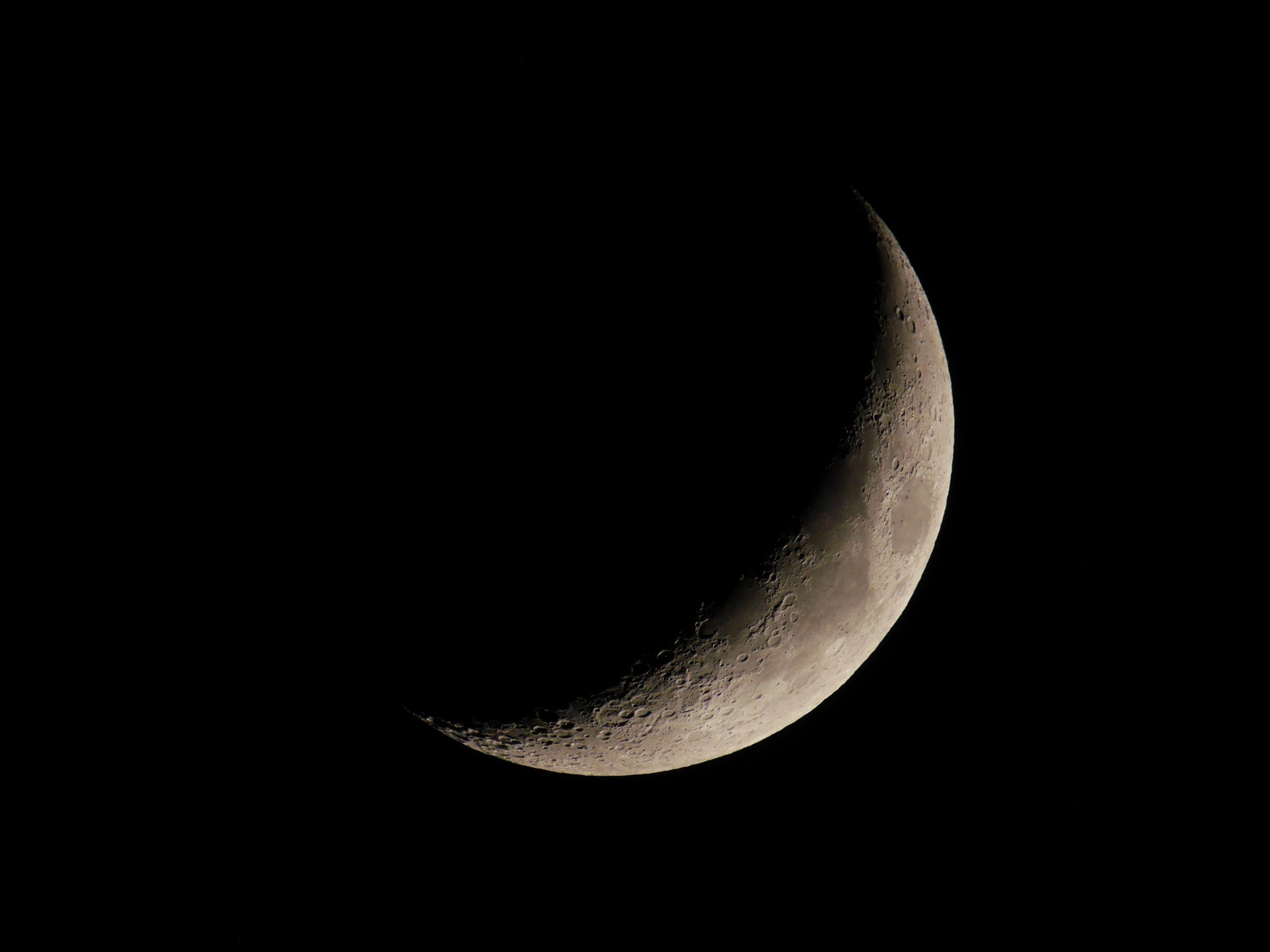
AI Applications: A Snapshot
Artificial Intelligence can seem ephemeral when it comes to enterprise applications, with many companies struggling to deploy it; either because their data isn’t clean enough, in the wrong format or they simply don’t have the capabilities.
