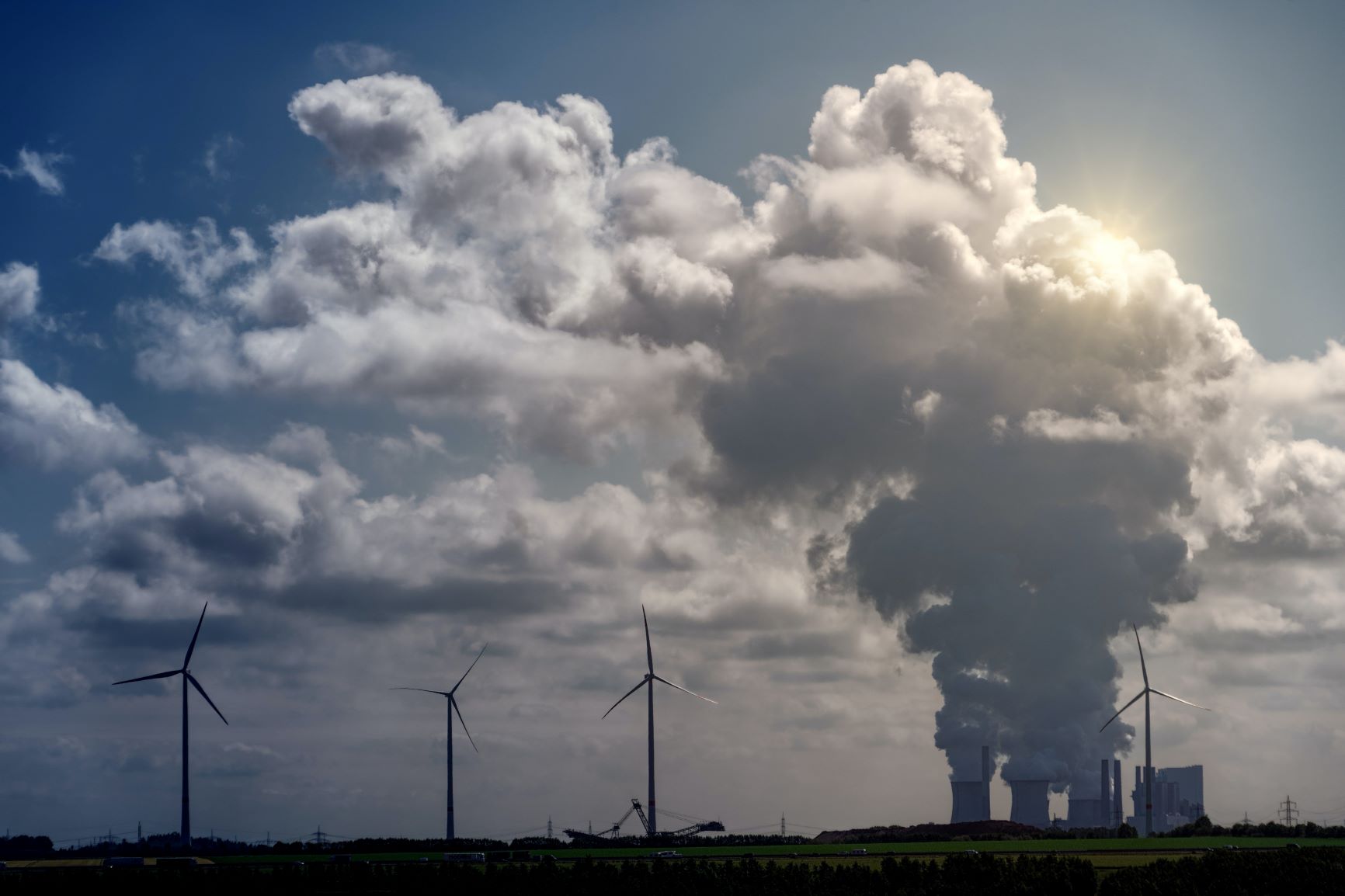
The share of wind and solar energy in the global power supply will need to more than triple by 2050 if the planet is to reach climate neutrality goals, according to estimates from the International Energy Agency. One crucial enabler for this will be the ability to match supply to demand accurately, despite the intrinsic unpredictability of renewable energy sources. As a result, applying AI and machine learning to renewable energy could be one of the technology’s most transformative applications.

Forecasting the output of renewable energy sources requires accurate weather predictions and a precise knowledge of how that weather will interact with wind turbines and solar panels. Predicting demand requires up-to-the-minute data on both energy consumption and the market price of power. Training machine learning models on an expanding source of datasets, satellite imagery and climate physics can help on both fronts, experts say, and help the world achieve carbon neutrality by 2050.
Machine learning to predict the weather
Current power grids were designed for electricity derived from thermal plants, powered by fossil fuels or nuclear energy. This kind of energy is described as ‘dispatchable’, meaning that it can provide a specified amount of power on demand. Energy derived from renewable sources such as wind and solar does not produce a steady, predictable output as both are subject to the chaotic behaviour of the weather, which typically results in surges and lulls.
