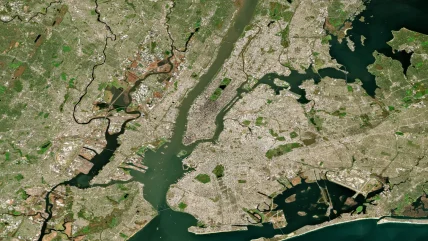
IBM and NASA have unveiled Prithvi-EO-2.0, an open-source advanced geospatial artificial intelligence (AI) foundation model developed in collaboration with Germany’s Jülich Supercomputing Centre.
The second-generation model builds on the capabilities of its predecessor, Prithvi-EO-1.0, by integrating enhanced architectural features and more sophisticated training methodologies to advance satellite data analysis and environmental monitoring.
Prithvi-EO-2.0 is based on the Vision Transformer (ViT) architecture and employs a masked autoencoder (MAE) approach for pretraining. It introduces two key advancements over its predecessor. The first is the incorporation of 3D patch embeddings and 3D positional encodings, enabling the model to handle spatiotemporal data. The embeddings divide input sequences of images into non-overlapping cubes that account for the dimensions of time, height, and width. Positional encodings are generated from sinusoidal functions for each dimension, which are then combined to enrich the model’s capacity to analyse dynamic patterns over time and space.
The second innovation involves the inclusion of geolocation data and temporal metadata during pretraining. Both the encoder and decoder components of the model independently process these attributes using weighted summations. A drop mechanism was introduced during training, randomly excluding geolocation or temporal data to help the model generalise and handle incomplete datasets effectively.
Trained on 4.2 million global time-series samples from NASA’s Harmonized Landsat and Sentinel (HLS) dataset, the 600-million parameter model represents a six-fold increase in scale compared to Prithvi-EO-1.0. IBM claims that Prithvi-EO-2.0 outperforms its predecessor by 8% on the GEO-Bench framework, a standard used to evaluate AI models on geospatial tasks such as image classification and segmentation. The model has also outperformed six other foundation models in various remote sensing applications, demonstrating its versatility across resolutions from 0.1 to 15 metres.
Applications extend to drones, agriculture, and urban planning
“Prithvi 2.0 can now better capture long-term processes season-by-season and also assimilate high-resolution information,” said Paolo Fraccaro, the IBM researcher who led the project. The model is being positioned for use in tasks such as disaster response, ecosystem monitoring, and land cover classification.
Beyond satellite data, the model extends its functionality to high-resolution imagery from drones, reportedly identifying features such as tree species, solar panels, and livestock. IBM highlights the model’s ability to handle both traditional Earth observation tasks and detailed, high-resolution analysis, enabling diverse applications across agriculture, urban planning, and environmental monitoring.
Prithvi-EO-2.0’s launch follows IBM’s announcement in September 2024 of a weather and climate AI model, also developed in partnership with NASA, with contributions from the US-based Oak Ridge National Laboratory. That model, available as open-source software on Hugging Face, was designed to power short-term weather forecasting and long-term climate projections. It complements the Prithvi family of AI models, which began with the release of Prithvi-EO-1.0 last year.
Prithvi-EO-2.0 is freely accessible via platforms such as Hugging Face, IBM’s TerraTorch toolkit, and GitHub, as part of efforts to make satellite data and geospatial tools more accessible to researchers and industry practitioners. By integrating advanced AI methodologies with metadata-driven training, IBM and NASA aim to address critical global challenges, including climate change, disaster preparedness, and sustainable resource management.
Recently, Google DeepMind unveiled a new AI model, GenCast, aimed at enhancing weather forecasting and predicting extreme conditions. In a paper published in Nature, the team detailed how GenCast improves the accuracy of weather forecasts, extending up to 15 days ahead. GenCast uses a diffusion technique, a form of generative AI typically employed in fields like image generation, which has been adapted for weather forecasting.