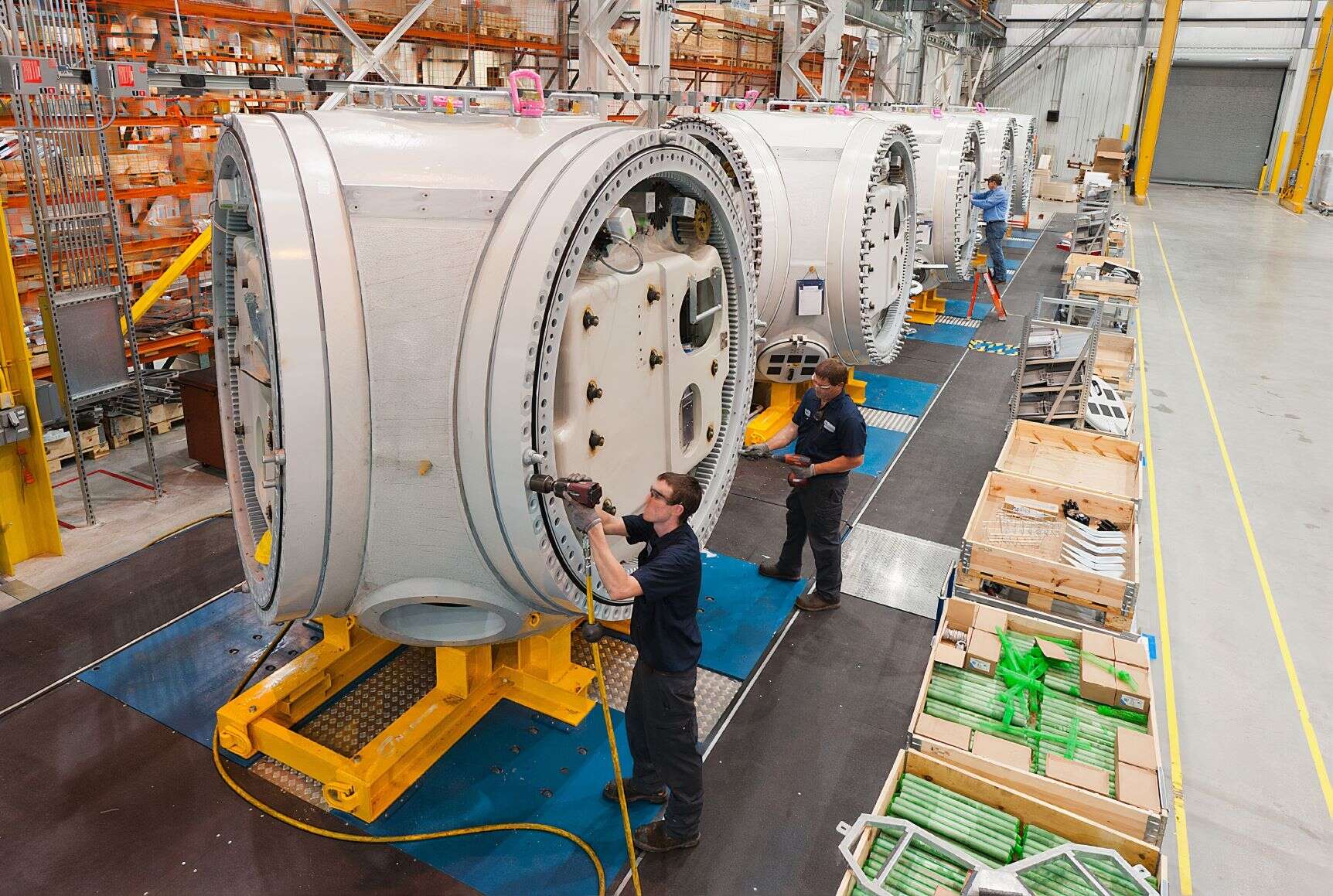
A new partnership between Siemens and Google Cloud aims to deliver more artificial intelligence tools to factories, with Siemens VP Axel Lorenz declaring that “the potential for AI to radically transform the plant floor is far from being exhausted” when the deal was announced on Monday. Adoption of AI across manufacturing has so far been patchy, and the sector will need to significantly upskill employees and update ageing legacy systems if it is to fully embrace the benefits of machine learning at scale.