At the Intel AI Devcon in San Francisco this week there were more than a few vendors pitching industry uses for the emerging technology. Computer Business Review took a tour of the conference floor…
“So Much Gobbledygook!”
Lenovo: “We have trained this model to detect whether a can is damaged or defective using a small data set of 16 images of different cans,” says Robert Daigle of Lenovo as he puts cans in front of a camera on a workbench.
The screen to his right flickers quickly as each new image is captured and read. It displays the defects of the beat up aluminium can by telling you what percent it is damaged, otherwise it “gives a green signal that this one doesn’t have any defects.”
The companies general manager Madhu Matta steps in at this point to say the product is a; “manifestation of a very simple vision we have.”
The product been tested on the line doesn’t need to be a can; any product checked by a human on a belt could be set up to be quality checked by the software.
AI is hard, Matta emphasises, pointing to a few of the more technical white paper stands dismissively: “So much gobbledygook”.
He wants businesses to know that in most of the cases they have already done the first step, “taken a repository of massive amounts of data.”
When it comes to installing this type of system into your business, Matta recommends “start small and scale out as you need.” No point in spending a large sum on a system which you only use 20 percent of the time.

EchoPixel: “EchoPixel have taken slices of a MRI brain scan and turned it into a 3D image that a surgeon can then move around to see all the sides, or move through into the middle where a tumour might be that has to be operated on.
Two things are in play here, first it’s utilising deep learning software developed by Intel to identify the tumour in the MRI brain segments. “It works via a biomedical image segmentation task,” explains Mattson Thiemem “by “taking two dimensional MRI images and producing essentially maps of where we think the tumours are.”
“The network takes as input a two dimensional single channel MRI image and produces as output an equivalently sized binary mask where each pixel assigns a class, tumour or not tumour”
The second bit is the interactive 3D image developed by EchoPixel. Wearing 3D glasses you can view the 3D brain and via a pen, take the tumour out to look at it.
On a normal MRI brain segment you can see a blood vessel, but you don’t know immediately where it turns, if it turns. With the 3D image you can follow it thus informing your cutting hand.
As Thieme was walking me through the technology an attendee at the conference walked up and when engaged with the device explained “I have a brain tumour” pointing to a noticeable lump on his forehead.
Luckily it is benign, but his interest in the tech was clear to see.
However talking to others at the event about the technology, some see it as more of a marketing gimmick which focus on the wrong points of using machine learning and may actually be just a small step in the right direction not a leap.
Google’s work with deep learning to identify tumours was cited more than once as the right direction: its prediction heatmaps produced by an AI algorithm had improved so much that the localization score (FROC) for the algorithm reached 89 percent, which significantly exceeded the score of 73 percent for a pathologist with no time constraint.
Moon Mapping
NASA Frontier Development Lab: As better space faring technology is being developed, especially automated spacecraft, we begin to turn to space for the potential of mining natural resources. Our closet potential mining opportunity is of course our Moon. Yet we still have not fully mapped out the Moon’s surface, which is pocked with craters.
Sara Jennings of NASA Frontier Development Lab talks about how they “work with NASA and private partners, one of which is Intel on a space resource challenge.”
These include challenges like “how to find resources on the moon like water?” “To find where resources are they need to have better lunar maps”
This poses particular challenge: The moon fully rotates on its axis every 27 days, but it also takes 27 days to orbit the Earth. So the effect is we only every see one side and the rest is in darkness. This then makes mapping the Moon; “very hard to do by hand because a lot the craters are in permanent shadows,” says Sara.
Using data and images captured by the Lunar Reconnaissance orbiter which was launched in 2009, they have set up a “computational neural net to help identify craters, with 89.4 percent accuracy.”
Included in the development of the software is a science tool game, where users circle a crater they have identified, which is then used for data labelling which; “feeds back into the algorithm to make it better,” explains Sara.
Whether you’re mapping the moon, tracking soft drinks or identifying tumours, real world – and sometimes off-world -applications are clearly coming thick and fast.