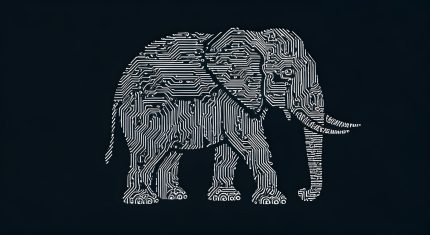
“We have an emergency,” announced President Trump – and the only cure, it seemed, was more data centres. Flanked by a trio of leading tech executives, hands crossed and eyes diverted like reverential bouncers, Trump described how his administration would bring AI home to America. That would start, he said, with a $500bn investment in AI data centres across the US. “I was in the real estate business,” Trump reminded anyone who didn’t know, so he knew something or two about the scale of what lay ahead. “These are big, beautiful buildings that are going to employ a lot of people.”
But what does it even mean to have an AI data centre? For most industry insiders, the term refers to a data centre capable of supporting the demands of heavyweight AI workloads. That includes training foundational models, particularly the large language models underpinning GenAI. But it can also mean heavyweight real-time inferencing or data analytics which relies on massive amounts of data.
As Chris Sharp, CTO of Digital Realty puts it, that means “lots more computing power, faster networks, and smarter storage than traditional data centres. They use high-performance GPUs, or even TPUs, to process complex AI models, which means they need higher power densities and advanced cooling solutions – like liquid cooling – to manage the heat generated from dense computing tasks.”
None of this is cheap. Overall spending on AI infrastructure will hit $200bn by 2027, according to IDC, with the vast bulk being accounted for by servers with “embedded accelerators”. Almost three-quarters of these units, meanwhile, are destined for cloud or shared environments.
Figures from real estate firm CBRE show acre after acre of data centre space coming online over the coming years, predicting that Europe’s data centre supply will grow 20% in 2025. The US, too, saw record data centre construction last year, record low vacancy rates and record preleasing rates of 90%.
So where does that leave the bulk of CIOs when it comes to contemplating their data centre strategies to support their AI ambitions? And what are the factors shaping those strategies?
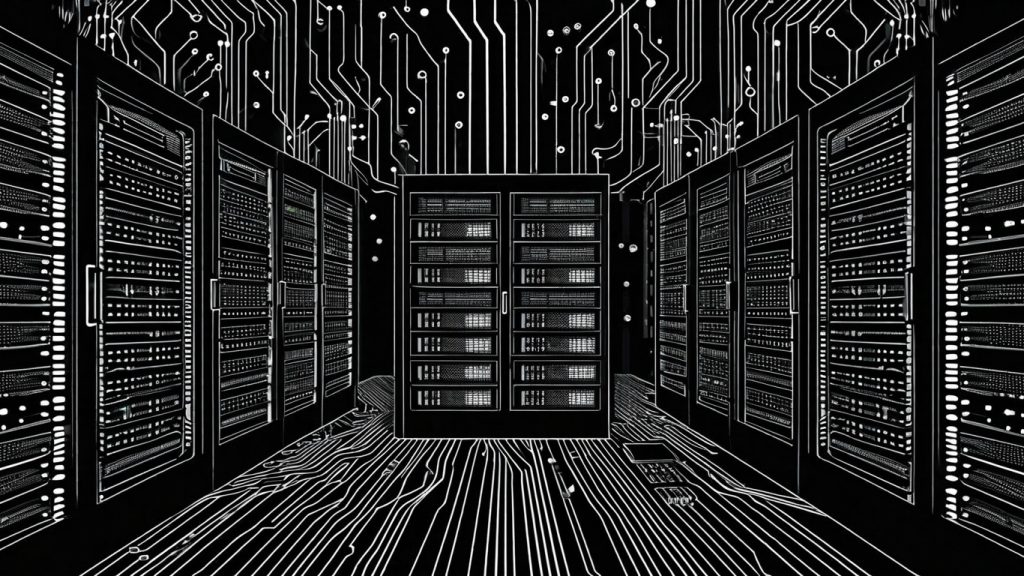
AI data centres are all about money and power
Some of it appears to be posturing. Consider that Facebook founder Mark Zuckerberg last year told investors that it was training Llama 4 models on a system that was “bigger than 100,000 H100 AI GPUs” which, if the rumoured price of $25,000 for that chip is to be believed, is equivalent to an investment of $2.5bn. Anyone eager to deploy Nvidia’s H200 chip at a similar scale, meanwhile, would need to cough up even more money – the price of that unit reportedly stretching to $35,000.
