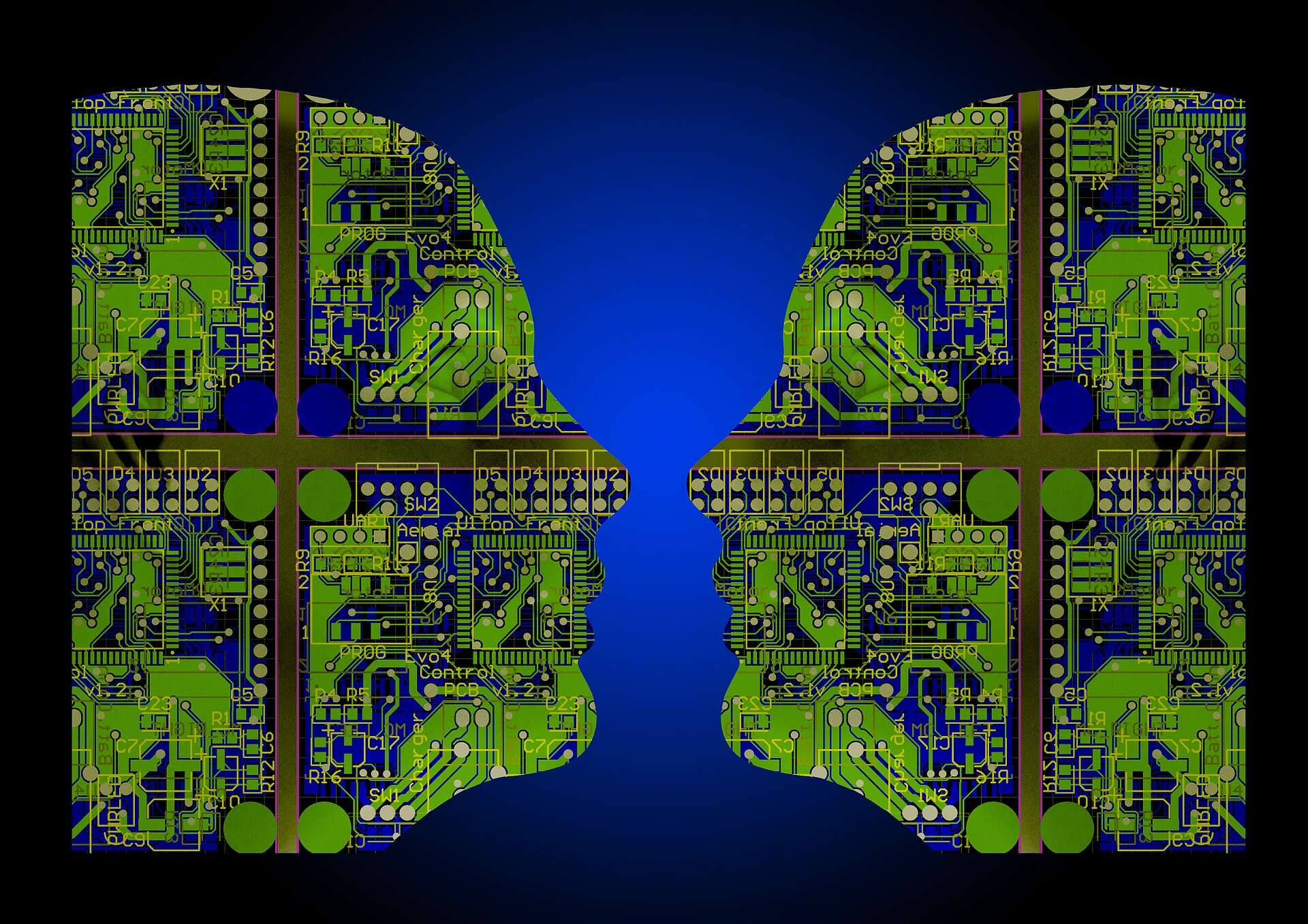
Professor Gina Neff is a Senior Research Fellow and Associate Professor at the Oxford Internet Institute, a research institute at the University of Oxford dedicated to the social science of the internet. The co-author of a new study, AI @ Work, which looks at artificial intelligence in the workplace, she joined Computer Business Review to discuss the hype and misconceptions around AI, as well as ways to ensure smooth integration of machine learning.
