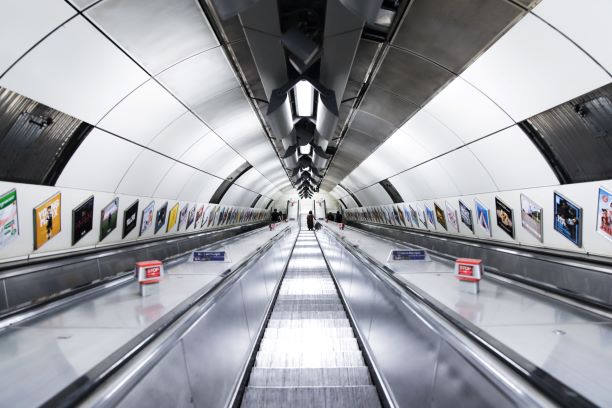
Forty people per year on average have died after being hit by trains on the London Underground over the past five years, Freedom of Information responses show.
Not all of these deaths were suicides: but many may have been avoidable and the company rightly wants to reduce the number to zero. Can technology help?
Launches “Technology Preventing Suicide” Project
Transport for London (TfL) hopes so. It has launched a new project, “Technology Preventing Suicide (TPS)” and is starting a feasibility study to “assess potential options for technological innovation to prevent suicide on the network.”
The company says it is “open to learning from the market” about the kinds of technology that could help it reduce deaths on its tracks to zero; in line with the London Mayor’s “Vision Zero” of zero road deaths by 2045.
“As part of the feasibility study, TfL are conducting early market engagement which should help shape how we define, contract for and procure and develop a totally new and innovative solution through assessing the current market capabilities as well as appetite from the market to partner with TfL to develop a new solution” it said.
Starting with a Questionnaire
The initial aim is to prove the “viability and feasibility of a technology, while also taking into consideration the possibility to scale up the deployment of the technology across the network to help reduce suicide attempts” TfL said in a public notice.
Various organisations have been using machine learning on public data sets in a bid to drive down suicide numbers.
Recent research led by the University of Swansea, for example, used algorithms running on neural networks to identify suicide risk.
Neural Networks To Identify Suicide Risk
Tapping Secure Anonymised Information Linkage Databank UK, a database of anonymised NHS data, the researchers extracted the data of those who died by suicide between 2001 and 2015 and paired controls.
They then built a binary feature vector coding the presence of risk factors at different times prior to death, ranging from GP contact and hospital admission; diagnosis of mental health issues; injury and poisoning; substance misuse; maltreatment; sleep disorders; and the prescription of opiates and psychotropics.
See also: Mental Health Data Hub Simplifies Datasets for Health Workers
“Basic artificial neural networks were trained to differentiate between the suicide cases and paired controls. We interpreted the output score as the estimated suicide risk. System performance was assessed with 10×10-fold repeated cross-validation, and its behavior was studied by representing the distribution of estimated risk across the cases and controls, and the distribution of factors across estimated risks.”
The team had notable success, extracting a total of 2604 suicide cases and 20 paired controls per case: “Our best system attained a mean error rate of 26.78 percent.”
Swansea’s Dr del Pozo Banos said: “We wanted to see if we could develop an algorithm that analyses routinely collected health data to flag people, so that when patients present with seemingly unrelated conditions, practitioners can ask them appropriate questions about their thoughts and feelings if required. “
He added: “Our proposal will not replace clinical assessment of immediate risk, but we hope it will help clinicians to identify vulnerable people when they access health services so that the right questions may be asked.”
ONS statistics show an average of 4,500 suicides for men and 1,500 for women yearly in recent years. The Samaritans can be reached free at any time on 116 123.