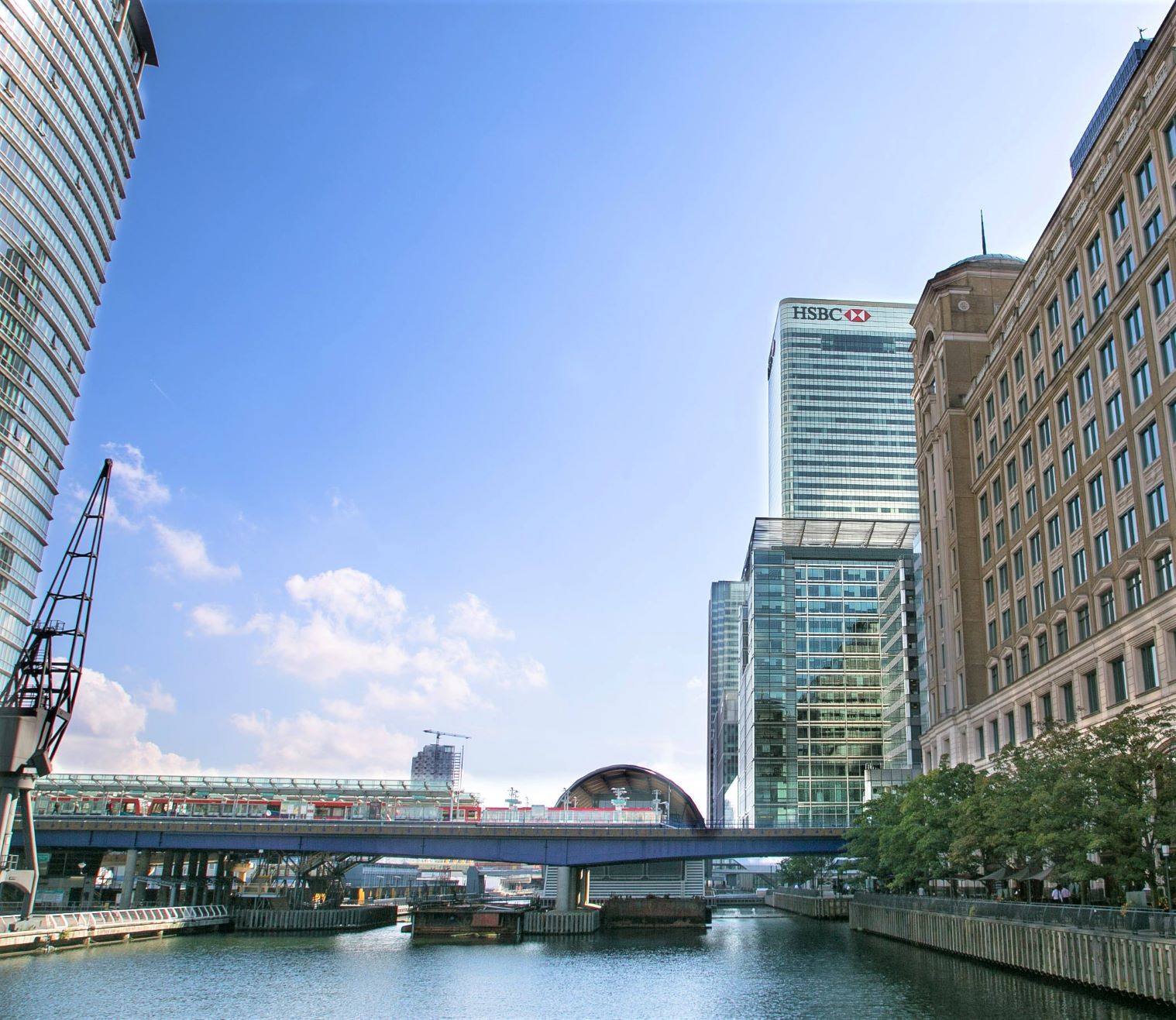
HSBC is building an AI-powered Client Intelligence Utility (CIU) built on 10 petabytes of corporate and institutional client data from 1.6 million clients.
The utility will be underpinned by the largest aggregation of corporate and institutional client data HSBC has ever put together: 22,000+ physical tables of information.
Collated over the past 12 months from across the 67 countries in which the bank operates, the project is being led by Chuck Teixeira, Chief Administrative Officer and Head of Transformation, HSBC Global Banking and Markets.
His team is now openly seeking help from AI specialists to develop the utility.
“We’re Being this Transparent Because we want to Create an AI Ecosystem of Partners”

It will deliver what HSBC is calling its “Client720” data asset, the bank’s first automated bird’s-eye view of client activity.
HSBC plans to use the CIU to tailor how products are structured, offer predictive insight into how macroeconomic and geopolitical events can impact a client’s global risk profile – allowing it to hedge exposure – and help automate compliance efforts.
It will support client products and services as diverse as loans, FX services or assistance in entering new markets.
(HSBC currently processes millions of transactions daily, sourced from 200 different data systems, 66 jurisdictions, 14 major product lines, and 75,000 data fields).
In a call with Computer Business Review, Teixeira – whose previous roles include one as MD and Global COO for capital and liquidity management at Barclay’s Investment Bank – said the Client Intelligence Utility was likely to run in the cloud.
See also: Five Key Takeaways from AWS’s Re:Invent Summit
He said: “I’ve been at HSBC for two years and when I first arrived it was a big surprise realising the extent to which our data is fragmented across different countries, different regions. We set out to put all the data in one place.”
“Trying to conform and aggregate it manually would have been nearly impossible, taken many years and cost hundreds of millions of dollars, so we partnered with a machine learning specialist, Tresata, to index it, join it up and cleanse it. It’s now in an on-prem Hadoop environment as real reusable data assets.”
He added: “We measure the data quality coming in on five different dimensions; accuracy, completeness, uniqueness, validity and consistency and then use ML to link transactions across disparate client identifiers. After pulling this together, we started using it for financial crime use cases. The next step is to leverage this to really shape how we service our clients.”
Client Intelligence Utility: Data Scientists, Engineers, Ethicists All in One Room…
A major challenge for financial services institutions trying to conduct projects like this is compliance concerns and legal issues, as well as struggles between data science teams, who need the data to train algorithms, and data engineers who are reluctant to share client data for compliance reasons.
Teixeira told Computer Business Review: “One of the first things we did is create a physically shared space for data science, data engineering and our IT teams to work together and also created a fourth team, a data sharing, privacy and ethics team who are physically co-located with them”.
Move to the Cloud Mooted…
He has hopes to migrate the dataset – all legal requirements being met – to the cloud.
Meanwhile, HSBC is looking for strategic AI companies to partner with.
It is working with CognitionX, an AI Advice Platform, to undertake a request for information process with leading AI firms to test their ability to deconstruct data and build truly scalable, machine learning software.
“Building this type of capability requires talent and expertise that is not only rare in the financial services industry, but rare across the globe,” said Texeira in an earlier HSBC release. “Selecting a number of partners – from corporate firms to individual and academic talent – will allow HSBC to build a collaborative ecosystem that challenges partners to solve use cases with HSBC, using the Client Intelligence Utility.”
He added in a call with Computer Business Review: “We’re being this transparent because we want to create an AI ecosystem of partners, even in academia. This ecosystem will allow these partners to come in and solve use cases directly with our front line employees, at speed, on a shared environment with reusable data assets.
See also: Databricks’ Open Source Platform Aims to Democratise “Machine Learning Zoo”