The new partnership will see Siemens utilise Google Cloud’s AI and ML capabilities to implement innovative and competitive factory automation solutions for its customers. Lorenz, who is VP of ‘control at factory’ automation for Siemens, described the partnership as a “game changer” for manufacturing, adding: “Many manufacturers are still stuck in AI ‘pilot projects’ today – we want to change that.” Indeed, it is no surprise that Google and Siemens have identified an opportunity in the sector when many factories have yet to deploy advanced AI solutions.
Adoption of AI across manufacturing
So far the pace of AI adoption in manufacturing has been slow, but it is increasing. In 2018 annual spending on AI software, hardware and services was just $2.9bn according to data from the Worldwide Manufacturing Forum. This is expected to grow to $13.2bn by 2025. What’s more, 15% of manufacturing companies approached by Mckinsey now use AI to optimise key areas of production such as yield, energy or throughput optimisation, up from 9% in 2018, while 12% use it for predictive maintenance.
Uses of AI in manufacturing
“The most common use for AI is quality detection” explains Elisa Roth, doctoral researcher in engineering at the University of Cambridge’s Institute of Manufacturing. “It’s easy to implement vision-based AI into production lines that analyse products on the go.” A survey by PwC revealed 24% of companies polled are adopting assembly quality testing. General Motors uses computer vision to analyse images from robot mounted cameras to spot early signs of failing robotic parts. “Computer vision-based quality inspection is state-of-the-art because it is not something we need deep neural networks for,” Roth says.
Another common use is in predictive maintenance. Sensors and AI are used to check “when machines will need repair or when certain materials have to be changed”, says Roth. These sensors are getting more advanced, yielding more nuanced data to streamline the production process. “A step up from having a sensor to make sure your tank doesn’t overflow is to incorporate humidity or other environmental factors” explains Ellen Eichhorn senior director of consumer goods at Gartner. For example, Japanese tyre manufacturer Bridgestone has implemented a tyre assembly system called EXAMINATION, which uses sensors to measure the characteristics of individual tyres based on 480 data points. This has led to better quality tyres and a 15% increase in uniformity, according to a report on AI in manufacturing operations by Capgemini.
Historical data can also be optimised by AI to predict changes in consumer demand, so manufacturers can alter production schedules and raw material procurement. French food product manufacturer Danone has been using machine learning to improve its demand forecast accuracy, as well as planning between marketing, sales, account management, supply chain and finance. The system has enabled a 20% reduction in forecast error, a 30% fall in lost sales, a 30% cut in product obsolescence and a 50% easing in demand planners’ workload.
How to herald a new age of AI
But while some progress is being made on AI adoption, challenges remain across manufacturing. “Legacy systems are restraining the use of AI, particularly for smaller companies and in older factories,” says Roth. Forty per cent of companies polled by PwC cited the inability of existing ERP or manufacturing execution systems (MES) to interface with AI as a barrier to adoption. “Manufacturing execution systems control the whole manufacturing operation,” Roth explains. “They collect data from all the machines, letting you control all the machines and software processes.” These kinds of systems can “hold together whole organisations,” Roth says, meaning altering them can be a slow process.
But just as important as interoperability and the updating of legacy tech is the expertise of staff. “It’s not sufficient to understand machine learning alone,” says Roth. “You also need to be a process expert and the issue is we have a lack of both [types of skill].” To close this skills gap, people will need to embrace change, rather than resist it, says Gartner’s Eichhorn, who believes that though new AI systems are often viewed with suspicion, they can help make life easier for staff. “People sometimes assume that automation means you’re not relevant anymore, which has nothing to do with it,” she says. “We have to get past that mindset”.