Hi Gina. Can you start by telling us a bit about your work.
I’m a Professor of Sociology, primarily interested in work and organisations, so I think about the people side of the economy, particularly the relationship between people and technology.
You’ve recently co-authored a report looking at the implementation of AI in industry. Can you tell us how that came about?
We hear a lot of stories about AI failures in the workplace, and what we wanted to do with this report was to see what we could learn from analysing the cases that get reported.
So we looked for reports in news outlets, academic papers and industry publications that covered the gap between how AI is said to be performing and what it actually does in practice.
So is that gap a wide one?
Yes, and our report is a bit of a “dog bites man” story. What we know is that news coverage about artificial intelligence is overwhelmingly dominated by the companies that are making and selling solutions.
And so we were looking for the stories that are out there about companies outside that sector, in other industries where they’re grappling with how they make AI technologies fit with their in their workplaces and with their staff.
Do you think there needs to be a greater understanding from industry of what AI is capable of? Or is it a case that systems are being hyped beyond their capabilities by the vendors?

Some of the most egregious headlines we saw described services being sold as completely automated when the work is being done as human labour elsewhere. So it’s still a buyer beware environment in many of these projects.
At the same time we’ve also seen the stories recently around exam results and the over-reliance on algorithmic and machine learning projects to make kind of hard choices that should have never been delegated to automated decision-making
There are common challenges that companies implementing AI systems are facing, and we hope can take lessons from.
What did you make of the fall-out from the A-Level results fiasco? Did it surprise you that misconceptions around AI exist at the highest level of Government?
Our report highlights three key challenges with AI in the workplace, around integration, reliance and transparency. In the case of the A-Level exam results, we see all three; there’s a challenge of integration because the A-Level results are part of a much bigger ecology that a lot of people – students, their families and universities – depend on in order to make certain decisions, many of which were taken way before the Covid-19 crisis.
In terms of reliance, we see an organization that relied on the seeming appearance of fairness in an algorithm rather than on the assessment of teachers, who may have had better information and whose grades would certainly have seemed fairer to the people involved. And then finally, in terms of transparency, we have a notion of algorithmic transparency. How does the model work? Is it a fair model?
In this case, and in our report, we want to call out that social transparency; who’s doing the work and who’s making the choices? And that’s something that all organizations need to be able to answer, regardless of how their proprietary algorithms work.
So what can organisations do to make sure their AI projects run smoothly?
It’s really clear from the cases we looked at that many companies aren’t prepared for the long haul, that they underestimate the time and resources that it will take to get a project up and functioning, much less a project that really offers a strong return on the significant investments in time and resources that they will have put in.
On the brighter side, we need to have a much bigger conversation in society about what the opportunities and promises are around AI, and what the real limitations are of the technology we have have at the moment. I think there’s still a lot of education that needs to be done around ensuring that the the goals of projects are realistic, so that’s something for business leaders to bear in mind.
I would also recommend to executives that they learn to be quite critical and make sure that they are asking the right questions to ensure that the AI projects being implemented are meeting the goals that they’re setting.
How important is staff buy-in when introducing AI to a business?
If there’s one key takeaway that I would want to to emphasise, it would be that the human resources already existing in a company or some of the most undertapped resources when it comes to implementing AI projects. Their knowledge of the business and their areas of work can be invaluable.
Many companies say they can’t hire data scientists fast enough, but we also need to be able to translate data science into good decisions. The key skill set of the next decade will be in that translation work between artificial intelligence and the C-Suite.
Download the full report here.