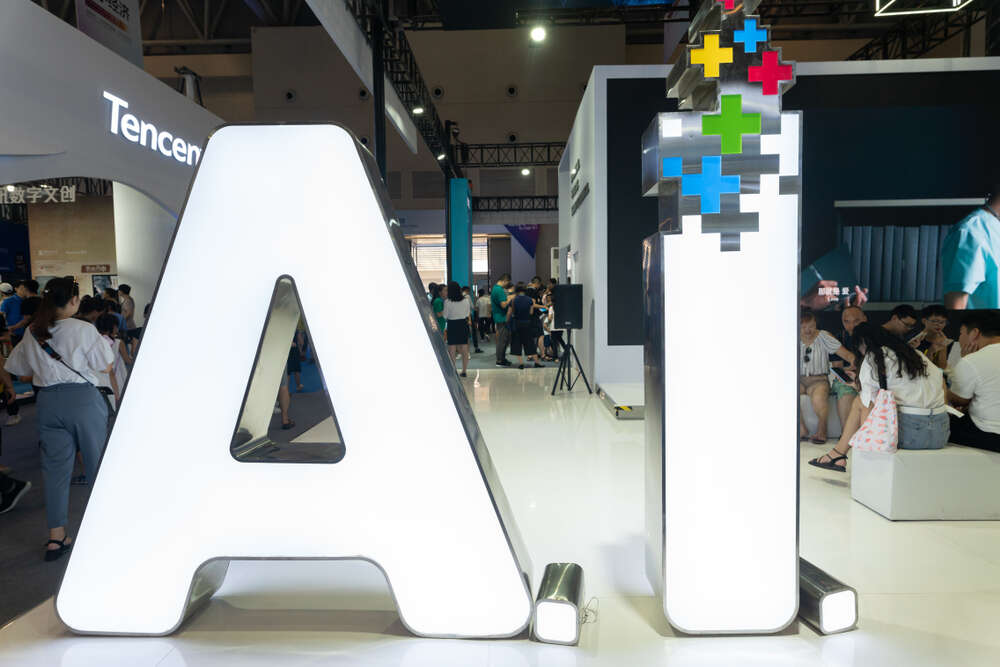
“When you’re fundraising, it’s AI. When you’re hiring, it’s [machine learning]. When you’re implementing, it’s linear regression.”
This popular developer quip encapsulates the ambiguity of the language of ‘artificial intelligence’. The words that describe these systems and what they do are vague and often context-specific. AI and related terms such as algorithm, machine learning and automation have specific meanings for expert ears, but they are often used interchangeably by laypeople.
This is not without consequence, as a new working paper from researchers at Saarland University and the Max Planck Institute demonstrates. In fact, the choice of term can affect how these systems and their output are perceived. It is an insight that technology leaders should consider when implementing “AI” systems for internal or public-facing use.

The study’s authors are human-computer interaction researchers who noticed that, even among their fellow scientists, the terms AI, algorithm, automation and more are used interchangeably, making similar studies difficult to compare. They sought to investigate whether the choice of language affects the perceived characteristics of “AI” systems, both in general and with respect to specific tasks, and so conducted a pair of surveys.
The impact of language on the perception of AI
In the first survey, they asked internet users to rate AI systems for various characteristics, such as their tangibility, complexity and the extent to which they have human-like characteristics (anthropomorphism). The questionnaire used different terms for different respondents – alternative terms for AI included ‘automated system’, ‘decision support system’, ‘robot’ and ‘sophisticated statistical model’.
This survey confirmed that the choice of words did affect the perceived characteristics of the system being discussed. 'Artificial intelligence' was perceived to be both more complex and human-like than the other descriptors, for example, but less 'controllable' than computers, robots or computer programs.
A second survey examined whether the choice of name impacts how people perceive the output of these systems. Respondents were shown hypothetical workplace scenarios in which an AI system makes a decision affecting a human worker, such as assigning work. The researchers again varied the term used to describe the AI and compared how respondents rated its actions for 'fairness', 'trustworthiness' and 'procedural justice' (freedom from bias, for example).
Again, the terminology was shown to affect respondents' assessment, with 'artificial intelligence' seen as less trustworthy and fair than the alternatives. The term 'statistical model' was seen as both the most trustworthy and the fairest (differences in procedural justice were not significant).
The researchers note that terminology is not the most important factor influencing how AI systems are perceived – other factors, such as the nature of the task it is carrying out, have a greater effect. Nevertheless, they say, the study suggests that nomenclature affects how systems are not just perceived but also adopted.
"If people are more likely to trust a statistical model compared to an artificial intelligence to [assign work], they may also be more likely to actually use and rely on a system described as being a sophisticated statistical model. Similarly, if people associate higher machine competence with artificial intelligence compared to automated systems, they may more likely use outputs generated by a system that is described as an artificial intelligence."
They also caution journalists and policymakers to choose their words wisely. "Take the example of policymaking documents where the authors may have the choice to use the term artificial intelligence compared to more familiar terms such as computer programs," they write. "Maybe if the European Commission’s 'Ethics Guidelines for Trustworthy AI' would have been called 'Ethics Guidelines for Trustworthy Computer Programs' there would have been less public outreach."