Meta isn’t just paying for silicon, says Sharp. Its data centres will “also have ultra-fast, low latency networking, often with five to ten times more cabling than regular data centres to keep data flowing smoothly,” he explains. What’s more, they’re often designed with energy efficiency, zero-trust security architectures, and seamless integration across cloud, edge, and private environments in mind, “making AI deployment faster, safer, and more adaptable.”
But it’s a power play literally, too. The power consumption of a single GPU has been compared to that of a domestic dwelling. Scale that up to Zuckerbergian levels and the power requirements are eyewatering. As Sharp says, “careful planning around power supplies is key.”
How the world has changed, muses Ben Pritchard. The chief executive of specialist power systems firm AVK-SEG recalls that, as late as last year, his industry peers were chattering about a contraction in the data centre sector. In fact, explains Pritchard, the hyperscalers had effectively paused to redraw their facility blueprints as they pondered how to scale capacity to meet surging demand for AI services.
What will those plans produce? The backup generators needed to keep a site ticking over in the event of a power failure give some clue. “A commercial building in London might have four generators,” says Pritchard. “A data centre typically has anywhere between 20 to 50.”
That power requirement is one reason why Spencer Lamb, chief commercial officer of UK-based data centre operator Kao Data, believes the vast majority of customers won’t be running their own data centres, at least when it comes to AI workloads. “CEOs are mandating that they need to get their AI strategy in place quickly, and therefore they need to have access to those AI resources quickly,” says Lamb. The idea of going out and “buying a data centre, or buying data centre capacity from someone like us, buying the NVIDIA GPUs, increasing the staff that can actually commission all that equipment and then operate it is probably not an option for most, if not all.”
At least not today. The problem is that the pace of change has dramatically changed how companies can plan their data centre strategies into the future. Dan Scarbrough has tracked the cloud and data centre industry for over 20 years. Now chief commercial officer of data mobility specialist Stelia, he argues that AI has changed the game for industry expectations around investment in data centres.
That, says Scarborough, affects “the way this asset is being consumed, who the customers are, the lease cycle of chips, commoditization of the pricing structure and the effect that has on the funded asset, and how you amortise that asset over a period of time.”
Beware of the white elephant
Historically data centre operators assumed a data centre had a lifecycle of 10 to 15 years. But that is an aeon when you consider the rate at which NVIDIA is scaling up its offering and making previous infrastructure look laggardly.
Scarbrough draws a comparison with Moore’s Law, where Intel’s ability to cram transistors onto a wafer shaped the industry for decades. “It’s now at the point where the thermal performance of the chipset is fundamentally altering the underlying infrastructure,” he says.
Geography plays a part too. GPUs might be in one place, but some or all the data they need to crunch might reside elsewhere, as might their end users. As such, connectivity becomes critical. Part of the reason somewhere like West London has a high concentration of data centres is that as well as space and power, it offers the opportunity to tap into the mainline of transatlantic connectivity. A greenfield site in the middle of England might not be so blessed, even if it has sufficient power. And latency will be a real concern for applications that have real-time demands – whether they’re financially focused, or pertinent to factory automation and optimization.
Consequently, the rush to build AI-capable infrastructure raises the risk of white elephants. “There’ll be a lot of data centres built in locations where they just won’t get customers,” says Penny Madsen, a senior research director for cloud and edge services at IDC.
It’s not just land or power that might be in short supply. Companies thinking about their AI data centre strategy may realise they’ve progressively shed the people they need to implement them, whether that’s networking specialists or just people with the right operational skills.
“I think you’re going to see a lot of movement over the course of the next year, as things stabilize and people go actually, we do need a trusted partner to be able to help with it,” Madsen says. And in the first instance, she adds, this will likely be their primary cloud provider.
So, most CIOs will simply not be in a position to build or run their own “AI data centre”. The weight of infrastructure needed and the complexity of marshalling and deploying it will simply be too much.
Of course, that frees them from the burden of managing and paying for that infrastructure. They just need to ensure that their AI strategy is lightweight and agile enough that they can move it between providers as they see fit.
But that is all in the future, because for now, whatever Starmer and Trump’s ambitions, we’re only just seeing the fruits of the first attempts to build data centres capable of hosting Silicon Valley’s AI fever dreams.
“We’re told that we’ve seen a massive change in data centres,” says AVK-SEG’s Pritchard. “The reality is, at the moment, it hasn’t truly hit.”