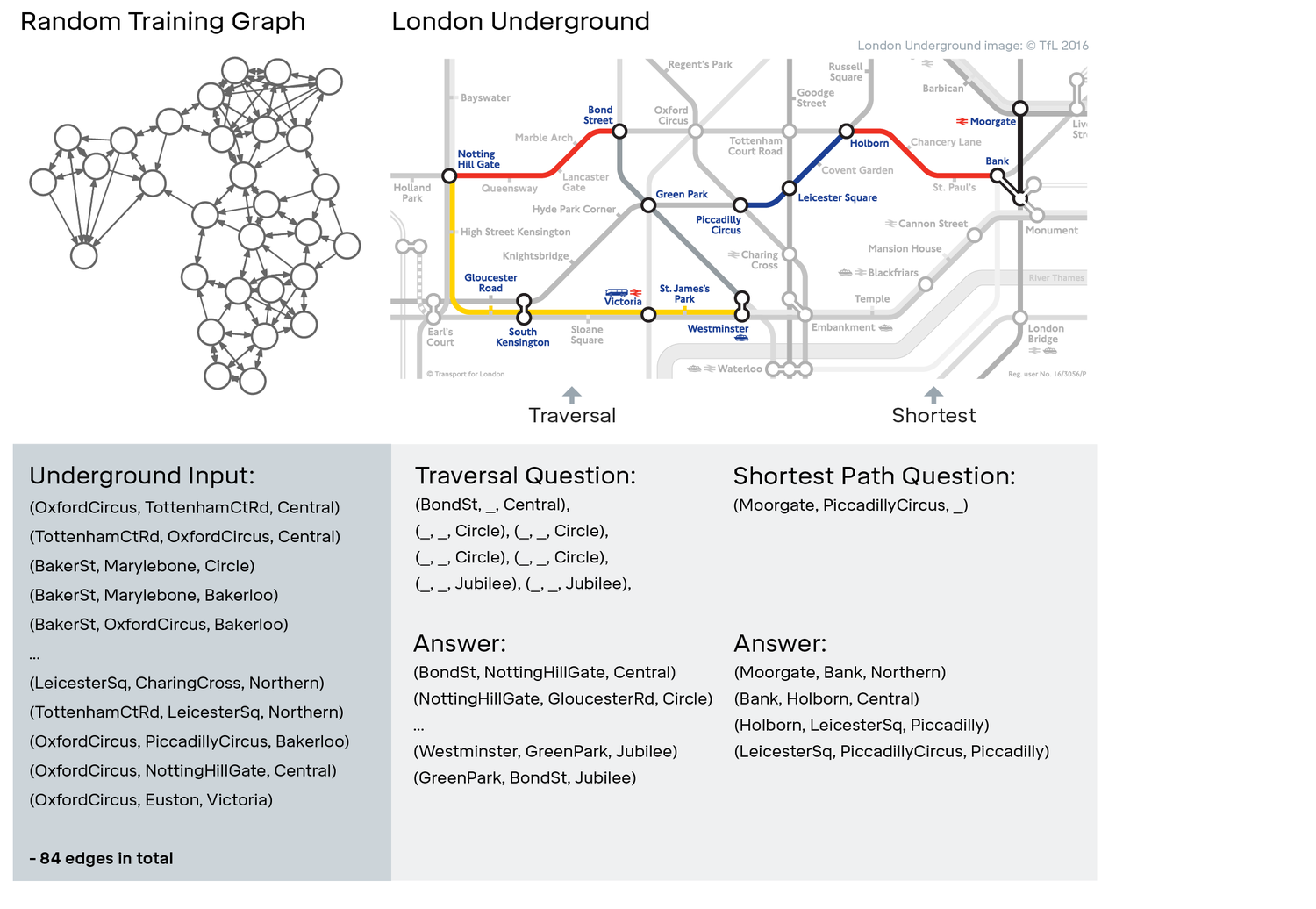
Scientists at Google’s DeepMind have developed a new programme, which leverages deep learning and external memory to navigate the London Underground system.
DeepMind said its new artificial intelligence (AI) model, dubbed a differentiable neural computer (DNC), can learn to use its memory to answer questions on complex, structured data, including artificially generated stories, family trees, and even a map of the London Underground.
It can also solve a block puzzle game using reinforcement learning.
Without prior programming, a DNC can organise data into connected facts and use those facts to solve problems.
While designing DNCs, the DeepMind team wanted machines that could learn to form and navigate complex data structures on their own.
A DNC is powered by a controller which is similar to a processor in a computer. It takes input, processes it, and writes it in memory and produces that can be interpreted as an answer.
A controller is capable of carrying out various operations on memory. Apart from writing, the controller can read from several locations in memory.
Memory can be searched depending on the content of each location, or the associative temporal links can be followed forward and backward to recall data written in sequence or in reverse.
The read out information can be used to produce answers to questions or actions to take in an environment.
The development has been published in the journal Nature.
The authors, which include Deepmind’s CEO and co-founder Demis Hassabis, wrote in the paper, “Taken together, our results demonstrate that DNCs have the capacity to solve complex, structured tasks that are inaccessible to neural networks without external read–write memory.”