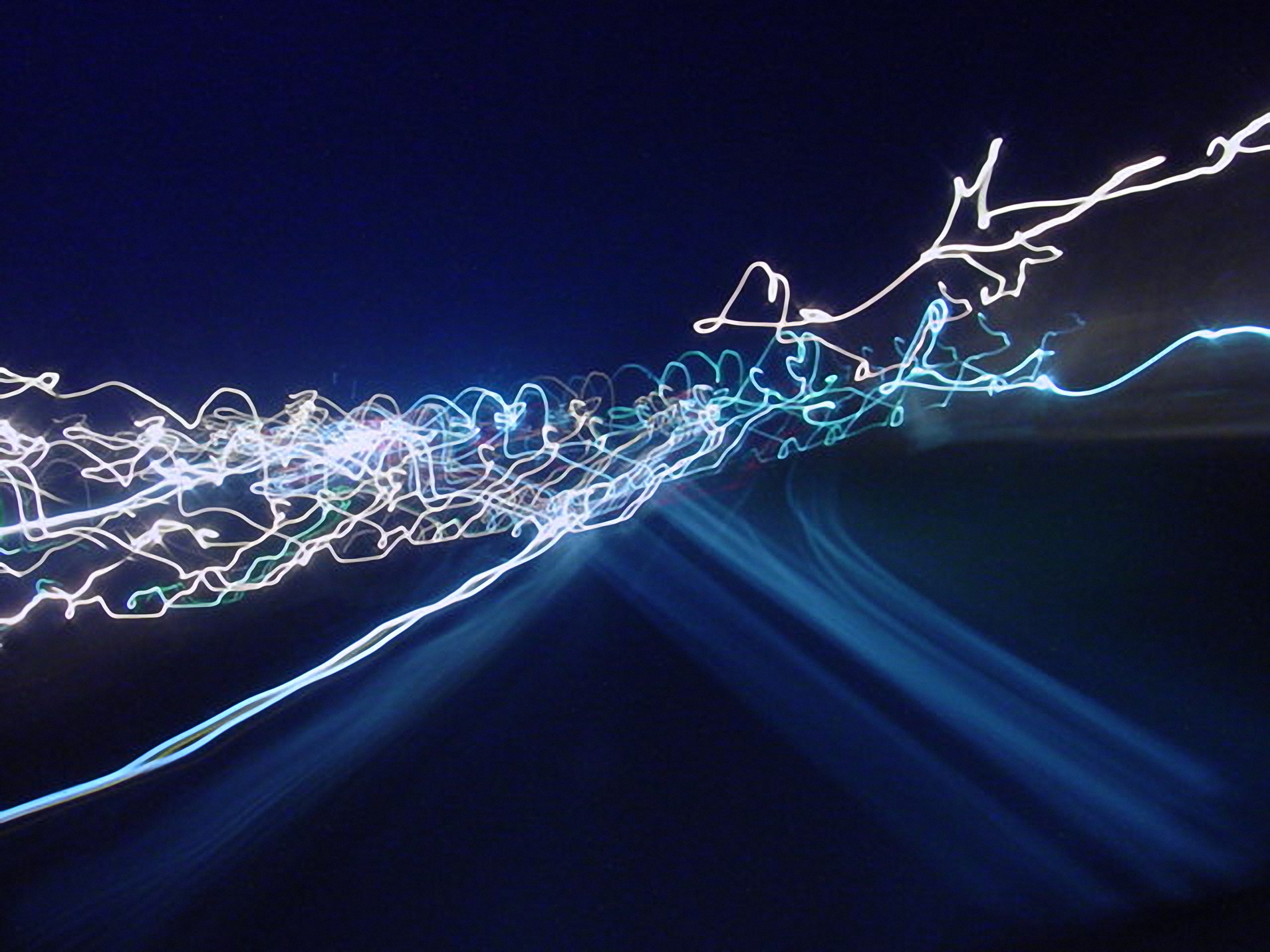
For better or for worse: How has the data science industry changed, and how has machine learning had an effect?
One of the biggest changes in the industry that I’ve noticed over the last few years is that more and more companies are embracing open source – for example, by sharing parts of their tool chain in GitHub. The availability of these tools is really great for making the most out of machine learning. I think data science and open source-related conferences are also growing, which means more people are not only getting interested in data science, but are also considering working together as open source contributors in their free time, which is a good thing.
Read more: Machine learning and data science workloads ignite Apache Spark adoption
Another shift in the industry that I’ve witnessed is the fact that deep learning is becoming more and more popular. However, this isn’t necessarily a positive change. There seems to be an urge to apply deep learning to problems even if it doesn’t necessarily make sense to. The willingness to embrace deep learning over the last few years is great, but sometimes it feels like lots of companies are succumbing to the urge to use deep learning just for the sake of it.
The positive thing to take away from this cultural shift is that people are getting excited about new and creative approaches to problem-solving, which can drive the field forward. One of the great things is that this excitement is driving communication and collaboration across different areas. For example, I’ve noticed that more and more people from other domains are increasingly familiar with the techniques used in statistical modeling and machine learning. Good communication in collaborations and teams is important, and a common knowledge about the basics makes this communication easier.
Looking forward: What’s the most exciting trend in data science and machine learning?
One trend I’m really interested in is the development of libraries that make machine learning even more accessible. Popular examples include TPOT and AutoML/auto-sklearn. These libraries further automate the building of machine learning pipelines. However, interpreting the outcomes of predictive modeling tasks and evaluating the results appropriately will always require a certain amount of knowledge. These tools don’t aim to replace experts in the field, but they may be able to make machine learning accessible to a broader audience of non-programmers. I see these tools not as replacements but rather as assistants for data scientists, to help automate tedious tasks such as hyperparameter tuning.
Another interesting trend I’ve observed is the continued development of novel deep learning architectures and the large progress being made in deep learning research overall. We’re seeing many interesting ideas from generative adversarial neural networks (GANs), densely connected neural networks (DenseNets), and ladder networks. Lots of progress has been made in this field thanks to new ideas and continued improvements of deep learning libraries (and our computing infrastructure), which is accelerating the implementation of research ideas and the development of these technologies in industrial applications.
Judgement Day: What’s the biggest misconception about machine learning?
Of course it’s the debate on the possibility of AI turning evil or going rogue. As far as I can tell, the fear mongering is mostly driven by writers who don’t work in the field looking for catchy headlines. I’m not going to iterate any of the arguments or evidence for this topic as I’m sure readers are capable of finding plenty of information (from both viewpoints) all over the internet, if they haven’t already. The only thing I’ll say on this topic is to quote Andrew Ng – “I don’t work on preventing AI from turning evil for the same reason that I don’t work on combating overpopulation on the planet Mars.” I think that says it all!