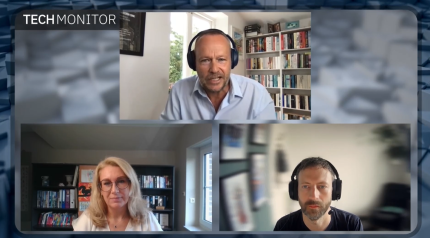
The transformative potential of generative AI has prompted talk of the dawn of a “Fourth Industrial Revolution”. However, while the levels of hype might be at fever pitch, the reality on the ground often looks somewhat different.
Enterprises across all industries recognise how GenAI initiatives might turbocharge productivity, creativity and growth, but where to prioritise and how to start generating value creation remains a challenge. Indeed, Microsoft’s 2024 Work Trend Index Annual Report found that a significant majority of business leaders worried that their organisations lacked a plan and vision to properly begin implementation.
The need to address these issues has prompted the creation of a Tech Monitor interview series, “Power of the Possible”, held in partnership with Snowflake. For the first instalment, associate editor Jon Bernstein sat down with Tatjana Wiebusch, Partner, AI & data at Deloitte, and Mike Taylor, field CTO, AI/ML, at Snowflake, for a discussion surrounding the cultural changes required to get going; bridging potential skills gaps; and the pitfalls to avoid.
Wiebusch spoke of the mindset shift required among one’s data leaders and beyond. “It’s such an important piece of the puzzle,” she began. “New AI tools allow for very decentralised access to data across all different business units, not just those in the central data organisation. That means the data experts ensuring all users have the right knowledge and skills to engage.”
The cost of getting GenAI initiatives wrong
But increased access comes with increased risks. Those with a strong existing data culture need not necessarily reinvent themselves anew, but they must be aware of how their exposure changes as data access becomes more democratised. “The foundational aspects of how you order and define remain the same, but the impact of getting it wrong has shifted,” Taylor cautioned. “You can find yourself getting left behind. This is something of a watershed moment. Mastery over this technology is becoming a major consideration in future viability for many organisations.”
No wonder the topic can seem overwhelming in scope and scale. With so much hype surrounding the extent of transformation GenAI is set to unleash, enterprises are often understandably daunted when it comes to deciding precisely where to begin. The answer, Wiebusch believes, is to think big, but start small.
“It’s about finding how you can cut it into slices so that you have use cases that both demonstrate value and allow the early adopters to work with these new tools,” she explained. “Yes, think about the big picture and how your organisation stands to benefit as a whole, but then look at what quick steps you can take to begin before scaling up. Being able to demonstrate early successes is really important.”
Taylor nodded in agreement: “There is no value in standing around on the sidelines. Start where it’s easiest, build something, then iterate.”
Looking within and without
However, “easy” all depends on context. The conversation went into some depth on a perceived lack of in-house skills and experience preventing projects from getting off the ground. Wiebusch spoke of the need for greater education and sharing of best practise – both to help better identify and leverage use cases, and to assuage any fear or hesitancy surrounding the GenAI discussion.
For Taylor, there was one skills issue above all others that enterprises must address. “You need a good handle on the regulatory and reputational components of all this,” he said. “If you don’t have total confidence that what you’re doing is legal, ethical and, frankly, isn’t going to embarrass you in some fashion, then you shouldn’t be doing it. Most other skills can be outsourced, but the buck stops with you when it comes to regulations and your reputation.”
On seeking the aforementioned external input and guidance, both contributors were unsurprisingly bullish. Indeed, Deloitte and Snowflake have an established partnership in place, a large component of which includes working together to help organisations maximize GenAI through better data strategy. “You can learn so much from working with those that have addressed these challenges before,” explained Wiebusch. “Bringing in that knowledge form outside will both help you get going more quickly and accelerate building up those skills internally.”
Taylor, who previously worked at both Accenture and Deloitte, was in agreement. “The third party model works best when you can tap into people who’ve already done what you want to start doing before.,” he explained. “Whether we’re talking consultants or software providers, it’s that sort of relevant experience you should be looking for in your partners.”
Time to get building
One consistent message running throughout this discussion was clear: Yes, this can be an intimidating, even overwhelming topic to tackle, but failure to embark upon this journey is quickly constituting a profound business risk. Indeed, in many industries that wastershed moment has already occurred. And, for those who fear they do not know where to begin, there is external expertise, guidance and technology readily available to help.
“Companies should not wait to get started,” Wiebusch cautioned. “Think about the right use cases and how you can build an ecosystem through combining internal and external partners that will enable you to get going as quickly and successfully as possible.”
“Business value will come eventually,” Taylor agreed. “But if you don’t get your hands dirty building something and getting it over the line now, it’s only going to become more and more difficult to do so in the future.
You can watch the conversation in full above. To learn more, download Unifying Enterprise Data for Generative AI, a paper from Snowflake and Deloitte on overcoming the data challenges GenAI adoption brings