A clear goal for researchers seeking to boost the share of renewables within the energy mix is to make wind and solar power more ‘dispatchable’. To do this, they are working to make output predictable, so that grid operators know how much power to feed into the grid and how much to store for future demand.
Jack Kelly, co-founder of non-profit climate change research and development lab Open Climate Fix, is among those using AI and machine learning to achieve this goal. Open Climate Fix’s primary objective is forecasting energy output of solar panels by predicting cloud cover. To do this, it analyses near-real-time satellite imagery.
“Every five minutes, a satellite takes an image across 12 different channels, including visible infrared and water vapour,” explains Kelly. These satellite readings and different data sets are fed into machine learning models which extrapolate from present cloud movements, revealing how much solar energy will be produced in the near future.
Another way to predict solar and wind output is to model the physics of the weather itself. “You sit down and you say, I think that heat moves around the atmosphere in this particular way,” Kelly says. “You write down those equations and then you run those on a supercomputer. As long as you do a good job of telling it what the world looks like, then that is a kind of top-down approach to modelling the atmosphere and doing forecasting”.
The UK’s National Grid uses deep learning methods to anticipate the impact of the weather on wind and solar output.
“We have hundreds of substations throughout the country, basically hubs where the power is distributed and connected,” explains James Kelloway, energy intelligence manager at the National Grid’s Electricity System Operator division. “For each one of those we run an ensemble of models. One will be tuned for a really sunny day, one will be tuned for a winter day, and so on.”
Each of these models is trained to predict the impact of the weather on each substation under particular conditions. Together, they allow the National Grid to anticipate how the weather will affect output from renewable sources around the country. “If you’ve got a cloud front moving in and [a model] has seen something similar before, we can predict the likely impact of that front on solar generation.”
If an engineer who controls a solar panel knows how much energy to expect on an oncoming sunny day, they can to arrange to store what they don’t immediately need, rather than turning it off when they have reached their quota, bringing them closer to dispatchable energy generation.
Forecasting energy demand with AI
Making renewable energy more ‘dispatchable’ also requires accurately forecasting demand: knowing how much electricity will be required allows grid operators to optimise the energy mix.
OVO Energy is a UK-based provider that buys energy from both renewable and fossil-fuel sources and sells it to the retail market. The company aims to reach carbon neutrality by 2030, using machine learning to forecast energy demand as well as supply.
That requires modelling the energy profile of its customers’ buildings says Conor Maher-McWilliams, head of flexibility at Kaluza, OVO energy’s technology platform ecosystem. “The first thing you need is how much energy is required to heat your home. We need to understand the physical parameters of the building, how leaky it is and how well it stores heat, and then build predictive models [using machine learning] around your home and your heating system”.
Once engineers at OVO understand how much energy is needed to heat a given building, they can compare this data against a forecast of when there will be sufficient green energy on the grid. “With those two forecasts, you can start to plan when you deliver that energy to your heating system, to coincide with periods when there’s the most renewable energy on the grid.”
A forecast of the market price of the energy is also needed to judge how much the customer should be charged. “Within a given day, the price moves around quite a lot. So we need to continually forecast and re-forecast what we think that price is going to be in real time, using the market data that’s available and some AI-based algorithms in order to really hone our prediction.”
Energy providers are also using machine learning to fit the needs of individual customers, which further helps them to optimise the use of renewable sources. UK-based gas and electricity supplier Octopus Energy, for example, analyses fine-grained consumption data to categorise customers by their usage habits.
“The old approach would be to use external data about your customer to model their consumption,” says data scientist David Sykes. “But actually, all the information [we need] is already in the time series data about their consumption.”
“We’re using a machine learning technique called ’embedding’,” he explains. “The model implicitly learns that two customers are quite similar to each other: they might both use night heaters, for example.”
Categorising customers based on their actual consumption allows Octopus to forecast demand more accurately. And because the system is always learning, it automatically updates in response to new conditions, such as the Covid-19 pandemic. “You can capture that change immediately because you’re looking at [customers’] real behaviour, as opposed to having given them a static label,” says Sykes.
Feeding renewable energy into the grid
Once the energy has been harnessed, it needs to go into the grid, in the right way and at the right time. AI and machine learning are used in this process, but, according to Kelly, it will be a long time before humans are completely excluded.
“It’s the electricity systems operator’s (ESO) job to fine-tune supply to exactly match demand in real time,” explains. “It is still quite manual, so it’s humans looking at what’s happening on the grid now and humans instructing generators to change their behaviour to try and better match demands, over time horizons of an hour or less typically.
“There will be humans in the loop for a long time to come. There have been attempts to train machine learning models to do this, but it’s really hard. From a regulatory perspective, they’ll probably want humans in the loop for a while.”
In the first quarter of 2020, coal and gas made up 60% of the global electricity supply, while renewable sources made up just 9%. Wind and solar have the potential to provide clean power to most of the planet but to do so they must be reliable and predictable. Human oversight may be essential for the foreseeable future but AI and machine learning evidently have a vital role in this energy transition.