be able to scale these insights as data sets scale and multiply.
From an application development perspective, businesses will also need to get to grips with speech patterns, semantics and contextualised development. Up until now, when building traditional systems, they used to begin with process and logic, writing the code and ultimately inserting the data. A cognitive-first application development strategy, however, follows a different path. Developers need to lead with the data, being able to gather all of the information in the moment, and let it drive them to the logic.
Prepare now for the Cognitive Future
Cognitive application development, predictive analytics and machine learning build on top of layers of existing technologies and applications to make them more powerful. They emulate the evolution of the human brain with its different interconnections and complexities. These must be catered for at the back end through sophisticated integration and machine learning technologies that can take existing and ‘learned’ datasets and merge with traditional analytics, to ultimately take apps one step further and produce predictive analytics. In doing so, we will see the adoption of many more B2B cognitive use cases and examples.